Data Usage to Identify Current Trends in Biology via bioRxiv
The skills the author demoed here can be learned through taking Data Science with Machine Learning bootcamp with NYC Data Science Academy.
Introduction
Our world is in the midst (or at least the beginning) of a technological and scientific renaissance. This is particularly true for the biological sciences where multiple concurrent revolutions are unfolding. Among other examples, the genomics and CRISPR revolutions each marked watershed event in human history. It will likely take decades before their implications can be fully understood. That makes keeping up with current advances in either field a considerable challenge.
Additionally, itโs important to consider the fact that both fields are advancing rapidly, and that each is influencing the another. In parallel, advances in computer hardware, mathematics, materials science, and physics, are also spilling over into the biological sciences, further increasing the pace of discovery. As such, the challenge of staying abreast of current research and data can be daunting.
So, to develop a better understanding of recent trends in biological research, I developed a web scraping app (bioRxiv_scraper.py)capable of distilling some of the information in the scientific literature. Specifically, the app collects the number of abstract and full text views, subject, author information, and paper titles for a broad group of articles across biological disciplines. In an effort to get the most up-to-date information, I chose to scrape data from bioRxiv, a pre-print server, rather than PubMed.
While PubMed may be among the the most informative sources about long-term trends inresearch, the long delay between mansucript submission and publication implies that the field is six months to a year ahead. By analyzing pre-prints, I hope to reduce this temporal limitation.
Data Analysis - Pt. 1
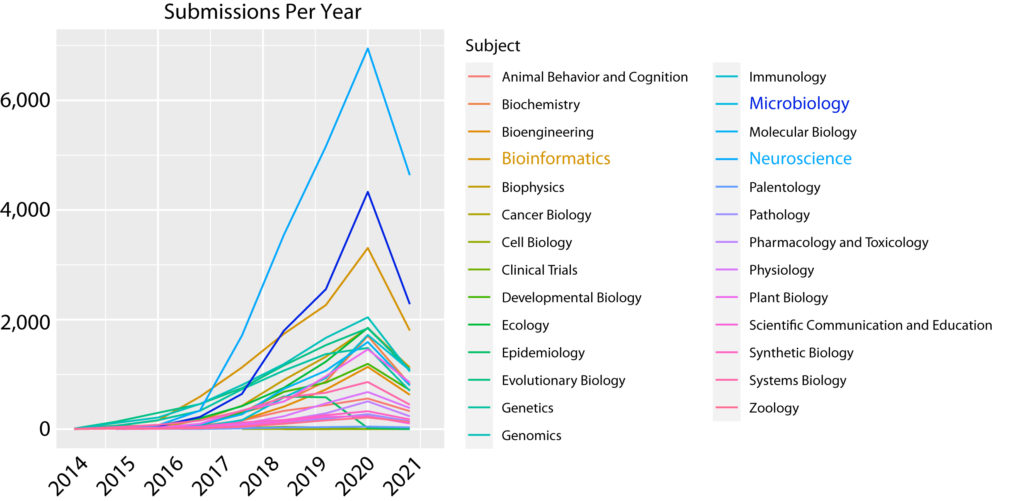
Figure 1. Manuscript submissions to bioRxiv by subject and year.
Scraping the full database took about four days, and yielded information from 128,175 manuscripts. The trends in the data are fascinating from a number of perspectives. First, it's clear that this is an excellent way of identifying papers that are attracting a great deal of attention. The data also reveals how the power and influence of preprint servers is increasing over time. Over the last five years, the number of submissions to bioRxiv has increased by an order of magnitude, a trend that is likely to only keep increasing into the future.
Notably, as this data set was collected half-way through 2021, the numbers for the current year only reflect the half-way point and therefore appear to drop. Yet as mean values, they still represent a 34% year-over year increase (in the example of neurobiology) relative to the mid-point of 2020.
Likewise, the data also demonstrates that research in neurobiology represents the most common submission, dwarfing the most other disciplines by more than 3:1. In close second/third are microbiology and bioinformatics respectively. Interestingly, neurobiology and microbiology came to dominate the submission landscape from 2017-2019, after initially ranking below other disciplines. This could be due to a variety of factors and does not necessarily reflect a change in overall productivity of any particular discipline.
For example, in the case of bioinformatics related submissions, it would certainly make sense that computer-savy scientists might adopt the use of pre-print servers ahead of other less-tech intensive disciplines. Irrespective of the reason, it seems likely that neurobiology and microbiology may be moving forward at a faster rate than other fields.
Data Analysis - Pt. 2
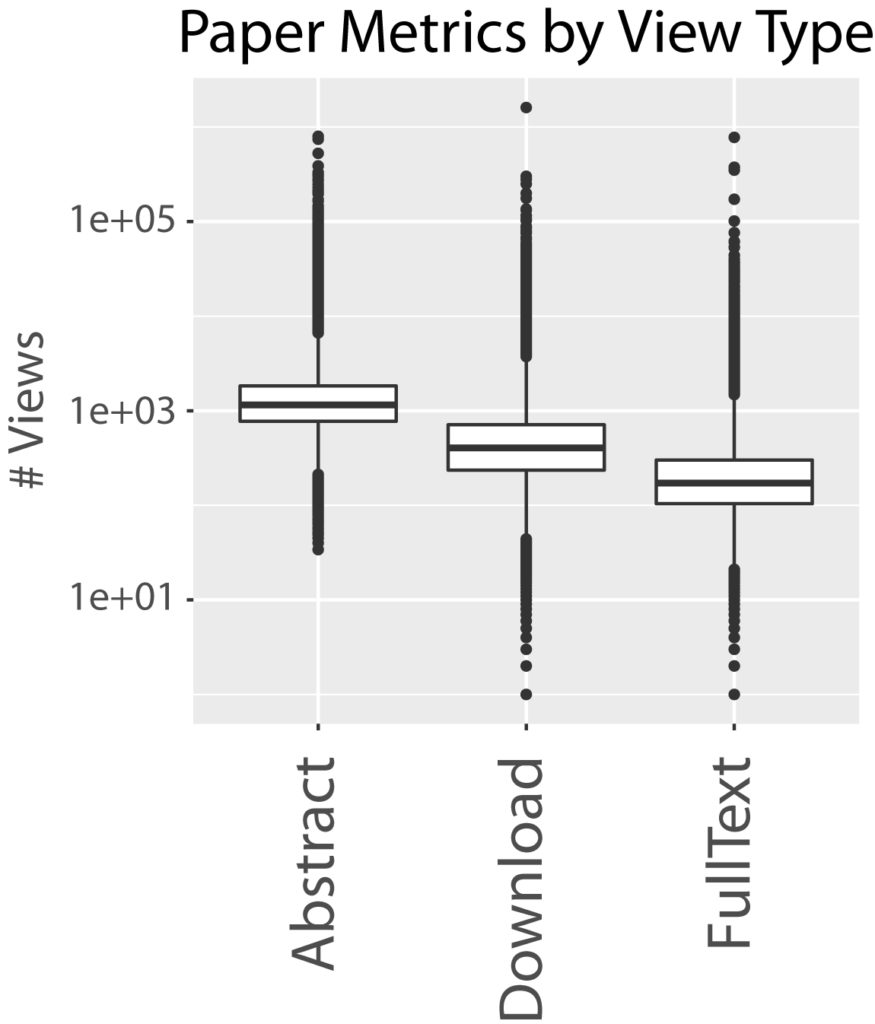
Figure 2. Viewing Metrics (note the log scale!)
Breaking this down by category (and temporarily ignoring the data distributions for the sake of clarity), we can see that interest in the details of individual papers varies significantly by field.
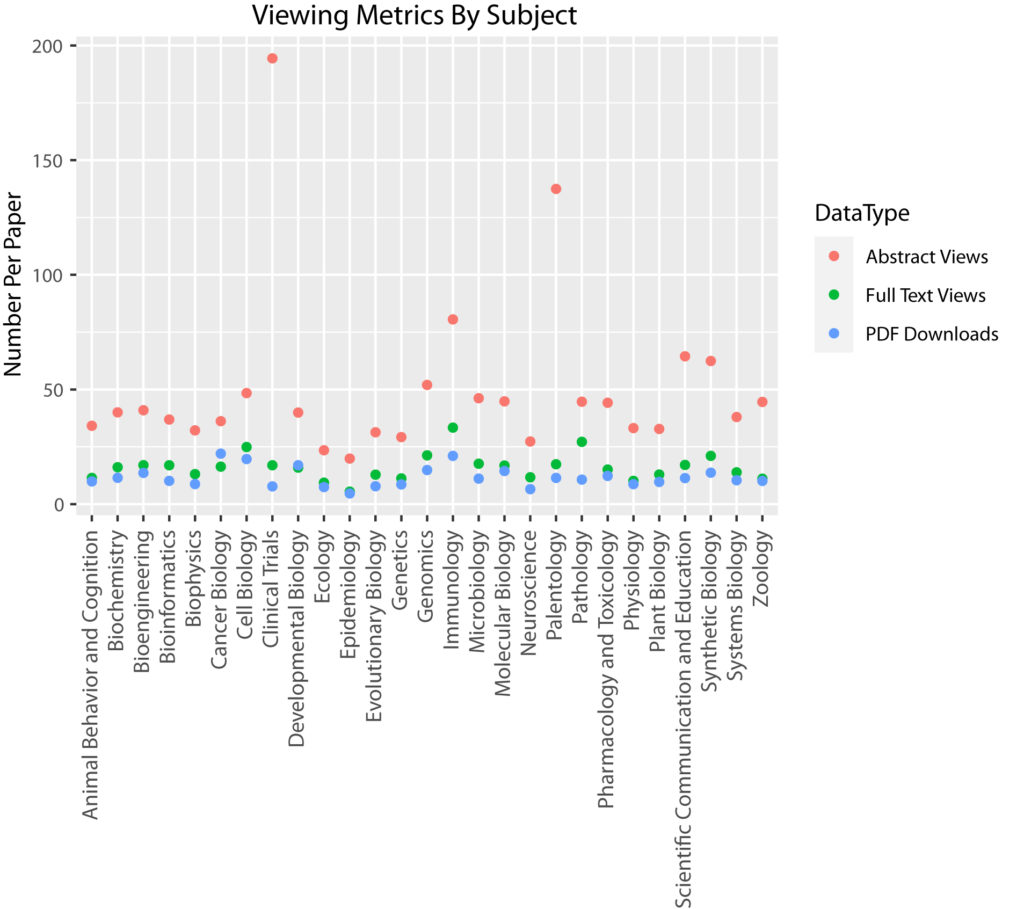
Figure 3. Average viewing metrics by subject and view type. Data points represent averages and are deliberately displayed without error bars for the sake of clarity.
For example, papers in the clinical trial and paleontology categories receive an unusually high number of abstract reads per manuscript. This trend is similar to the median value (Figure 4) suggesting it's not due to the effect of outliers:
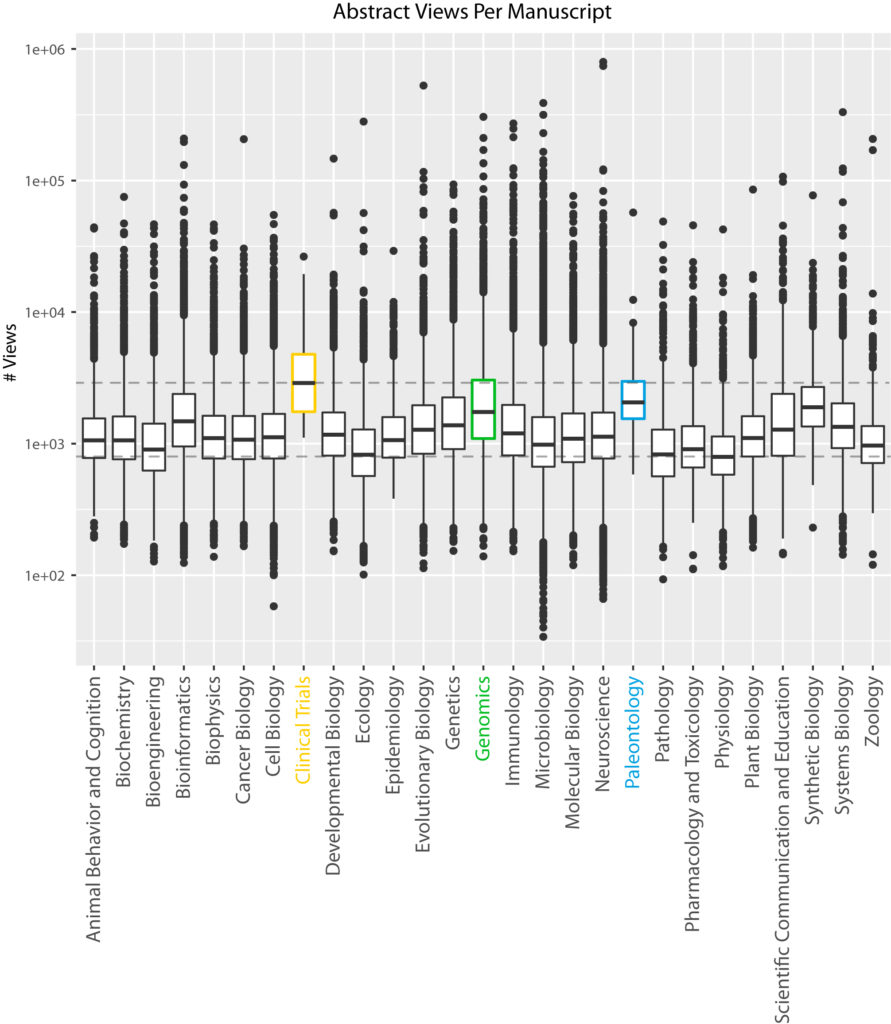
Figure 4. Distribution of abstract views per paper by subject and view type. Top three subjects are highlighted (colors). The top and bottom subject median values are indicated with the dashed line for comparison.
Looking a bit deeper, we can get a sense of whether each paper is read in detail or simply skimmed by dividing the number of full text reads by the number of abstract reads (Figure 5). This shows that the clinical trial and paleontology papers tend to be skimmed rather than read in full. This certainly makes sense as clinicians are likely to care far more about the outcome of a given study than about its design which should be fairly standard. Likewise it may be unusual for paleontologists to develop new or detailed methods, allowing the abstract to encapsulate the majority of a paperโs message.
Notably, manuscripts in the cell biology and cancer biology categories tend to have a higher number of full views per manuscript, in keeping with the detailed nature of such studies. In a somewhat surprising finding, microbiology and neuroscience have a lower frequency of full views despite being every bit as detail oriented as cancer biology. One possible explanation is that these categories may represent a collection of more specialized sub-topics, making their finer details less relevant to the broader community than their general conclusions.
Perhaps unsurprisingly, the number of abstracts is directly proportional to the number of Tweets (Figure 6). However, certain subjects tend to receive more attention on Twitter than others which is an interesting metric. It could very well suggest that Tweets about ecology papers have a greater impact on readership than Tweets about animal physiology.
That would make it particularly important for some disciplines (e.g. ecology and systems biology) to harness Twitter. Of course, it's also possible that higher readership simply drives more Tweets making this a less interesting relationship. Further work would have to be done to identify causality.
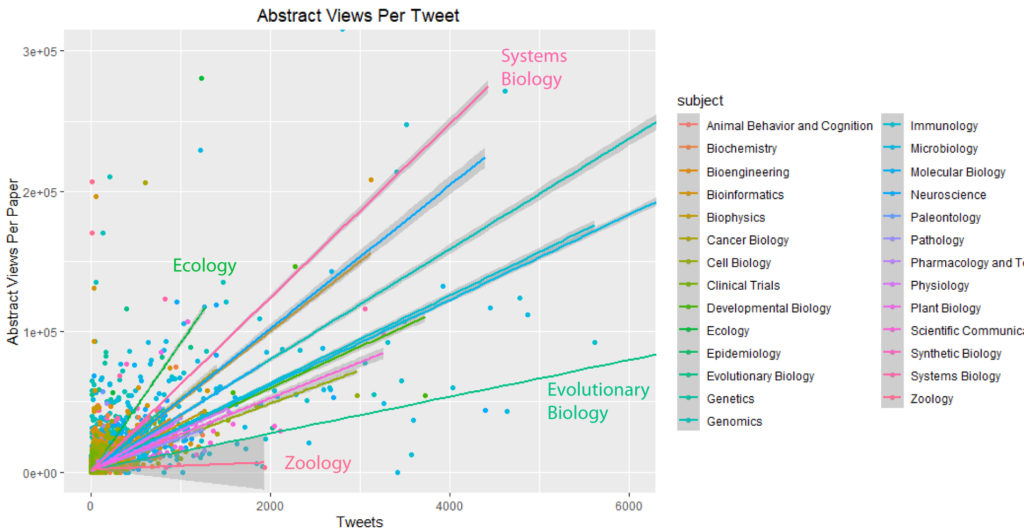
Last, and perhaps most interestingly, I identified the most viewed paper in each category, both overall, and for the months of June and July 2021. On its own, the monthly update may be the most interesting and useful application of the app.
In June, the winner far and away was a manuscript on the Sars-Cov-2 virus by Jesse Bloom, a researcher at the Fred Hutchinson Cancer Institute who specializes in viral evolution. This manuscript received over 36,000 views in a single month! Similarly, July's top manuscript (topping 85,000 views) is a study of Sars-Cov-2 neutralizing antibodies by the Laudau lab at NYU. Overall, the top papers table is probably worth a glance, if only to keep up with some of the most interesting new data.
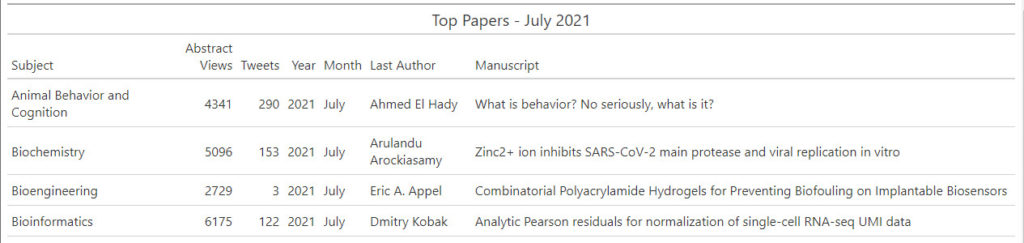
Likewise the top papers of all time is also quite informative. Below is a short excerpt showing the top two manuscripts across all disciplines. The first, perhaps not surprisingly is again on the SARS-CoV-2 virus. But the second is a study of the tau protein, one of the causes of Alzheimer's disease.

Final thoughts: Overall, this exercise has been informative about a number of trends across biological disciplines. The broad adoption preprint servers is only increasing over time and in the case of bioRxiv, the most common submission by far, is in the field of neuroscience. By compiling the number of paper views and Tweets, the app was able to identify some of the most significant papers in each field in an ongoing basis, providing scientists with a tool for keeping track of a variety of fields.
Technical methodologies and statistical analyses:
The bioRxiv scraper can be downloaded from github.
After scraping the bioRxiv data using Selenium with Python, I analyzed the data in R Studio. Perhaps unsurprisingly, the identify_outliers function and QQ plots show that the viewing metrics are not normally distributed, due to outlier manuscripts with extremely high numbers of views.
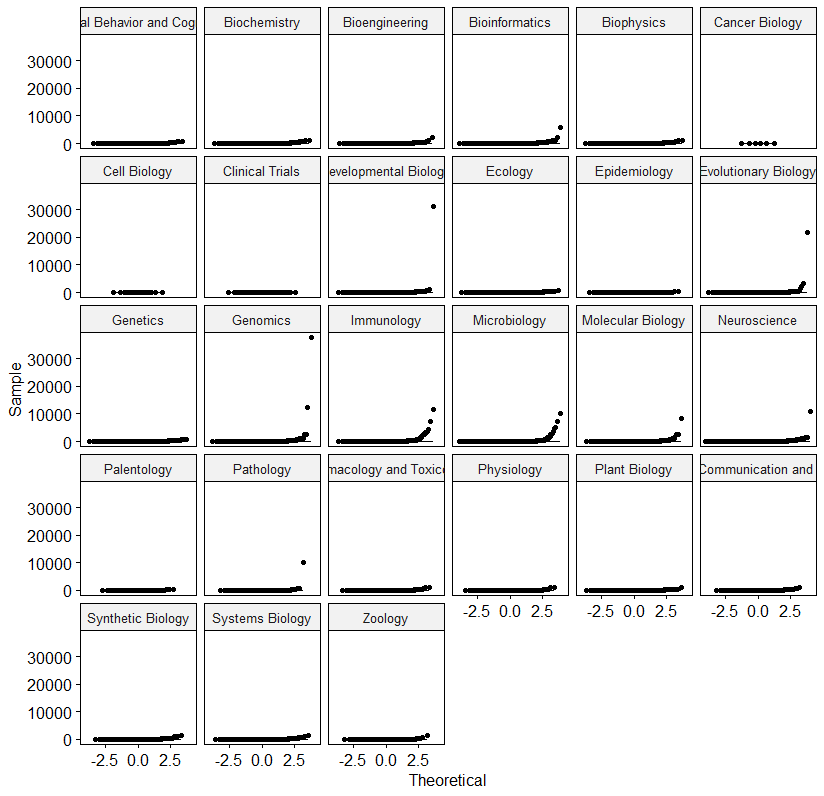
The Levene test for all the groups shows that variance is non-uniform (p = 10-26!) meaning that non-parametric tests are the appropriate option. I used the Kruskal-Wallace test to analyze the number of abstract views per subject which returned a p-value of 10-16, indicating significant differences between subjects.
To identify individual subjects with significant differences I used the Dunn test which performs pair-wise comparisons between groups. While it's certainly clear that some groups are different than others, it was more informative to compare all the groups to the same mean. For this, I picked the Ecology group which has a mean and standard deviation that's fairly representative of the group as a whole.
Compared to the ecology category, a number of subjects have a statistically significant difference (positively or negatively), e.g. bioengineering and biochemistry have more full reads than normal whereas clinical trial papers have fewer full reads than normal. The full list is:
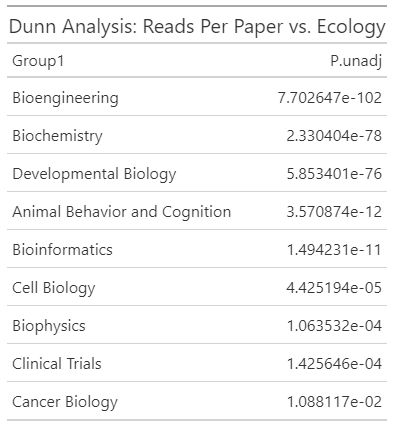