Is there a clear path to success for startups?
Introduction
Imagine you're an eager entrepreneur who wants to know what it takes to increase your chances of success when founding a startup. You might ask yourself several questions:
- Where and when should I establish a startup?
- Which insights from leading industries can be applied to startups?
- What are the chances of success vs. failure?
This project explores these inquiries to better inform founders on what decisions to make to increase their chances of success.
Data and Pre-Processing
An informative source for startup data is Crunchbase, a website that aggregates information for startups, established companies, and investors, including what the company does, media postings, funding, and much more. Unfortunately, their website is purposely difficult to scrape to prevent bots from draining data property and server resources, so to circumvent this, used a data table from Kaggle called Startup Success/Fail Dataset from Crunchbase. This was a data-rich table (53583 rows, 14 columns), though it required some feature engineering.
For pre-processing I created and transformed several columns to better visualize the data:
- Change date columns from string to datetime
- Create year and month columns for founding year, first funding year, and last funding year
- Calculate duration between various landmark years (i.e. years between founding and first funding)
- Normalize total funding raised by rounds
- Create a country name column from country code
- Create an industry column with each value a single industry name
Data Limitations
One caveat with this dataset is that there isnโt any information after the year 2015. Consequently, the findings will not be based on the most current trends. Regardless, there are plenty of impactful insights from the nearly 100 years of data.
Analysis:
Where and when to establish a startup?
Based on this dataset, the United States, Great Britain, Canada, India, and China are major global hubs for startups; however, the United States has by far the greatest number of startup companies among the countries. In fact, Great Britain, which is the country with the second most startups, has ten times fewer companies than the United States. The disparity between the US and the rest of the world can be clearly shown in Figure 1, a heat map that shows the count of companies per country.
Figure 1. Heat map of number of startup companies per country
Not only does the US have the most startup companies, it also has the greatest number of startup industries, as shown in Figure 2. Based on this analysis, the USโ exponential rise in startup industries in the 1980s occurred years ahead of the other leading startup nations, showing its legacy of ingenuity and focus on entrepreneurship. This boom in US startup industries could have been the catalyst for the surge in other nations that followed.
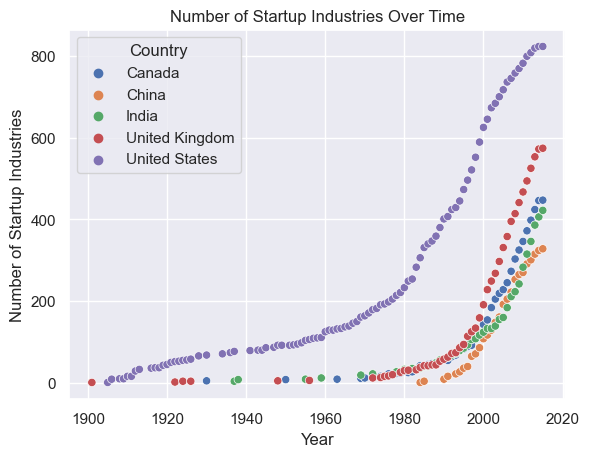
Figure 2. Number of startup industries per country over time
Now focusing within the United States, performed a similar analysis but shaped the data by city rather than country (Figure 3.) While New York has consistently had the highest number of startup industries, the US cities with the most startup industries were relatively similar in density until the early 2000s. However, since then, New York and San Francisco saw a significant increase in new industries, distinguishing them as the global hubs for startups.
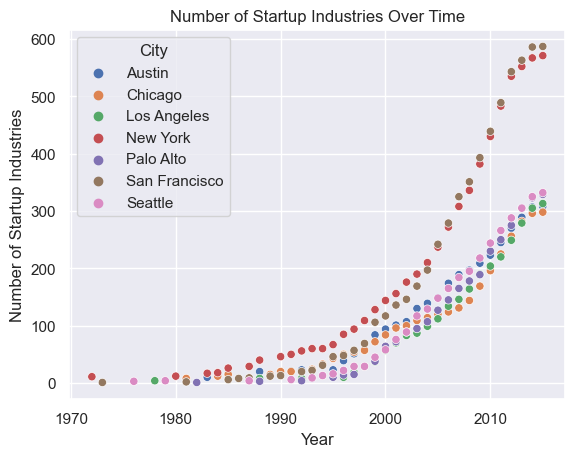
Figure 3. Number of startup industries per US city over time
In trying to understand if certain times of year are more or less ideal to start a company, I analyzed the number of startup companies that received their first round of funding during each month, as shown in Figure 4. Based on these results, most months have a relatively similar spread in the number of companies that received first-round funding. There are, however, two months with standout distributions:
- December has a lower 75% quantile
- January has a higher 25% quantile/median
The hypothesis for why there are differences in these particular months is that investors and companies likely shut down operations during December due to the holidays, reducing the number of deals. Therefore, those discussions are pushed to the new year, increasing the number of funding rounds in January.
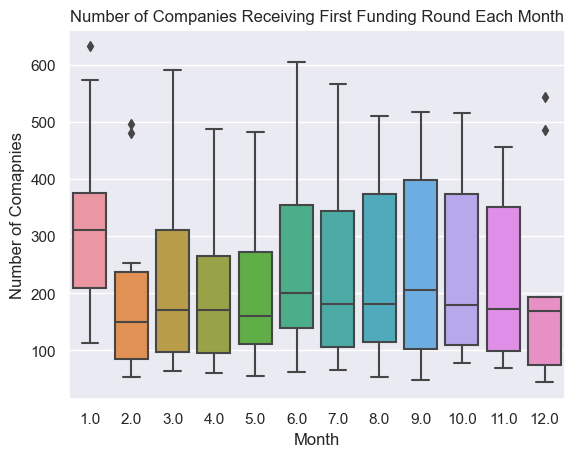
Figure 4. Distribution of the number of companies that received their first funding per month
Assuming one has the privilege to start a company anywhere in the world, this analysis suggests that the ideal location is within the United States and specifically, in New York or San Francisco. This recommendation is based on the assumption that being in an area with a large number of startup companies and industries would offer unparalleled access to networking and talent pool. In terms of what time of year to plan to raise the first round of funding, there isnโt an optimal month to do so.
What are the insights from leading industries?
Based on this data, Software, Mobile, Biotechnology, E-Commerce, and Social Media industries have the greatest number of startups. Therefore, the subsequent analysis will be focused around their trends.
Figure 5. Top 15 industries with the highest number of startups
An aspiring founder would be interested in understanding the average value for a first-funding round to bolster negotiations with investors and also anticipate resource allocation post funding closure. In Figure 6, assessed how the average value of first-funding rounds have changed over time and observed a significant increase in the mean around 2000, ranging from 12-27 million dollars. However, in 2005, there was a downward shift in the mean that remained relatively constant for the next decade. Based on the last decade in this dataset, a founder should expect around 3-15 million dollars for a first round of funding.
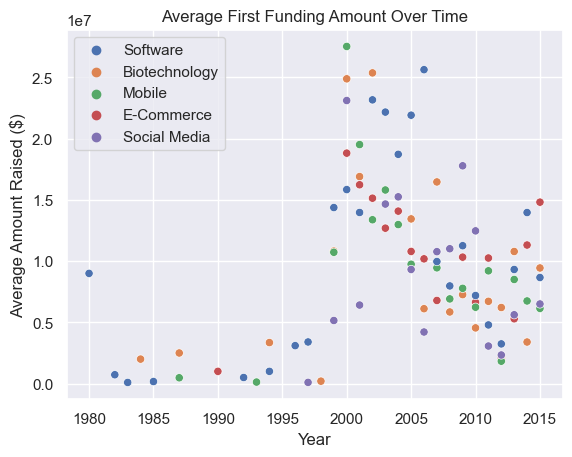
Figure 6. Average value of first-round funding per year within top 5 industries
Interestingly, when investigating the total sum of first-round funding received per year, the total amount of capital raised per year continued to increase despite the downward shift in average fundraise size (Figure 7.) In other words, venture capital firms and investors are spending less per deal, but as a whole, more money is being raised for the startup industry. Therefore, is this relationship caused by an increase in the number of investors or deals?
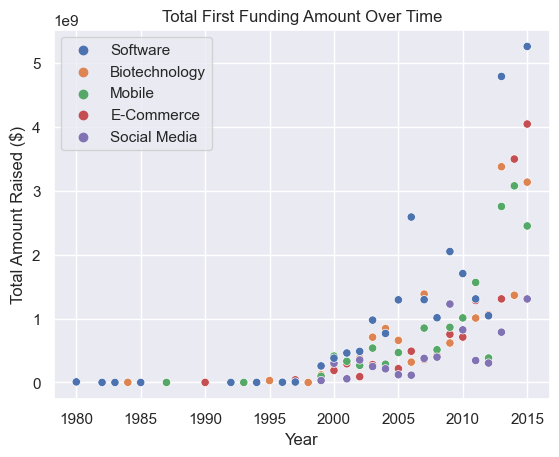
Figure 7. Total sum of first-round funding per year within top 5 industries
To answer this question, I investigated the total number of companies that received their first-funding round per year, as shown in Figure 8. Based on this analysis, itโs clear there was a significant increase in the number of first-funding rounds between 2005-2014, which would explain the increase in total startup spending despite the decrease in average fundraise size.
Interestingly, there was a decrease in the number of startups receiving first round funding between 2013-2014 and then a negative slope between 2014-2015. An initial thought was that this dip was due to poor data integrity. However, it was confirmed that the dataset had these results through the entire year of 2015, indicating a real downturn. That raises the question: what is the cause?
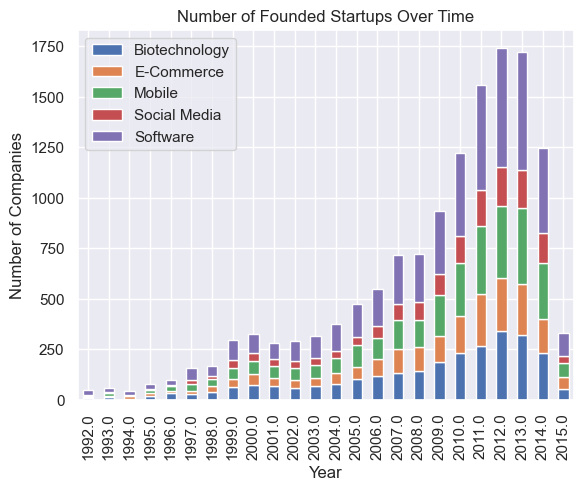
Figure 8. Total count of number of companies that received first round of funding within top 5 industries
The hypothesis is that the downward shift in companies receiving first round funding is due to a decrease in the number of founded companies two years prior. When overlaying the number of companies that were founded and first funding per year, itโs clear that both metrics observed an increase during the first decade of the 2000s (Figure 9.) However, in 2011-2012, there was a tipping point in the number of companies founded per year.
Focusing on years where the number of companies founded and received first funding peaked (2012-2014) revealed that there is a difference of around two years between both metricsโ high points. In fact, this delta of two years correlates well with historical data, in which the average number of years between founding and first-funding is 2.67 years (Table 1). Therefore, the decrease in the number of companies receiving first-funding from 2014-2015 is due to fewer companies being founded after 2012.
Figure 9. Number of founded and first-funded companies per year
Table 1. Summary statistics for durations between founding vs. first-funding for startups
When gaining insights from the leading startup industries (Software, Mobile, Biotech, E-Commerce, and Social Media), I learned that the average value of first-round funding had decreased from the early 2000s, landing between 3-15 million dollars. However, although the average value of fundraising has decreased, more companies are receiving first funding and the average time between founding and first funding is 2.67 years. All of this information would be extremely impactful for the founder's planning and resource allocation (i.e. managing burn rate, establishing hiring plan, asset management, etc.).
What are the chances of success vs. failure?
This dataset categorizes each company status by either โoperatingโ, โacquiredโ, โIPOโ, or โclosedโ. In this project, the metric for a successful company is one that is acquired or IPO, and a failed company is one that is closed. While not all founders aim to launch an IPO or be acquired, this analysis assumes that founders are interested in making a return on their shares.
After calculating the frequency for each company status, itโs clear that chances of success are quite slim (Figure 10.) The majority of companies are operating (79.2%) and IPO is the least common status, having a frequency of only 2.69%. The frequency of being acquired vs. closed are similar, ranging from 8.94-9.17%, respectively.
Figure 10. Frequency of each company status (acquired, closed, IPO, and operating)
Interestingly, even when calculating the status frequencies for the five biggest startup industries (Software, Mobile, Biotechnology, E-Commerce, and Mobile), similar results are observed (Figure 11.) Therefore, founding a startup within the โhottestโ industries alone does not change chances of success or failure.
Figure 11. Frequency of each status (acquired, closed, IPO, and operating) for the top 5 industries
During this study, investigated if there was a relationship between successful/failed companies and the total amount of funding raised (Figure 12.) The hope was that the amount of funding raised could be a Key Performance Indicator (KPI) and a benchmark for how the startup was performing compared to historically successful/failed companies. During this process, successful companies (acquired, IPO) have higher median than other statuses. However, there are many closed companies that raised more money than IPOs or acquired companies and visa-versa. Therefore, thereโs not a clear relationship between success and total funding.
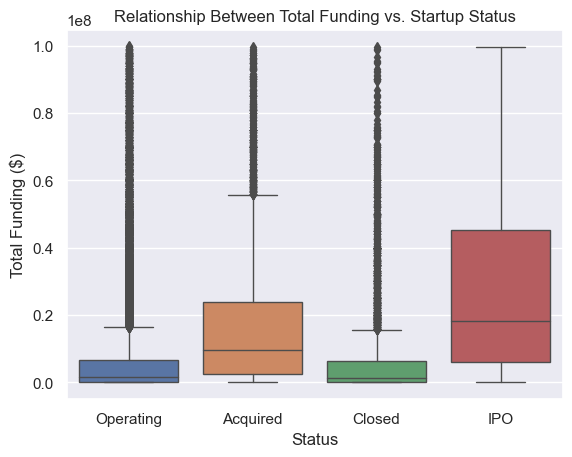
Figure 12. Distribution of total funding raised by each company status
Another KPI that could be potentially used as a performance benchmark is the number of funding rounds for successful vs. failed companies. Therefore, plotted the distributions for the number of funding rounds for each company status (Figure 13.) This shows that successful companies have higher medians than other statuses. However, again, there are many closed companies that have more funding rounds than IPO or acquired companies and visa-versa. Therefore, thereโs not a clear relationship between success and number of funding rounds.
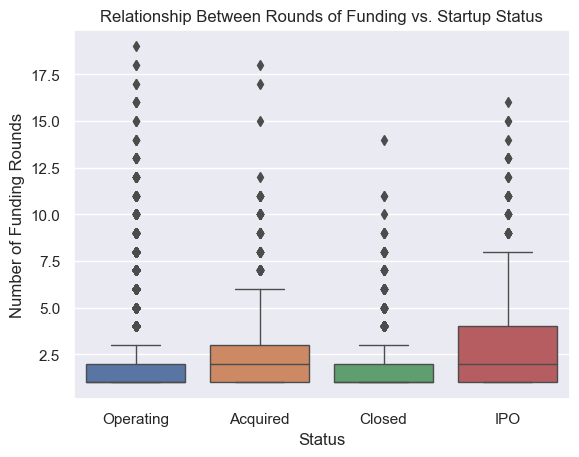
Figure 13. Distribution of number of funding rounds by each company status
Finally, in exploring the behavior of successful vs. failed companies, studied the relationship between the number of funding rounds vs. capital raised (Figure 14.) This could help founders anticipate the number of rounds needed to raise desired capital and be a point of reference for their rounds' performance. As expected, there is a positive, linear relationship between the number of funding rounds vs. total funding raised. However, there is significant variation, suggesting that using status and number of funding rounds alone will not accurately predict total funding raised. It is clear that IPO companies have a similar slope to the other statuses, though the higher y-intercept suggests that IPO companies generally raise more money per round.
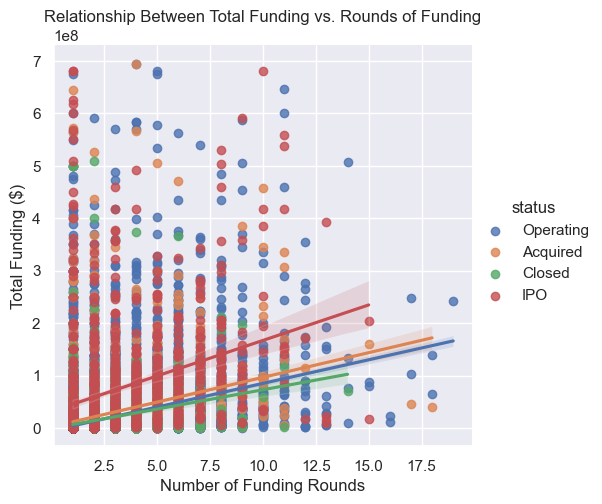
Figure 14. Relationship between number of funding rounds vs. total funding raised, colored by the various company statuses
During this analysis, I learned that the chances of success are 2.67% for IPO and 8.94% to get acquired, while chances of failure is 9.17%. Also, the relationship between status and total funding raised or number of funding rounds showed that successful companies had higher medians for both metric, though there was significant variation. Overall, there wasn't a clear path of success using the features in this dataset.
Conclusions and Next Steps
Although there clearly isn't a clear path of success for any startup, this analysis synthesized a powerful data package with actionable, data-driven takeaways and invaluable benchmarks for steering company-wide planning (Figure 15). Whether itโs a better understanding of where to build a company or how much time it takes to achieve the first round of funding after founding, these insights are extremely useful guides for a potential startup founder.
Figure 15. Data package from this analysis
In the future, this analysis could be expanded upon by:
- Applying inferential statistics
- Applying classification machine learning to predict outcome (i.e. IPO, closure, operating, acquired)
- Using an updated dataset to gain insights from the last decade of startup trends
- Exploring why there the number of founded startups around 2011-2012 declined