App-tastic! - Navigating the App Jungle
This blog is cogitation of the bustling and enchanting digital wonderland, a treasure trove that melds the ingenuity of developers, the wonder of enthusiasts, and the insatiable delights of users.
It draws on the Google Play Store dataset from Kaggle to scour the essential metrics and variant patterns that bear out the dynamic Google Play Store, unknot the existing trends in the famous Android app market, and unearth substantial business insights regarding advertisements and investments.
Data Overview
There are 10,841 observations configured into 13 elaborate features, comprehending unique product names, categories, ratings, number of reviews, app sizes, installations, subscription options, product prices, content ratings, app genres, last update dates, current versions, and compatible Android versions. However, the core of my analysis centres upon some extracts of this assortment.
Data Preparation
I exempted the Genres, Type, Last Updated, Current Ver, and Android Ver features because of their irrelevancy to this study, then excluded a row consisting invalid inputs to guarantee a cleaner dataset. These procedures countenanced converting the Reviews, Size, Installations, and Price features to numeric type to secure a more helpful study with denotative visualizations. Lastly, I substituted the lost values in Ratings with their mean to rectify the missing data (1474) entries. This was the most eligible technique due to the arbitrariness of the lost data and its solidity against conceivable systematic errors.
Data Analysis and Visualization
The specific objectives and data types swayed my preferences for the displayed graphs to enhance a practical illustration of centrality measures and inexplicit patterns.
Rating
A user's quantitative estimation of a product is its rating. The rating scale offered on the Play Store spans one to five, one denoting the lowest score possible. This impressionistic feedback gives developers an assessment of their product(s) spanning performance to user satisfaction and also furnishes prospective users with perceptions of its quality, functionality, and satisfaction.
The figures below depict the statistical distribution of the ratings in a histogram and box plot.
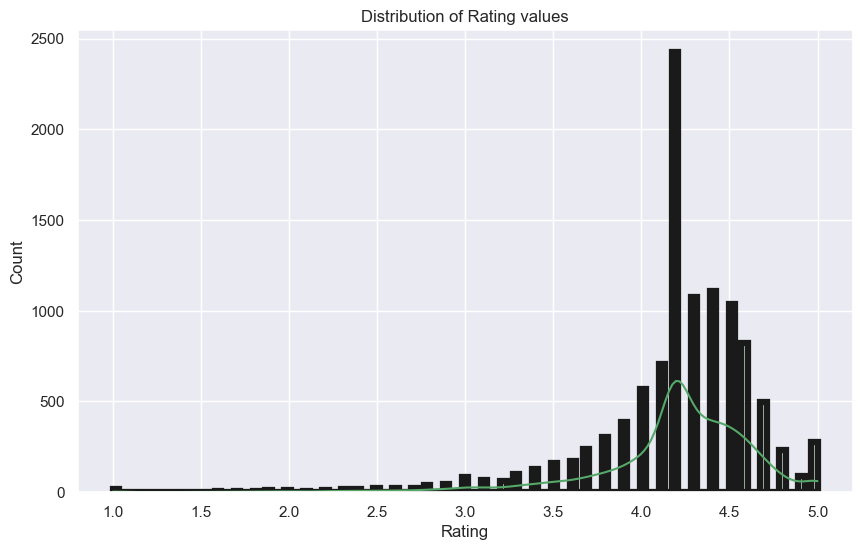
The peak around 4.2 in the histogram denotes the median value, while the modest elevation at the scale's perfect value signifies a few perfectly rated products. The panoptic distribution of the data points exemplifies an ideal democracy of user opinions.
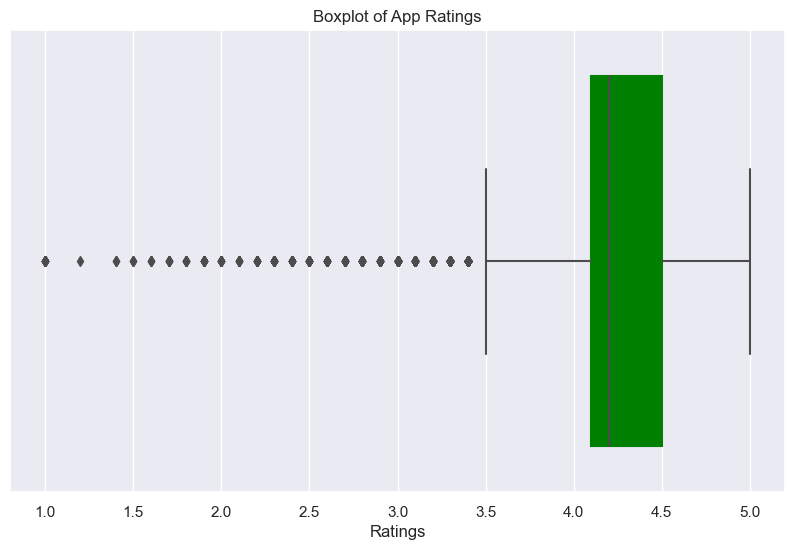
The left-skewed box plot above expresses the following statistical disclosures:
- The calculated mean (4.19) is lower than the median (4.30).
- Approximately 81.6% of accounted ratings range between 4.0 and 4.5.
- Instagram, Subway Surfers, and Google Photos are noteworthy products with striking attributes. They tout a remarkable average rating of at least 4.5, significantly overstepping the overall mean. Additionally, their active user engagement is sustained by the considerable installations and user reviews they garnered.
- 274 products received perfect ratings but recorded comparatively low downloads. These include Hojiboy Tojiboyev Life Hacks, American Girls Mobile Numbers, and Awake Dating.
In contrast, House Party - live chat, Speech Therapy: F, and Clarksburg AH registered the lowest ratings among their counterparts.
Reviews
Reviews are the unverifiable feedback, experiences and opinions of product users delivered in textual, video or audio formats. They encircle multiple attributes - product functionality, usability, reliability, design and cost-effectiveness. The graph below is a tally of the accounted reviews.
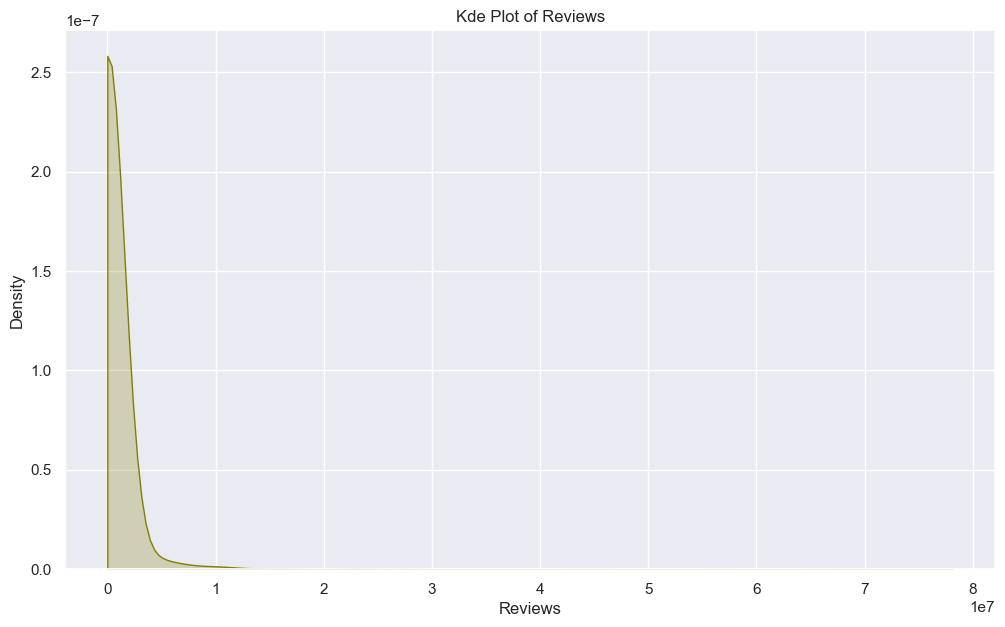
The Kernel Density Plot above denotes the bulk of products, recording less than half a million reviews. In addition to the statistical provisions it divulges, the violin plot below highlights the modicum of products which registered at least a million reviews.
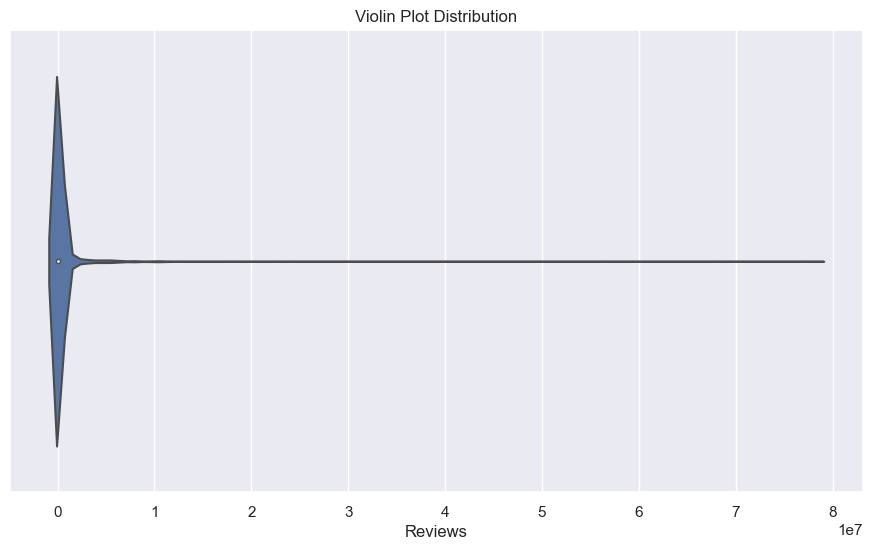
Below are some key statistical highlights of the Review analysis:
- There were 444,152 reviews on average, with a median of 2,094.
- The most reviewed products are Facebook, WhatsApp Messenger, Instagram, Messenger - Text and Video Chat For Free, and Clash of Clans.
- Facebook yielded 78,128,208 reviews, while the rest cumulated between 445,000 and 69.1 million reviews.
- Facebook recorded the lowest rating compared to its noteworthy counterparts.
- WhatsApp Messenger, Instagram, and Clash of Clans retained consistently striking ratings.
- The peculiar behaviour of Facebook affirms that exclusive dependency on review counts may not accurately distinguish highly rated products. The graph below illustrates this assessment.
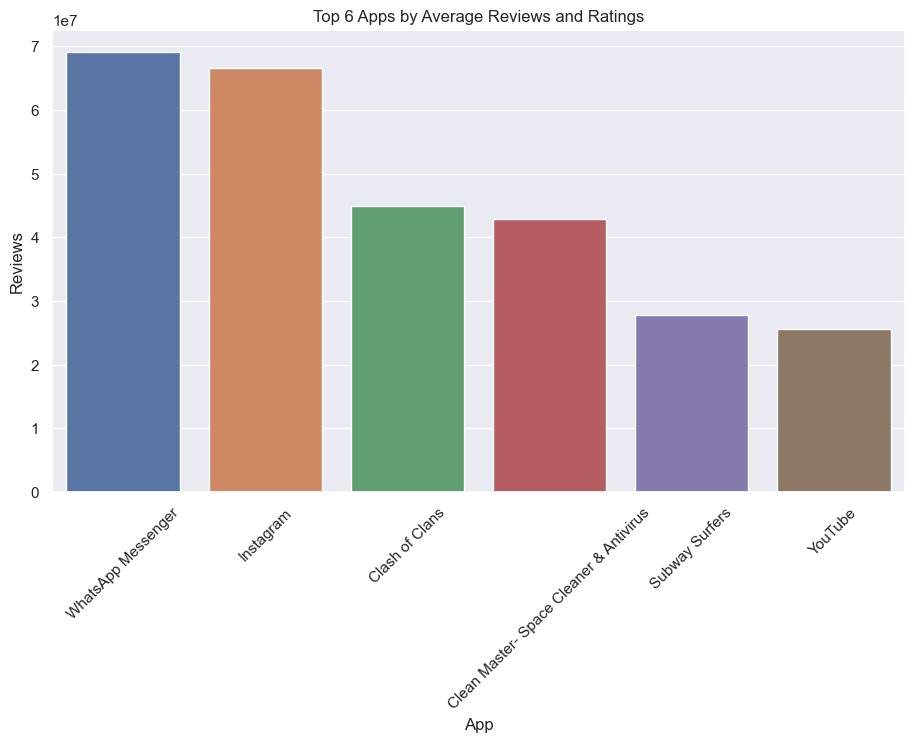
Installs
Overall, the products registered 15,464,338 installations on average, while the median installation count was 100,000. However, mean, as a measure of centrality, is tractable to significant outlier values hence the considerable disparity between the two metrics. The violin plot below, demonstrated by the juts along its tail, instances the disproportionate values swaying these sensitive statistical metrics.
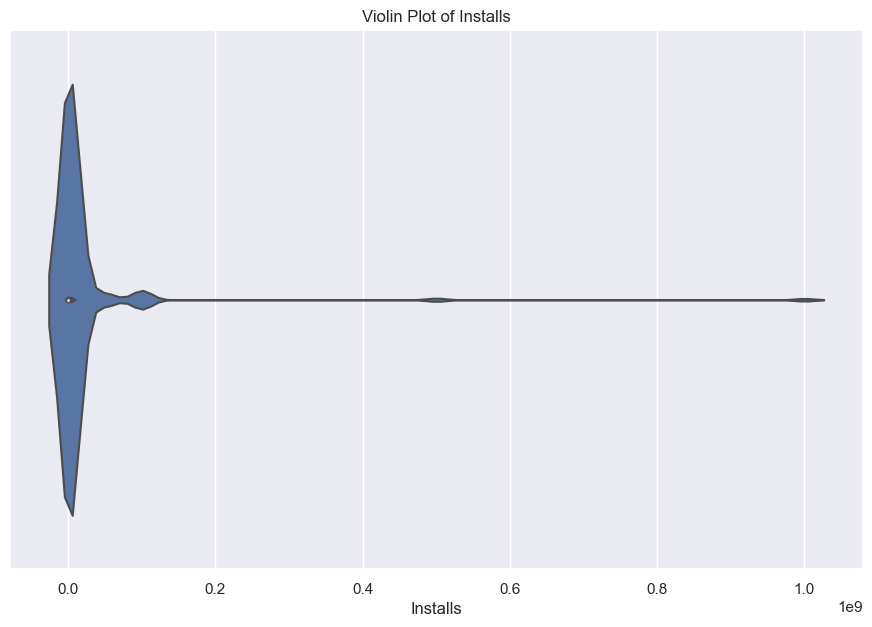
The umbrella-shaped section of the graph foregrounds a density of values between zero and fifty million (0.05 * 1e9). The discreet black-and-white figure at the centre attempts to underline the median value. Notably, 58 (0.5%) products recorded a billion user installations. Of these, Subway Surfers, Instagram, and Google Photos registered the greatest average ratings.
Price
Most products, 99.8% (10,816), cost $50 or less, and 92.6% (10,040) are free.
There are only 24 products priced above $50, all of which are variants of the I'm Rich Lifestyle App, the most pricey product at a whopping $399, but mediocrely installed.
In contrast, despite being premium, Minecraft and Hitman Sniper lept out with stately ratings of 4.6 and 4.5, respectively, and up to 10,000,000 installations. However, Minecraft costs $6.99, and Hitman Sniper only $0.99.
The violin plot below renders the statistical distribution of this attribute.
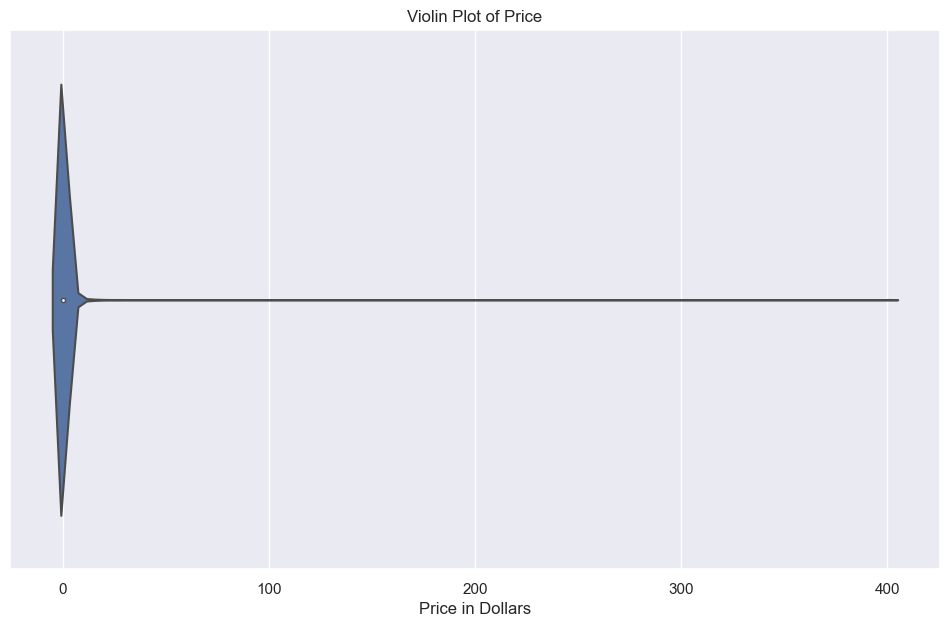
Feature Correlation
Next, I used the heatmap and pair plot below to recapitulate any interrelations between the numeric features.
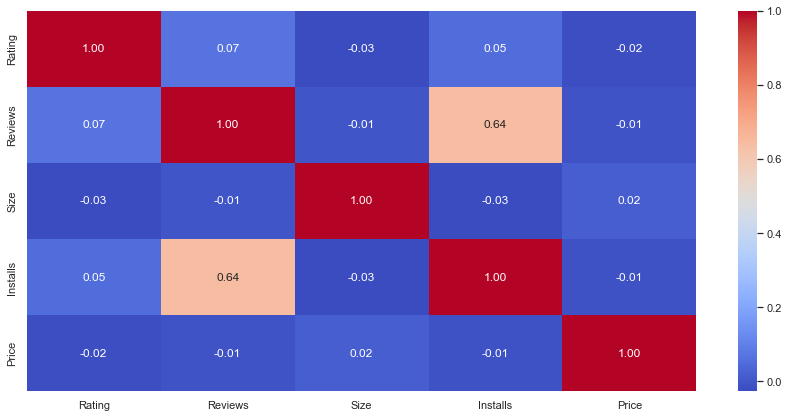
Except for the moderate affinity between reviews and instals, the heatmap reveals a prevailing mild relationship among the numeric type attributes. This outcome was unsurprising, considering the results of the earlier assessments. The pair plot below further scours the affinity between the numeric features.
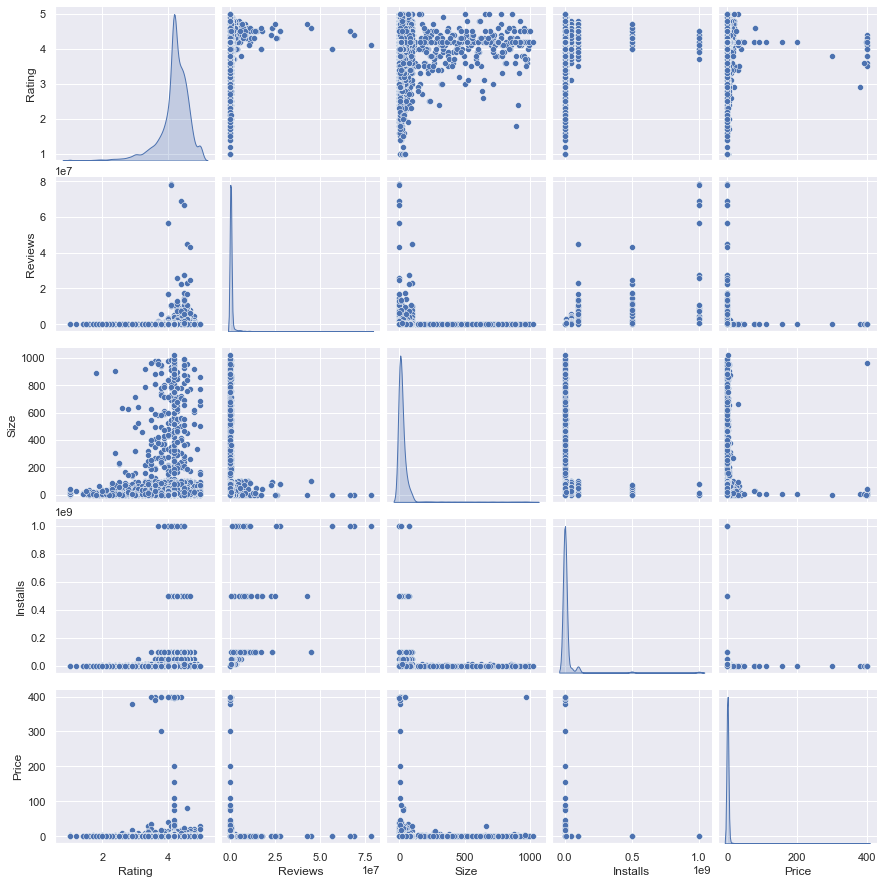
I sequestered Installs and Reviews because of their comparatively significant correlation, then rendered them using a scatter plot with a regression line to seek out any extraordinary inferences.
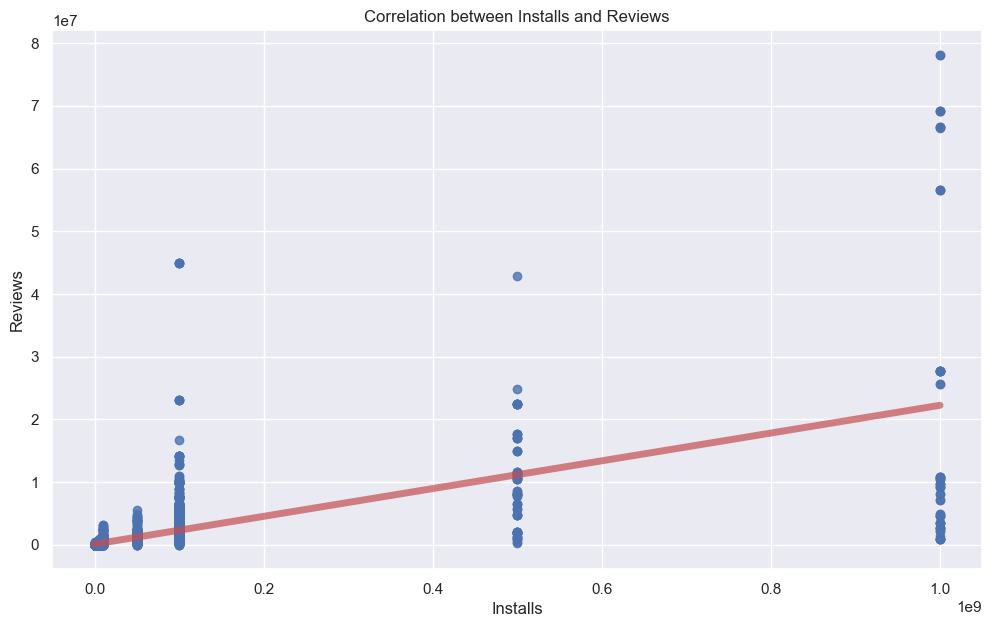
An echt positive correlation displays all data points along a regression line, and under less ideal scenarios, they are huddled closer to the regression line but not removed far from it.
However, this graph shows a more diluted theme. There is a marked distance between the regression line and a considerable number of data points for values on the independent axis, which de-escalates the intensity of the correlation between Installs and Reviews.
This demonstrates that both attributes largely hinge on the user's choice, so should not be sequestrated for deciphering any cogent inferences.
Business Insights and Recommendations
- Their blazing downloads and tiptop ratings indicate a high preference, making Facebook, Subway Surfers, WhatsApp, Instagram, YouTube, Google Photos and Clash of Clans eligible products for prospective advertisement and investment.
- Facebook, Subway, Instagram and Clash of Clans will benefit brands seeking visibility due to the manifest engagement inferred from their figures in instalments and ratings.
- Minecraft and Hitman Sniper are cut-price products game lovers can try. Their excellent ratings and striking downloads make the potential experience worthy.
- Most expensive products showed unremarkable user preference, evoking a consideration for cost-effective development strategies in product creation.
- The unconventional relationship between ratings and reviews recommends that prospective product users should favour both when deciding on a product.
Future Work
- I want to analyze sentiments using natural language processing methods to deeply construe user reviews, especially for notable products.
- With more data, I would explore the performance of competing apps to identify their strengths and weaknesses and benchmark them against industry rivals.
- Also, I would like to analyze app popularity and user engagement in different regions to aid the developers in deciding where to focus efforts for app localization and marketing campaigns.