What do Great Thinkers Think About?
In the information age, we are bombarded with more and more information, the quality of which is often suspect. As Nate Silver points out in The Signal and the Noise, more data does not mean better decisions. We run the risk of forgetting the lessons of history and in particular the universal truths that great thinkers have distilled from deep contemplation throughout the ages.
For my web scraping project, I wanted to answer the following question:
What do the great thinkers think about, and what are the relationships between them?
Why is this an important question? Great thinkers expose us to new ideas, lead the advancement of progress and ultimately change the world. Their ideas teach us how to live a happy and spiritually splendid life.
To answer this question, I collected quotes from great thinkers, and developed a Shiny app which serves as an educational tool for exploring great thinkers and their ideas.
Data
The first challenge was to determine who the great thinkers were. Pantheon is a collaborative MIT educational project that provides a dataset of 11,341 historical figures spanning the past 6000 years throughout the world. They also provide some very cool visualizations of the data, as seen below.
Bias Alert
How does one define "historical significance"? Pantheon uses a fancy equation based on wikipedia pages: This is of course biased. By their metric, Shania Twain is more historically significant than Mark Twain. Their methods section discusses this bias, as highlighted below.
It is important to note this is a sample biased towards figures found on Wikipedia, and generally, in Western culture. Using the .csv file provided by Pantheon containing their historical list of figures, the next step was to scrape their quotes.
Scraping
The website BrainyQuote contains quotes of influential individuals, often accompanied by uplifting and cheesy images.
I scraped author quotes using the handy library Scrapy in Python. While scraping the quotes was a fairly straightforward process, the challenge was in connecting the Pantheon data set with authors on BrainyQuote.
The gist of the scraping algorithm is:
- Iterate through each figure in the Pantheon data set
- Search their name on BrainyQuote website
- Check that resulting page contains found authors
- Try to match the figure in Pantheon with the found authors
- If matched, scrape their quotes pages
- If not matched, add the name to a list of missing authors
The full scraping "spider" is given below.
Bias Alert II
We now have a second sampling bias: Only authors found on Pantheon and BrainyQuote are considered. The results of scraping reduced the number of figures to 4295 quotable humans: Less than half of the total on Pantheon. This is because many could not be matched exactly, as can be seen by a portion of the missing authors text file.
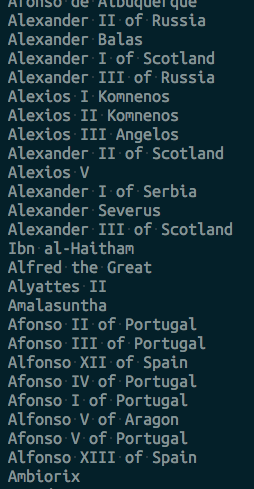
Afonso XII of Spain may have said some interesting things, but is unfortunately not included in this analysis.
Now that the biases and limitations of the data have been discussed, we can (finally!) move on to the model.
Vector Space Model
With author quotes scraped, I wanted to quantify their similarity and uniqueness. To do so, I employed the vector space model, often used in information filtering and retrieval systems. Briefly, the vector space model represents documents (d1, ..., dn) as vectors in an n-dimensional space, where each dimension corresponds to a word in the corpus. If a word occurs in a document, its component in that dimension will be non-zero. A query q is then represented as a vector and similarities between documents and the query are computed using the angle between them, as show in the graphic below.
For this analysis, a document is simply an author's consolidated quotes. There are several ways to assign weights to words in a document vector: I chose the popular method term frequency inverse document frequency (TF-IDF).
TF-IDF does exactly what its ridiculously long-winded name implies: It weights terms (i.e. words) by the frequency they appear in a document, and inversely to the frequency they appear in all documents. The latter weighting ensures terms that don't appear often in the corpus are weighted higher. For example, if only one document uses the word "ballet", we would want to weight that word higher than words that appear in several documents.
Upon quantifying the compiled wisdom into good old vectors, we can start to investigate.
Insights
I first wanted to investigate the effectiveness of the vector space model by seeing the most common words in various categories. Below is a breakdown of highest TF-IDF words by the domain of the authors.
The plot shows what we would expect: Quotes in a domain contain words related to that domain. Arts, for example, contain songs, film, actress, while exploration contain shuttle, astronauts, lunar etc. One interesting note is that the outdated term "tis" was marked in the humanities, indicating that possibly older authors are grouped primarily into this domain. 'Tis a shame we don't use this word anymore!
I then wanted to see how word usage changed throughout time. The highest TF-IDF terms by century is shown below.
Here we have some fascinating insights. Before 1000, "gods" (note the plural) is the highest term. As we move through the early centuries, we see words that are antiquated: iniquity, righteousness, doth, maketh etc. It is also interesting to see bodies, motion and gravity appear in the 17th century, coinciding to the scientific revolution when Newton formulated his theory of gravity. The 18th century contains government, rights, and liberty, indicating that this century was rife with political change, namely, the formation of the United States and the French revolution. The modern centuries reflect the skew in the data: The 19th century has "american" and "america", while the 20th century has "film", "movies", "films", and "actors". This is not surprising given the fact that the majority of authors on BrainyQuote are celebrities.
Author Similarity
As mentioned above, similarities between documents in a vector space model can be computed using the angles between them, which in practice uses the cosine similarity. Using this similarity we can construct a similarity matrix which stores all pair-wise similarities between documents. Below is the code in R I used to compute the similarity matrix of authors using the quanteda library.
Once the similarity matrix is constructed, we can find the most similar authors to a given author based on their quotes:
When we search for Aristotle, we get Plato, Ralph Waldo Emerson, Samuel Johnson, Francis Bacon etc. This seems like a reasonable result, but how can assess the similarity matrix quantitatively?
K-Nearest Neighbours
We want to get a sense of the goodness of fit of the similarity matrix. Ideally we'd have labelled data telling us which authors were similar to use in an assessment. In lieu of this data, I decided to use the similarity matrix to predict whether similar artists were in the same domain based on the assumption that similar artists should often be in the same domain. The idea is that if the similarity matrix reflects true similarity among authors, the model will perform better than random chance.
For the model I used the K-Nearest Neighbours (KNN) algorithm to predict an author's domain. Briefly, given an integer k and a point to predict, KNN selects the k closest points to the given point and classifies it based on the majority vote of the neighbouring points. For example, if k = 3 and two of the most similar authors of a given author are arts, the author will also be classified as arts.
Below is a graph depicting the accuracy over varying levels of k.
What is this telling us? For k=1, We get the best accuracy of about 70%, and as k increases the accuracy linearly decreases to about 50% when k=10. In other words, the most similar author is in the same domain about 70% of the time. This gives us some confidence that the similarity matrix is effective at representing similarity. We would not expect the accuracy to be too high, because similar authors aren't necessarily always in the same domain.
The goal of this project was to find similar thinkers, irrespective of their domains.
To further elucidate on this point, the Shiny app allows you to explore the authors in space and time, as seen below.
We see the civil rights activist Malcolm X, along with his quotes on the bottom and similar thinkers on the right. Notice that two of the three similar authors, Martin Luther King Jr. and Rosa Parks, are also social activists. The second most similar author, Paul Robeson, is actually a singer. This seems surprising until you read his biography:
Paul Leroy Robeson (/หroสbsษn/; April 9, 1898 โ January 23, 1976) was an American bass singer and actor who became involved with the Civil Rights Movement. (Source: wikipedia)
We see that he was a singer involved in the civil rights movement. This is an example of how the model finds similarities in the author's thoughts that extend beyond occupation, place and time.
Future Work
The vector space model could easily be extended to a content-based filtering recommendation system, which would allow users to like certain authors and get a list of recommended authors in the Shiny app. Similarity could also be improved by incorporating sentiment analysis and metrics such as occupation, geography, and time period.
Conclusions
By analyzing our data of the thoughts of the great thinkers, we see expected words in various domains, and interesting trends of word usage through time, including antiquated terms in the past as well as a bias towards celebrities in more recent times. A vector space model is a simple representation of authors that allowed us to compute similarities which was used to build an interactive Shiny app to explore great thinkers in time and space. The validity of the similariarities was assessed using the KNN algorithm which gave us some confidence that the model was working correctly.