Can scraping data and NLP techniques aid your understanding?
The skills I demoed here can be learned through taking Data Science with Machine Learning bootcamp with NYC Data Science Academy.
Spoiler: a little, but you still need to read the research!
Thirty-second summary
- I used a web scraper to extract publicly available research content from two of the top machine learning techniques (NeurIPS and ICML) over the period 2007-19 to generate a rich dataset of ~12,000 texts
- Unsupervised topic modelling is used to explore clustering of terms across research areas; however, manual topic creation more clearly demonstrates trends over time
- I also experimented with recent transfer learning techniques to develop a language model to generate โfake abstractsโ which might be quite hard to distinguish for non-experts
- Tools used: python, Google Colab, Scrapy, gensim, spaCy, regex, fast.ai libraries
Introduction
As a data scientist in training, I needed a fun project to experiment with scraping tools and Natural Language Processing (NLP) techniques. I also wanted a reason to familiarise myself with important machine learning (ML) research - so I designed an end-to-end process looking at progress in the area. This blog post will be structured to cover:
- Data collection - scraping the dataset from conference pages
- Text understanding - using a variety of techniques to explore language clustering and trends over time
- Text generation - experimenting with more recent development in language models
- Summary of key insights
My analysis focuses on content from two of the top annual conferences in the machine learning community: the Conference and Workshop on Neural Information Processing Systems (NeurIPS, formerly NIPS) and International Conference of Machine Learning (ICML). Both have grown substantially in recent years by various measures (attendees, paper submissions etc) - my analysis looks at various types of scheduled content (like poster sessions or workshops) and the growth in volume here is clear.
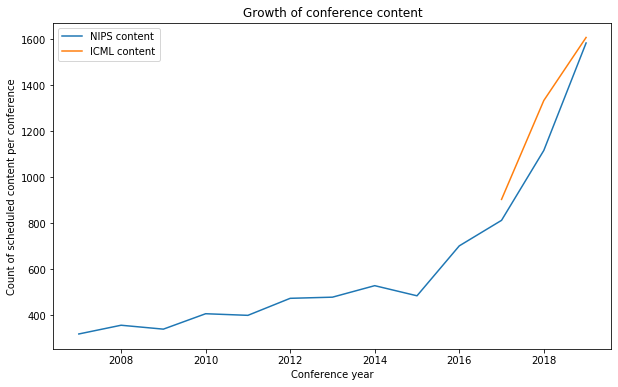
This post explores techniques taught in the NYC Data Academy as well as transfer learning models outlined in the FastAI NLP course (which I thought was fantastic top-down resource, available here)
1) Data collection
Core data is sourced from the NeurIPS and ICML schedule pages - conveniently these have very similar structures and formats, but I couldnโt find existing datasets collating this information (although some posts suggest people do this to optimise their own schedules at these busy conferences!).
I used the scrapy package to crawl c.12,000 web-pages to collate information on all session types including title, author, abstract and links - this proved straightforward with a combination of xpath and regex. The schedule captures a variety of session types, mainly poster sessions on specific papers but also varieties of talks and workshops.
I also explored data enrichment with a Kaggle dataset (here) which covers some NeurIPS information from 1987-2017 and helpfully includes the full paper text. Merging this dataset (using shared titles) means I have mixed availability through time - most of my analysis focuses on abstracts and titles in the period 2006-19 to maximise consistency and recency. Note this does create a bias to more recent years in the language modelling since there is simply more content, but I consider this to be ok.
2) Text understanding
Automated approach
First off, some simple word-clouds demonstrate how the key language has shifted between 2006 and 2019. To obtain these I processed the data in the standard manner to remove stop words and apply lemmatisation (see appendix).
It's clear immediately that "deep" networks and "reinforcement" approaches are referenced more now, while "Bayesian" and "inference" techniques seem less prevalent. "Representation" learning and issues relating to "efficiency" or "optimization" are also more frequently discussed.
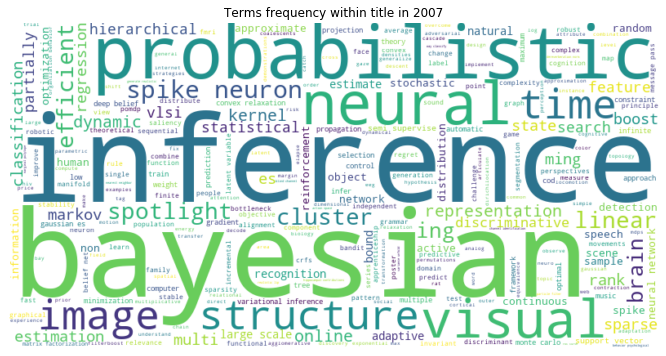
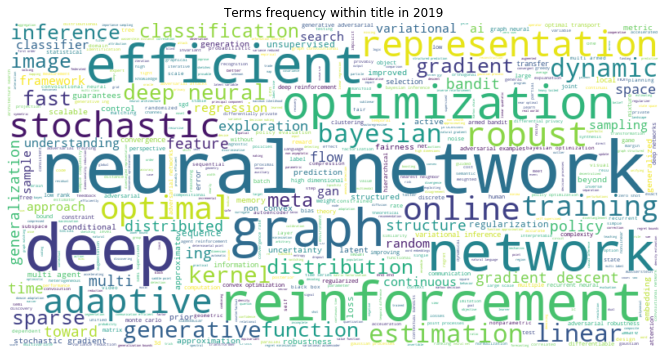
These sessions are not tagged or labelled in any way so it's difficult to explore trends. โTopic modelling via Latent Dirichlet Allocation (LDA) offers an unsupervised approach to examine clustering of words within documents. For a specified number of topics, clusters of co-occurring keywords are created - so topics represent a distribution of words and documents capture a distribution of these topics.โ
After parameter tweaking (see appendix) the approach does yield some success, generating ten categories which occur with similar frequency and have limited overlap. These are portrayed below via the pyLDAvis library as bubbles where the distance reflects a measure of the separation between topics.
Some of these topics have intuitive interpretations - I highlight topic 8 which is well-separated and captures a number of terms associated with reinforcement learning (like "agent", "reward", "RL", "environment", "exploration", "policy").
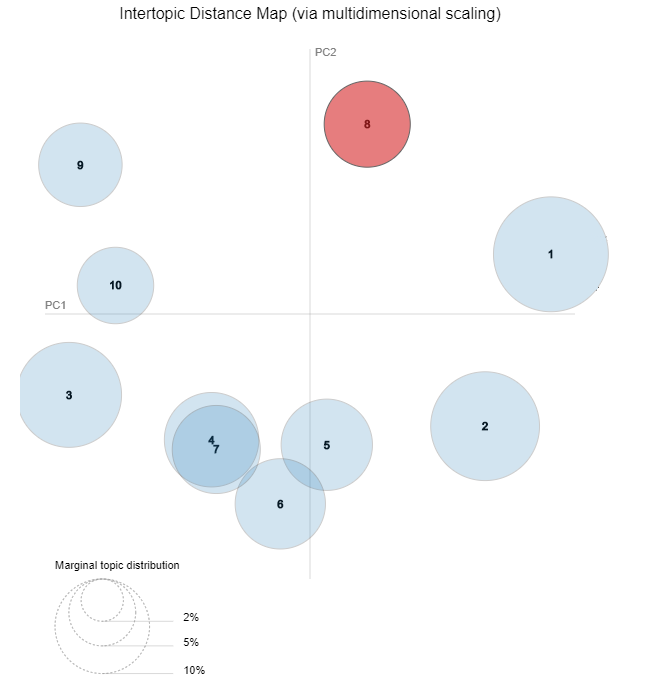
However, interpretation of many of the other clusters is more difficult and highly subjective. Much of the language is common across topics and the outcome appears very sensitive to the modelling configuration, so I'm hesitant to rely on this clustering.
I also applied sentiment analysis using textblob to explore any trends in optimism or pessimism - unsurprisingly the conference content was highly objective with medium-low polarity across time, in line with academic writing styles.
Manual approach
Using automated clustering does point a way forward - intuitive groupings can instead be generated by manually defining specific dictionaries. This does require some basic domain knowledge but I was helped by the LDA groupings and wordclouds above.
I considered references to different techniques/model architectures, domains/applications and specific datasets, looking at the % of summaries (abstracts) containing each topic by year. My approach used short dictionaries with highly specific terms to minimise false positives; this does mean that proportions are probably underestimated. Linear trends lines are used as more complex profiles are difficult to discern given the data volumes.
Whilst the approach is simplistic and reliant on the choice of dictionary terms, a few trends do jump out - the rise in "deep" "neural networks" specifically generative adversarial networks (GANs) or convolutional neural nets (CNNs), and the growing references to reinforcement learning.
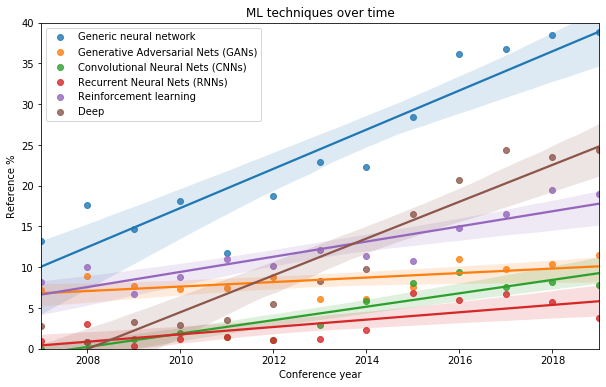
With static or slowly declining references to classic statistical approaches:
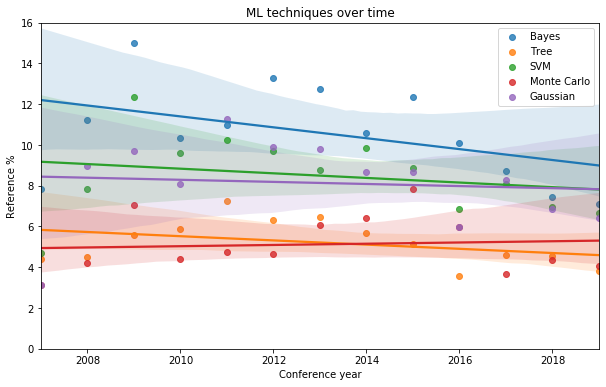
The approach suggests NLP and computer vision are the most commonly referenced domains. There is also some evidence that game-related analysis and ethical considerations are of growing relevance.
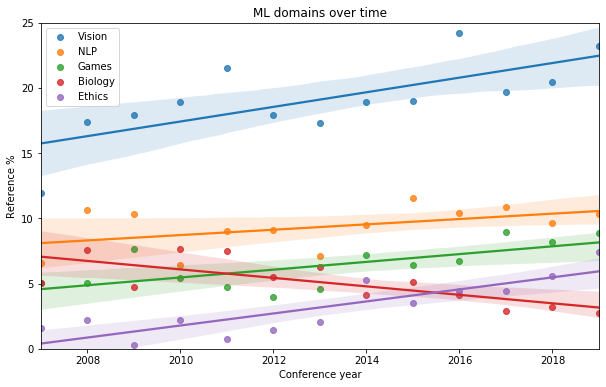
I also looked at the references to key benchmark datasets in the computer vision, hinting at the growing importance of CIFAR, MNIST and ImageNet benchmarks.
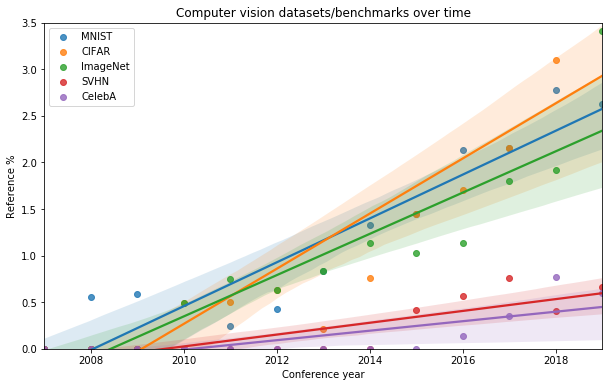
3) Text generation
As a somewhat separate challenge, I also wanted to explore the other side of NLP: text generation. This requires more complex modelling as the context and sentence structure matters unlike the frequency-based approaches explored above.
For this, I explore language modelling using transfer learning which requires a different pipeline:
- Switch back to raw text feeds (so no removal of stop words or lemmatisation)
- Use a pre-trained language model based on the English text in wikipedia (see appendix for details)
- Train the weights in the final layer using the documents in my NIPS/ICML dataset, to predict the next word on the basis of the prior word sequence
- Unfreeze the full set of weights and retrain the full network over c.10 epochs
- Seed the language model with an introductory phrase and generate a set of fake abstracts, using some randomness to produce variety (via the temperature metric)
Example
Picking an example seeded with the phrase โWe present":
We present a novel learning framework for incrementally learning in the state - space representation of a nearby channel 's learnable distribution . We show that this approach naturally generalizes the canonical exploration model to a wider class of structures than the possible neural network . Demonstrate a generalization of the sparse coding model to low dimensional sparse spaces . We demonstrate the effectiveness of our approach in a large range of source and target domains .
I think the result is quite good. Whilst there is no coherent meaning to this text, the structure of the sentences and overall passage seems reasonable and most of the phrases are plausible. I think this could feasibly pass as a real (yet incomprehensible) abstract for some audiences!
Note that this was just a quick experiment using the abstracts across all years - a more coherent passage might be achievable by training on a narrow topic and year group, using the full text from the papers as well.
4) Summary
This project has been a fun experiment with scraping and NLP which offers a few tentative insights:
- There do seem to be distinct vocabularies in some areas, especially reinforcement learning. But much of the technical language seems to generalise across approaches and domains
- Many of the publicised trends - ubiquitous neural networks, "deep" everything, the importance of computer vision and key benchmarks - are visible through simple analysis of the references in conference content
- Automated clustering is hard; domain knowledge proved more useful for categorising the texts
- Incoherent but plausible abstracts can be generated using transfer learning
Whilst key research papers and trends are summarised elsewhere, this process has highlighted several niche topics of particular interest to me which I may not have otherwise discovered. More importantly, I now have a broad and categorised dataset covering ML research over the last 12 years - this should serve as a useful resource to accelerate my data science learning.
Further work
There's plenty more work I would like to do in this area - the most obvious extension would look at author and citation information to explore networks and academic vs industry contributions. I did start to exploreโโ this but was constrained by request limits via Google scholar.
I would also consider redirecting this framework to another research area. For instance, could I quickly re-purpose the scraper and NLP code to explore trends in microeconomics research?
Appendix: technical notes
- Best results were obtained with the longer list of stop-words from spaCy (~330 words) with additional terms common to this source (~25 words like "program", "algorithm", "learning", "problem", "analysis")
- This analysis uses the fast.ai libraries with associated tokenisation. The language model is an RNN with the default dropout parameters and LSTM modules; underlying model here.
- For the LDA I explored optimisation across two parameters: the number of topics in the range 2-30 and the decay value in the range 0.5-1. The selected parameters covered 10 topics with alpha of 0.6
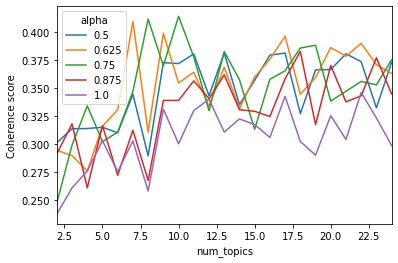