Analyzing Housing Data with Machine Learning
The skills I demoed here can be learned through taking Data Science with Machine Learning bootcamp with NYC Data Science Academy.
Photo by Jeffrey Czum from Pexels
Background
This project is based around a competition hosted here on Kaggle, with the object of prediction sales prices given historical data. The Data is originally from an Ames Housing Market dataset built by Dean De Cock for, coincidentally, educational purposes. Ranging from 2006-2010, the data provides an in-depth look into the numerous features/amenities associated with properties in the Ames, Iowa area and their purported sale price. The data set also comes with test data, used for trying out your program and evaluating its accuracy. You can find the code for this project on my GitHub.
What is Machine Learning?!
Not nearly as scary as it sounds, ML is basically just a fancy name for program that is โtaughtโ using sample data, to piece together patterns and make โassumptionsโ on those patterns with a certain degree of certainty, with minimal user intervention. Instead of managing the pattern recognition manually and doing all the calculations, we can make a program do the legwork for us! Most programs are very different from the idea of โartificial intelligenceโ, and are better described as efficient and advanced data analysis tools; In other words, a really smart calculator.
Exploratory Data Analysis (E.D.A)
For this dataset, which was quite elaborate, (50 columns worth of categorical and numerical data), I decided to run an str function, aka a structure function, as well as a summary and quantile to get a bearing on the distribution of of the data. Then, I utilized ggplot2 to quickly explore the data set, which we will see in a just a moment. I made sure to compare the count values against categorical descriptions to understand prevalence of relevant features. I also checked the skew of the data to see where analysis efforts were best allocated.
Data on Different Types of Housing
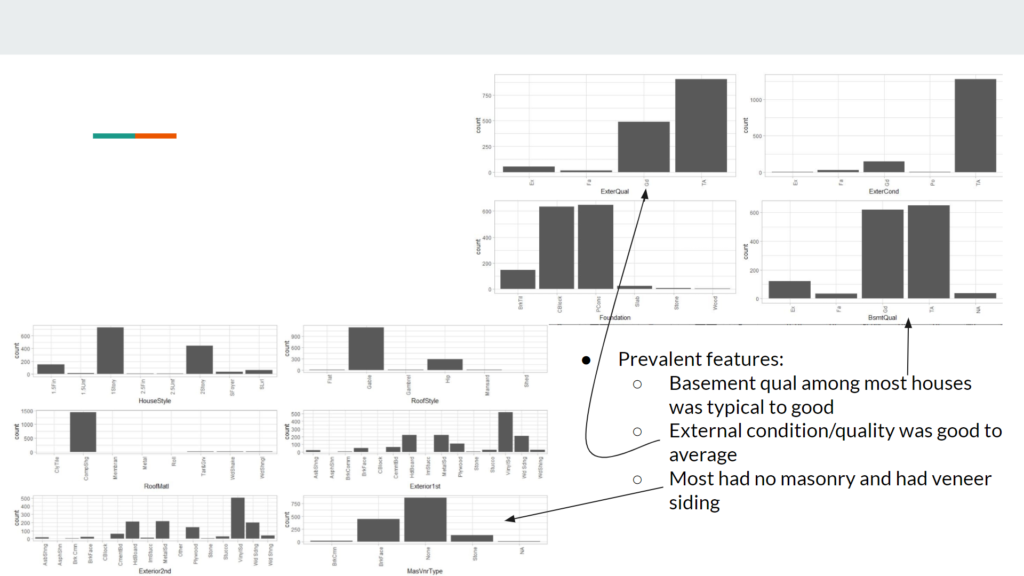
With over 50 columns/categories of data, there was a lot of EDA to do with this set. In this first feature, we can see the proportion of houses from the set with exterior qualities ranging from TA (typical to average) quality to EX (excellent quality), with most of the houses meeting the TA/Good quality. Basement quality was on average also within this quality range, with almost none of the houses having any masonry work done. These may sound like random factors but these are are qualities that people look at when deciding how much they want to pay for a new home, and also when looking to sell one.
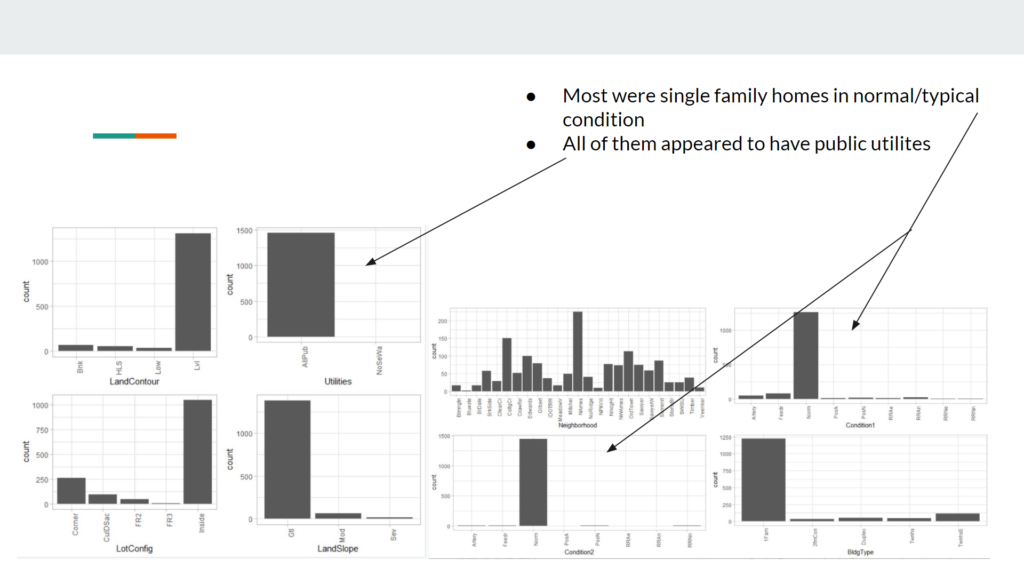
A lot of these houses were single family homes, of normal/TA condition, which is a good sign, and all of them were connected to public utilities (aka. sewer) instead of having to deal with septic. Septic can be an expense that becomes costly to deal with, so public sewer/water access is usually a big plus when evaluating property pricing.
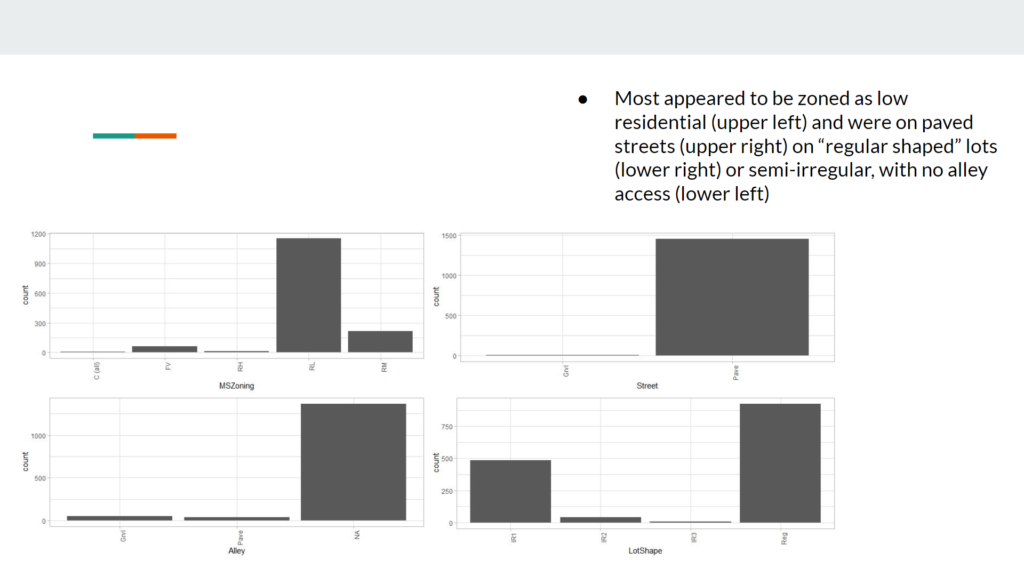
A majority of the houses were zoned as low residential (meaning lots of open space), had paved streets which is a big plus, on regular shaped lots with no alleys around them. All of these factors seem to paint a picture of homes with lots of space to grow families in apparently affordable areas.
Data on Different Neighborhoods
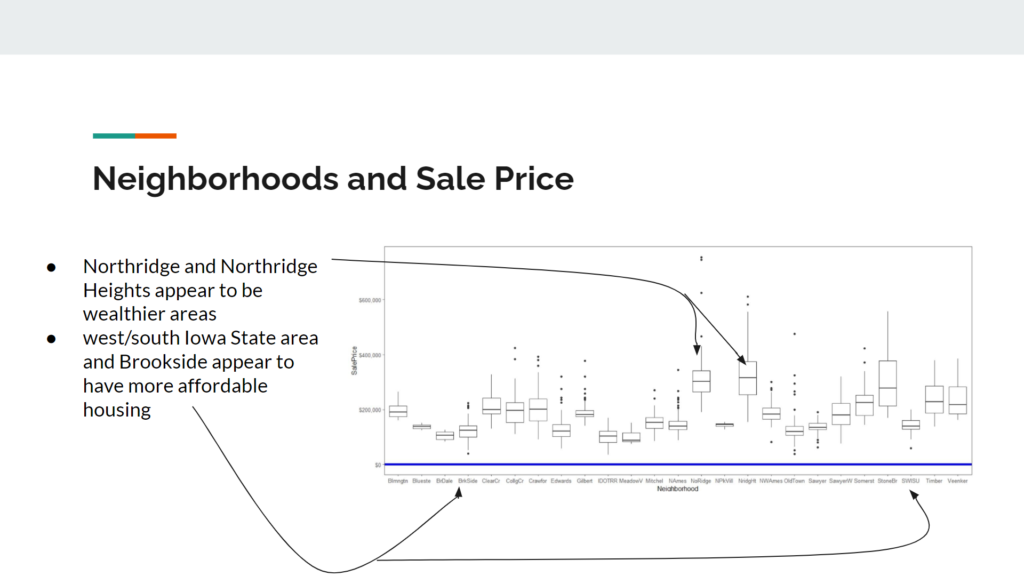
This figure shows us the spread of homes across all the neighborhoods in the Ames, Iowa area. The two areas that stuck out (marked by arrows) at the top were the Northridge and Northridge Heights areas, apparently containing a large proportion of homes with high resale value. The two regions also marked by arrows, that sit at the opposite end of the sale price spectrum, were around the Iowa State University area, and Brookside, containing houses that were on average more affordable.
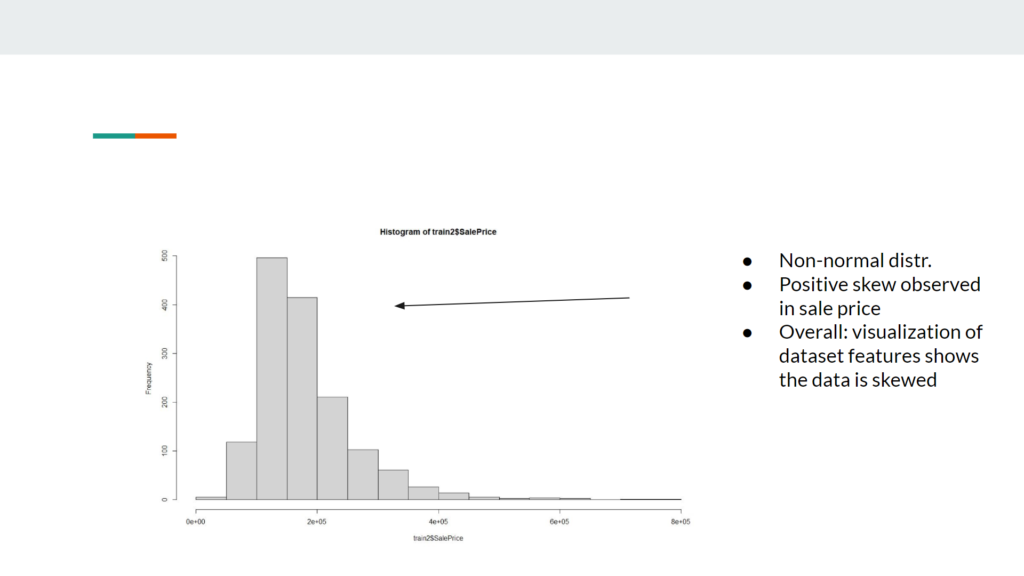
This histogram shows a very important feature of the data, which is the positive skew. We can clearly see that the sale prices of the homes in Ames sit at the lower end of the sale price spectrum in a much larger proportion, than those at the higher end. This visualization confirms that we can focus the efforts of the analysis on this end of the price range, to get the most accurate data.
Heat Map Data
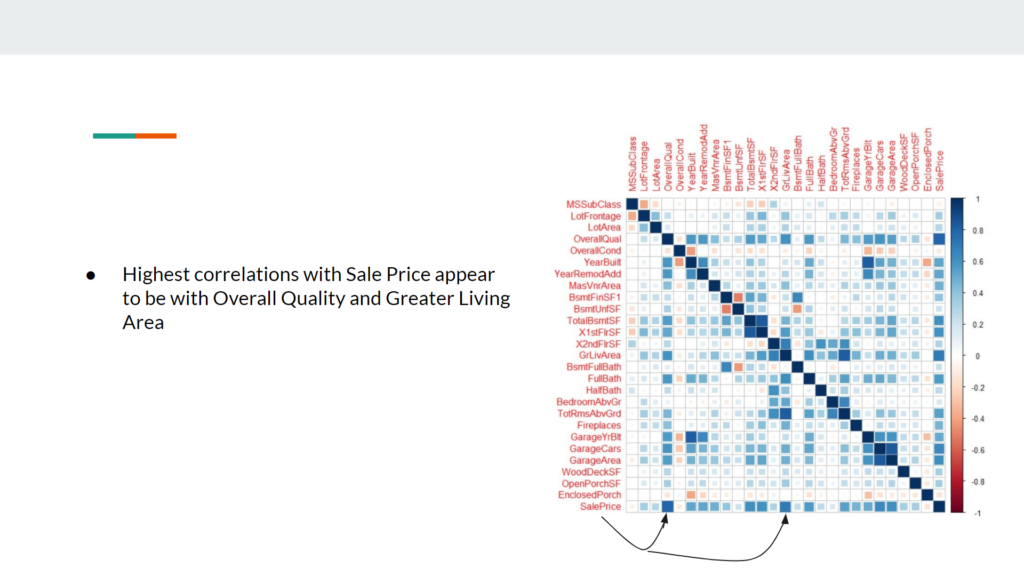
This heat map, or correlation plot, helps visualize which categories appear to mean the most when it comes to determining sales price. Dark blue means highly correlated, and dark red means the opposite. Marked by arrows, we can see sale price (bottom left) is highly correlated with two things in particular, Overall Quality and Greater Living Area. This makes sense, as a prospective home owner, you would want a home that's in good shape in a desirable area, that meets your needs.
Scatterplot on Quality of Housing
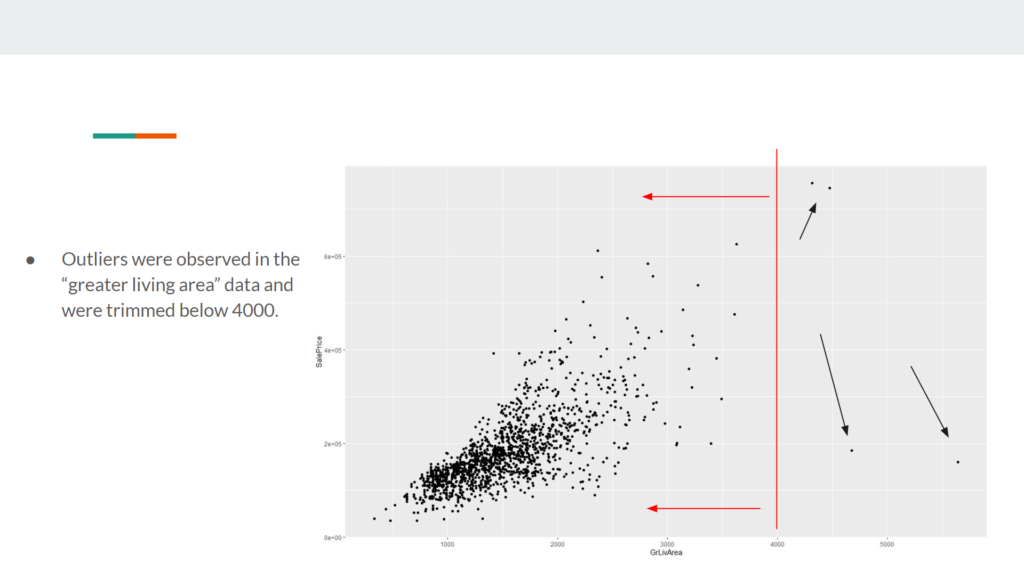
When taking a look at the Greater Living Area data in this scatterplot, it became evident that there were some outliers in the data. Normally, you want to keep as many data points as possible, however a majority of the data has a living area below the 4000 mark. In order to limit the effect of these outliers on the predictive accuracy, the data set was trimmed to those points below the 4000 mark. In addition to this trimming, all of the data was factorized so the program was run, and all the N/A data points were changed to "None"
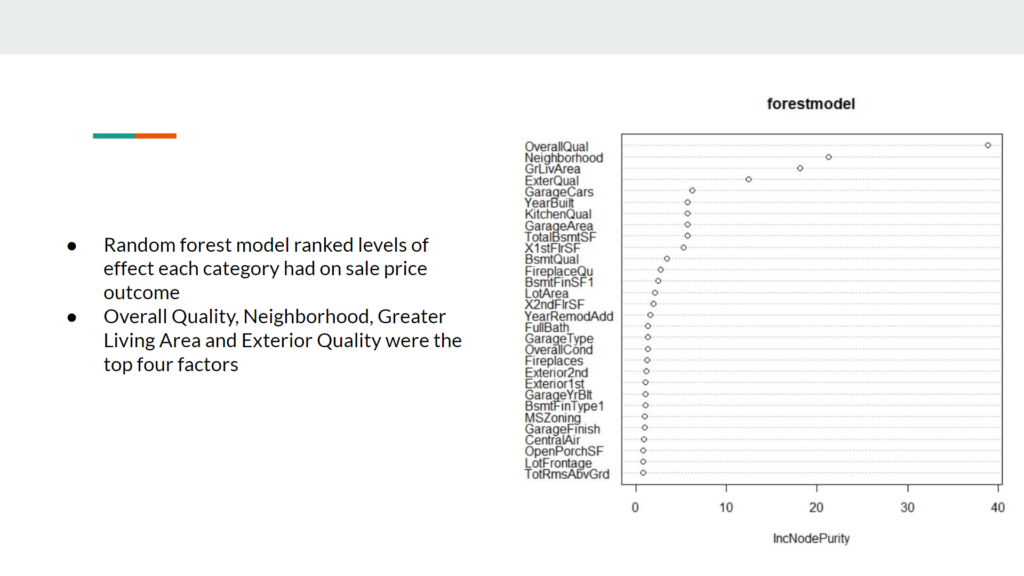
When running our model through Random Forest, the model resulted in this plot, which ranked the importance of certain features on the outcome of the predicted sale prices. As we can see, in correlation with the previous heat map, Overall Quality and Greater Living Area are major factors when it comes to determining sale price, along with Exterior Quality and the Neighborhood the home is located in.
Data Results
Running Random Forest resulted in a regression during which 500 trees were processed, and ~ 87.6% of the variance being explained by our model, with a Mean Square of Residuals of about 0.02, which isn't half bad. As a comparison, a quick Simple Linear Regression was run as well, using the top for importance categories from the previous figure as reference. This resulted in a model that explained ~ 83% of the variance with an evaluation RMSE of 0.83 as well.
Takeaway from Gathered Data
Comparatively, both Random Forest and Simple Linear Regression were not far off from one another, with regards to this data set. A simple SLR may be more efficient in this type of market evaluation. Random Forest results in a more In-depth analysis, and definitely takes more data into account. This analysis is very useful for models with lots of different types of data to take into account.
On the other hand, this analysis can be time consuming, especially when it comes to preparation of the data, and in general tends to be a much more complicated process. Simple Linear Regression on the other hand is quick and easy and is a good way to explore potential results, taken with grain of salt of course. In that case, you are working with โdummified dataโ, because SLR cannot work with nearly the same amount of categories that Random Forest can.
This analytical model doesnโt necessarily take every factor into account, so it's better for quick personal use or just quick evaluations, where you are trying to decide where to concentrate your efforts.
Down the Road
I would have really liked to try out XGboost and other models in python to see how the design fares, efficiency wise, vs building it in RStudio. I would also like to boost the accuracy of the models in this project. While high 80's is not bad, generally it is best to work with in the mid 90% range to ensure you're getting proper data from your model, especially in a professional setting.