Home Data Analysis: Fitting Towards Ames
The skills I demoed here can be learned through taking Data Science with Machine Learning bootcamp with NYC Data Science Academy.
Introduction
Online real estate listing companies like Zillow, Trulia and Redfin each provide a home value estimator which is their estimate of how much a home is worth using their internal models.
The Zestimate from Zillow is among the most popular in the industry. While millions of homeowners, real estate agents and investors use the Zestimate as a benchmark to price a home, their pricing model is still proprietary. Thus users do not have adequate visibility into which aspects of their home or neighborhood most influence the Zestimate. What's more, oftentimes, homeowners are left wondering if the Zestimate is correctly pricing their homes.
Our objective therefore was to come up with a pricing model that values homes in the city of Ames more accurately than the Zestimate and to provide greater transparency to homeowners. By applying local knowledge of the housing market in Ames and fitting machine learning models to the local real estate market, we believe we can create a home value estimator with a higher explanatory power and greater transparency.
A table of initial results below shows how competitive our model is using results from our cross-validated training dataset:
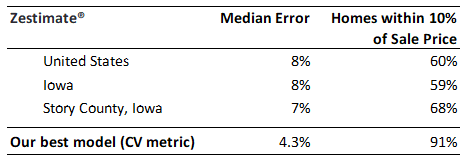
Before we dive into Ames and its real estate, let's also note that the Ames housing dataset comes from the city's property assessor's office, is fully public and easy to scrape. Zillow says it collects all relevant public data for each individual property, which makes our accuracy directly comparable to Zillow's.
Ames and the real estate context
Working with a housing dataset has one clear advantage: everyone can relate to the data and understand most features. We decided to explore the real-life context of Ames and its real estate market to get more intuition about the factors that determine house pricing.
Amesโ demographics, economy, quality of life and virtually any other aspect of the town is defined by the Iowa State University, a large public research university. Over 75% of Ames is either studying or working at the ISU, which makes the town essentially one large extended campus:
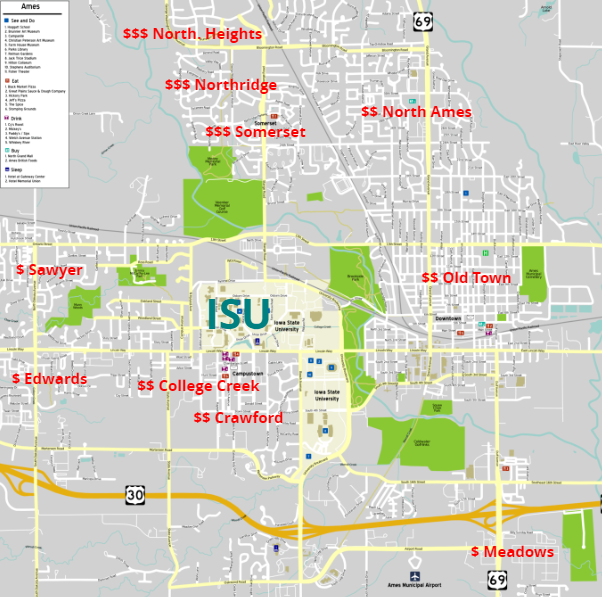
As in most college towns, the real estate market is defined by a very large proportion of rental properties. The income they generate depends eventually on the ISUโs annual budget, which helps explain the amazing stability of house prices (as well as transaction volumes) in Ames.
The Ames data was collected in 2006-2010 and this was a very turbulent time in the U.S. real estate (and the economy in general). However, none of that volatility has taken place in Ames:
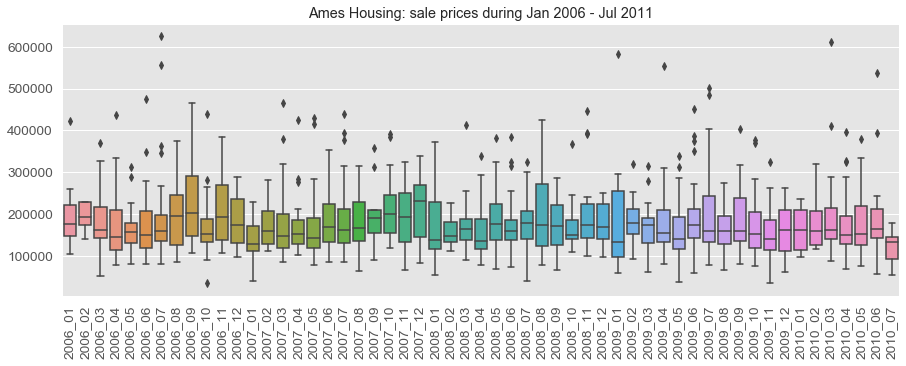
From the machine learning standpoint, such price stability means we cannot extract useful information from the timing of the transaction.
The last observation regarding the Ames real estate picture is that the dataset includes homes from very different price segments, ranging from upscale neighborhoods with $300,000 homes next to a golf course to the neighborhoods with $100,000 homes next to the airport.
The neighborhood and the zoning category strongly influence the house prices and came up as important features in all our pricing models:
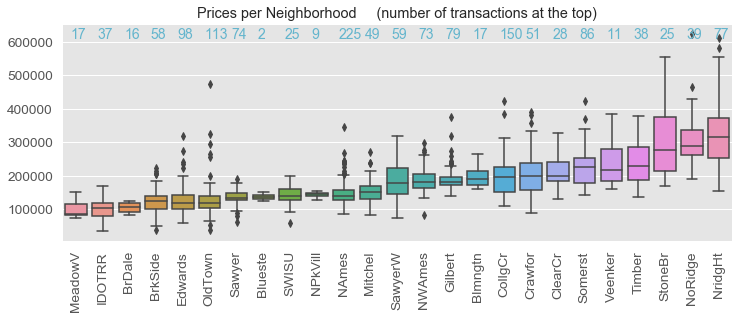
However, it is difficult for an algorithm to predict prices equally well for the entire spectrum. Our models perform better in the middle segments, where more transaction data is available. Note that it would be difficult for a human real estate agent to know all these different segments equally well, real estate agents tend to specialize.
Exploring the Data
The Ames housing dataset contains 2,919 homes evenly split into train (1,460) and test (1,459). Each home is described by a collection of 79 features (excluding ID), ranging from square footage to location to some elementsโ quality estimated by the tax assessor. This is quite a rich feature set which is why it explains about 92% of the variability in the sale prices, as we will see below.
Because we have such different market segments in the dataset, the relationship between the sale price and the above ground living shows a good deal of variability. For example, a 1,500 sq.ft home can cost anywhere from $100,000 or $250,00. We also observe a significant fanning effect: the prices vary more for the larger, more expensive homes.
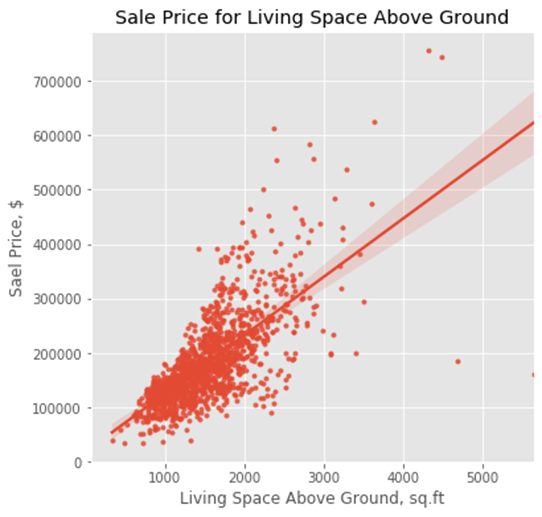
To compensate for this and make the price distribution closer to a normal distribution, we take the logarithm of the price and make it the target variable in all our models:
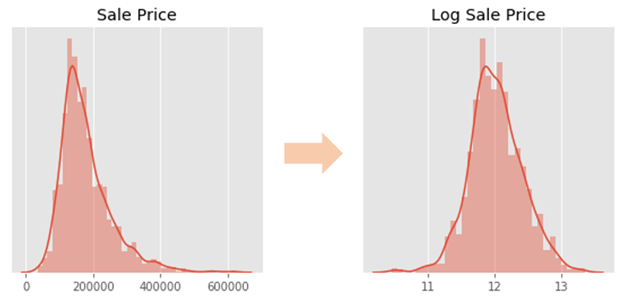
We have implemented a standard process of feature conversion, preprocessing, and imputation, which produced the features ready to be trained by ML models. After such preprocessing, some of the most influential features such as Overall quality, Kitchen quality, Exterior quality exhibit strong linear relationship with the Log Price:
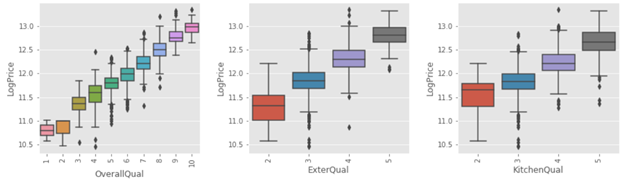
Modeling and Prediction Accuracy
Since the log price has a strong linear relationship with many predictors, we decided to deploy a few penalized linear models. We also implemented gradient boosted trees hoping to catch non-linearities or hidden feature interactions.
For each model, the best hyperparameters were determined through cross-validation, which estimated the actual out-of-sample metrics quite accurately. The optimization of the ridge linear model is shown as an example below, with the best R2 at about 92% and the best RMSE of the log price at 0.117.
The Ames housing dataset is sparse - only 1,460 training samples - and thatโs why the standard errors for the R2 and RMSE (the grey bands on the charts) are quite significant.
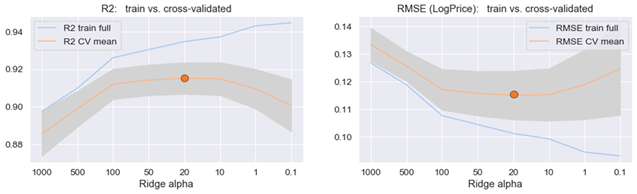
We optimized three models: LASSO, Ridge, and XGBoost. Although cross-validated performance was better for XGBoost, the test set metric (Kaggle score) was almost identical for all three models. This disparity was probably the result of overfitting the numerous hyperparameters for XGBoost, and the dataset is too small to allow for a proper train/validation/test split to avoid such overfitting.
We also deployed an ensemble of XGBoost and Ridge, and it showed the best results: 0.1169 RMSLE. The ensembling performed well apparently because the two components came from completely different families.
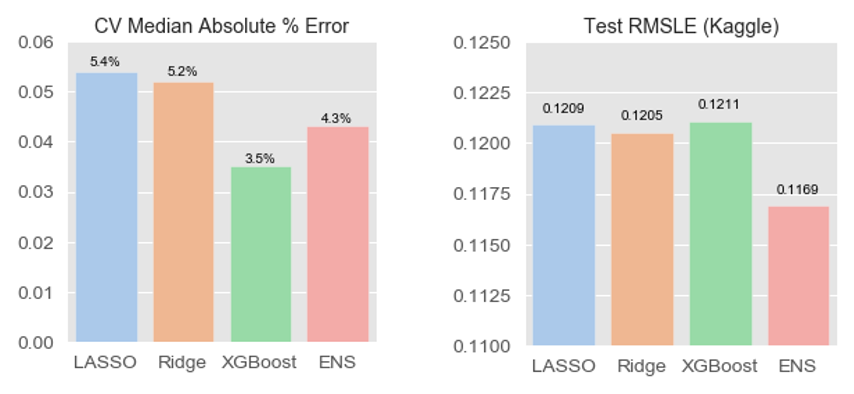
Our best result placed the team in the top 8% on Kaggle. However, this accuracy should be put in context. Improving the RMSLE by say another 0.002 would place us at the top-4% but, for an average house in Ames, this is equivalent to improving the prediction by a mere $160. Such fluctuations in price have to do more with noise than anything else.
To explain the remaining variability, machine learning models would need more data and more features, many of which are not accounted for by the Ames' property assessor office.
Insights - Value Proposition
While model results are impressive, we want to make sure the results are tangible to homeowners, local real estate agents and investors in the Ames housing market. To give that specific audience the needed transparency, we ranked the top features that contribute the most to the value of a home according to our best pricing model:
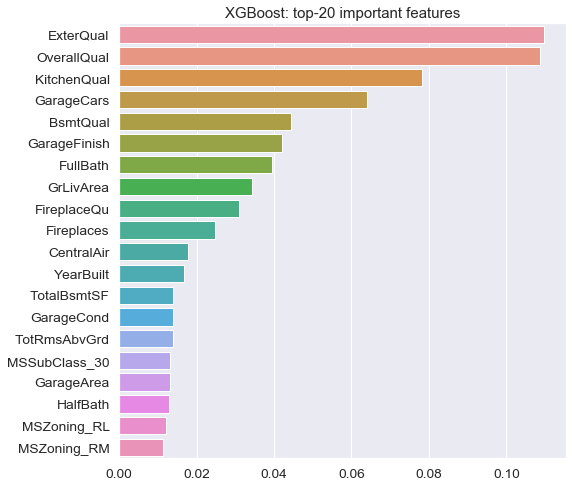
It comes as no surprise that overall home quality, condition, size and zoning are among the top influential features. Interestingly though, fireplaces and central air conditioning are important as well, perhaps due to the harsher winter conditions in the mid-west.
Re-assessing the best model
Even though our model performed well on the test (out of sample) data, we note that it tends to overprice lower value homes and undershoots at the higher end of the price spectrum as shown in the plots of residuals below. The market seems to demand an extra discount for the cheapest houses and on the other hand, extra premium for the most expensive ones.
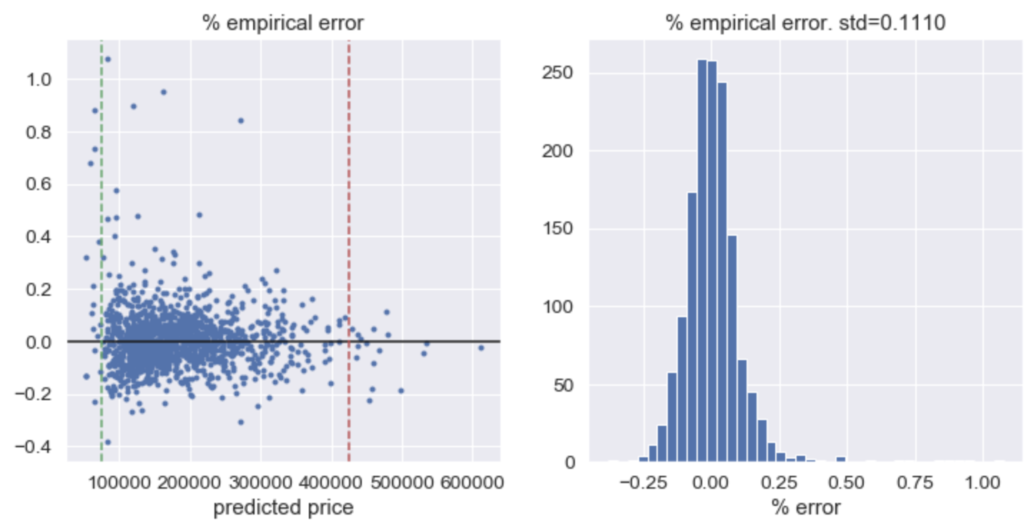
Conclusions
We can increase the prediction accuracy of our model in a couple of ways:
- Increasing the explanatory power through:
- Price segmentation and fitting to each price segment
- Adding more observations particularly to the tails of the house price spectrum. We note that this project only used sales data from 2006 - 2010.
- Adding more recent and non-standard features such as:
- Major upgrades to a home or immediate surroundings
- New developments that influence the desirability of a neighborhood
- Recent re-zoning
- More specific location data (e.g. near busy road or a frat house vs. close to a waterfront)
However non-standard data is harder and often pricier to obtain. Furthermore, price segmentation will increase the complexity of our model and make it harder to interpret results to homeowners.
Therefore, we conclude that our current model achieves a good balance between complexity and transparency while maintaining an accuracy competitive with the industry standard.