Using Data to Predict House Price with Machine Learning
Data Science Introduction
Purchasing a new house is always a big decision! Is it the location ? Is it the overall quality of the house ? Is it the size ? Could it be sold at a good price in future? Moreover, economic growth plays an important role as housing demand is often seen as elastic in terms of income, leading to an increase in revenues for households. In this text we will use data to predict house price with machine learning.
Undoubtedly it is a tough call to consider which features should be of most importance. To ease this decision-making, in todayโs world, Machine Learning allows an entrepreneur on forecasting the house price with a maximum accuracy of market trend and building model of the historical dataset on โwhat happened and whyโ to predict โWhat is going to happenโ.
In this blog, a step-by-step technical approach have been described for predicting house sale price for a Kaggle data-set of Ames, Iowa. The project endeavors to extensive data analysis and implementation of different machine learning techniques in python for having the best model with most important features of a house on insight of both business value and realistic perspective. The dataset consists of 79 different features for 1460 houses in Ames which can be used as training data to predict the sale price of another 1459 test data set of machine learning model.
Process Steps
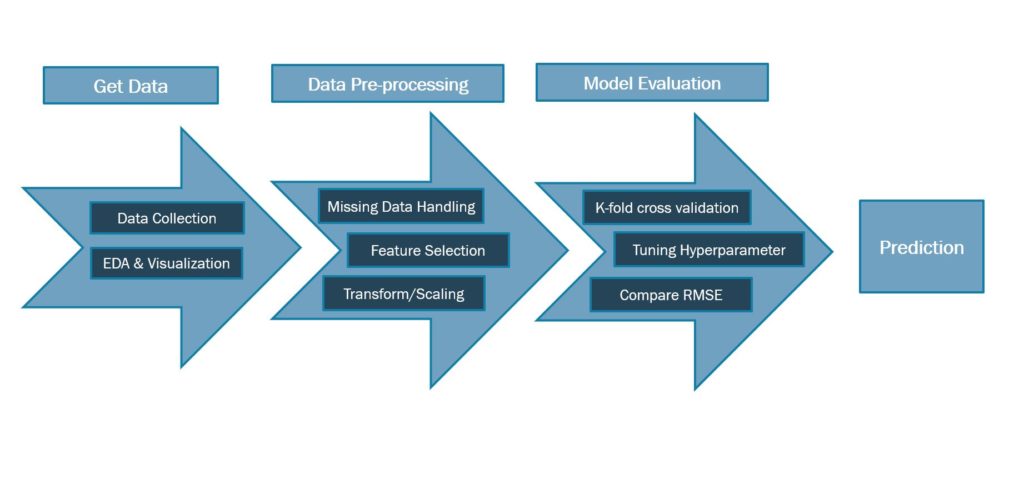
The entire process of machine learning can be divided in 4 main steps to get the desired prediction.
- Get Data โ Data can be collected from the source in this step for exploratory data analysis and visualization for understanding the current/historic data and determine the next step.
- Data Pre-Processing โ Pre-Processing aka data wrangling is the technique of cleaning and transforming the raw data (which can be incomplete and inconsistent) to a proper format to be used for modeling.
- Model Evaluation โ Different machine learning algorithms can be used and evaluated in this step to measure the accuracy and other performance metrics.
- Prediction โ Depending on choice of the best model, prediction is done.
Exploratory Data Analysis
This is the way of analyzing, visualizing, summarizing and interpreting the dataset to achieve some insights on statistical measure and validate the hypothesis.
For this dataset, the first step of EDA is to check the distribution of Sale Price on train data-set. Itโs observed in the histogram that the data is rightly skewed with a skewness of 1.88 and kurtosis as 6.53 which needs a log-transformation to correct the distribution in feature-engineering step.
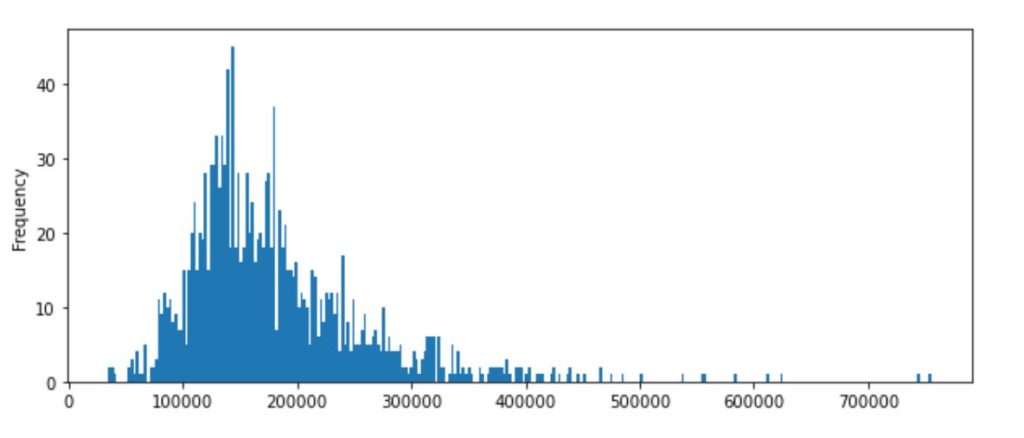
In the next step, further data analysis done to reveal that the percentage of houses built before 1980 is 58.7% and percentage of houses remodeled is 47.8% as Ames is an old place.
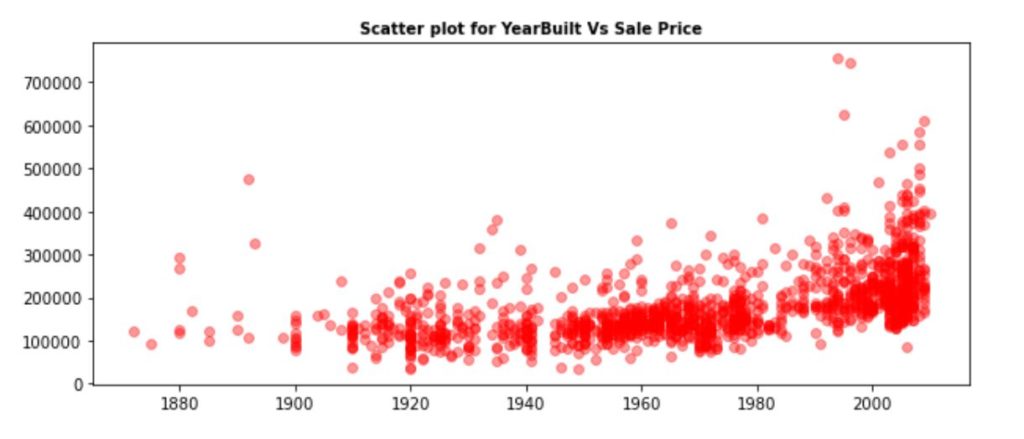
Correlation Analysis - Correlation analysis helps to identify top features highly correlated with Sale Price. Multicollinearity found as well for couple of features which needs to be handled in feature engineering step to get best model.
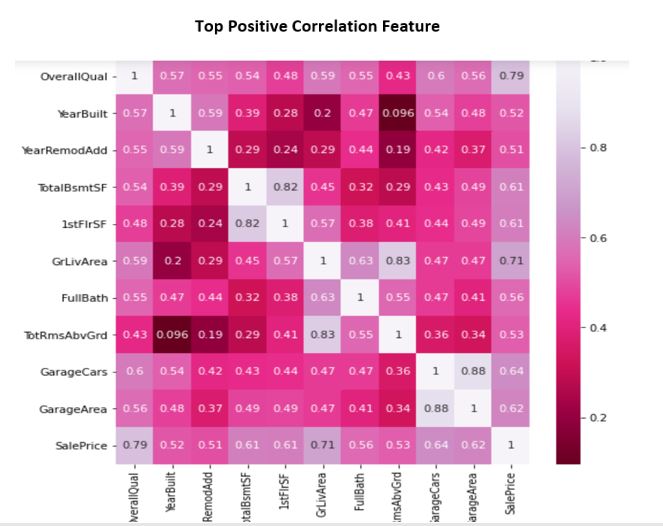
Outlier Analysis - Couple of outliers found for Living Area, Lot Area and neighborhood which can be removed in pre-processing. Since the train data does not contain school district information and crime rate, neighborhood is an important factor, implying above factors. It's observed that the variance of an expensive neighborhood is typically higher, which explains the skewness of the sales price density as well.
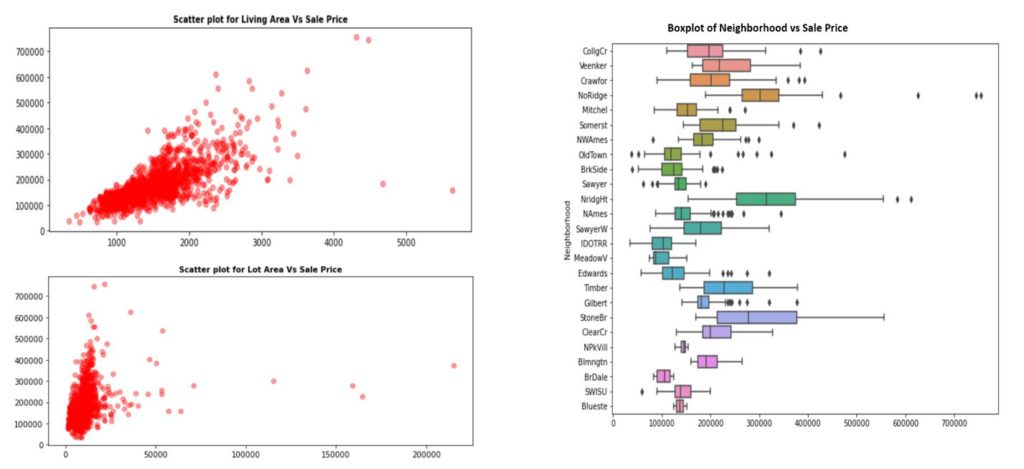
Pre-Processing
Missing Data Handling - Overall, 2.68% data is missing in the training data
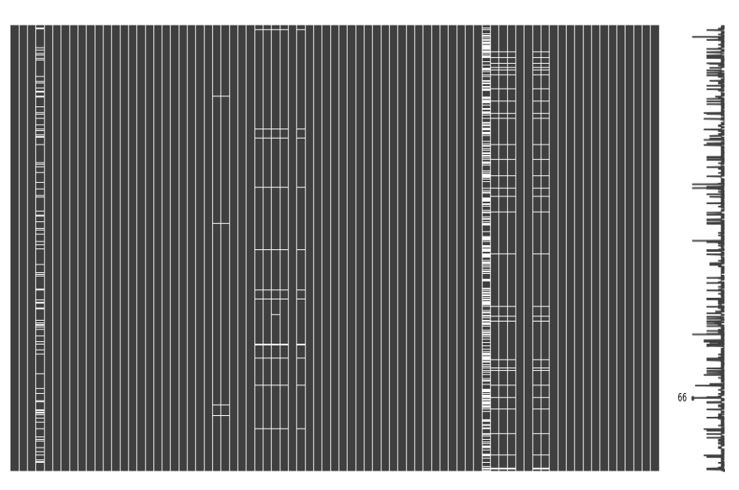
Below are the steps done for missing data
- As PoolQC, MiscFeature, Alley and Fence features have more than 90% data missing, dropped these features as there will not be any impact.
- LotFrontage missing values imputed based on median value of neighborhood LotFrontage.
- The features having many missing data but having correlation with sale-price less than 0.5, have been dropped as there will not be impact.
- For some numerical predictors, missing values are imputed as mean value or 0 based on analysis. For some categorical predictors, missing values are imputed as NA or the value mostly used.
Feature Selection/Engineering - This step identifies the selective features needed and having most significance with the target feature.
- Log transformation applied to SalePrice to correct the skewness.
- 4 new features created to get more business importance from usability standpoint โ TotalSF, Total_Bathrooms, Years_SinceRemodel,HasGarage.
- Some numeric predictors dropped to avoid multicollinearity based on correlation analysis. Outliers removed from the training data accordingly.
- Predictors having negative correlation with Sale Price โ dropped.
- Categorical predictors dummified to use in regression process and some of them dropped if only one value is dominating.
Model Evaluation
Model selection and evaluation is a critical step of any machine learning project as identifying the pattern and applying the correct algorithm is not a very easy process. Machine Learning provides multiple number of models to generalize it to the unseen data from the same population and measuring the performance.
Along with meeting the business objective, the model should take care of accuracy, execution time, complexity and scalability as well to be considered as best model. Sometimes size of training data set and numbers of predictor features can be decision-making criteria for model selection. RMSE (Root Mean Square Error) is the performance metrics for this project.
For this particular dataset, it's observed to have linearity and normal distribution of Sale Price data, which directs to evaluate the linear regression model first. To reduce the overfitting issue, both Ridge and Lasso also comes under consideration for evaluation. To check whether the non-linear models outperform, Random Forest and XGBoost also added. Firstly to split train-test data set, 10-fold cross validation method applied which shows an interesting statistics as below:
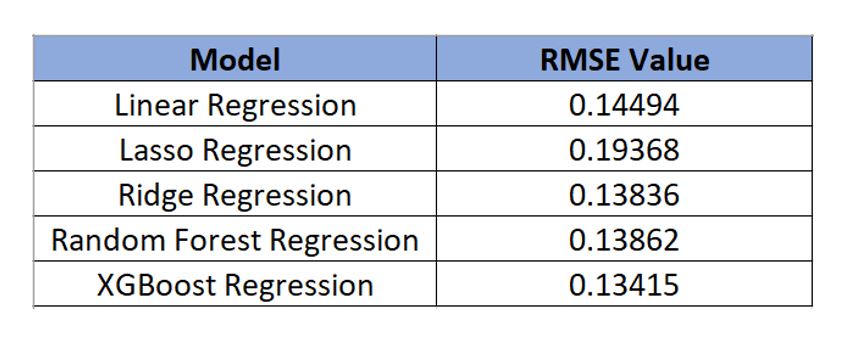
Performance metrics of RMSE resulted to be very close for Ridge, Random Forest and XGBoost. As Random Forest took significant time to get the result, further analysis is done between Ridge and XGBoost for the best model selection.
For Ridge, by introducing an additional hyperparameter lambda, a fine-tuned penalized regression trades off a slight increase of biases for a significant drop of its model variance, which results in an improved accuracy. With tuning the hyperparameter setting alpha as .01, the best RMSE and R-Square value received for this model in training data which reduces the risk of overfitting to a great extent.
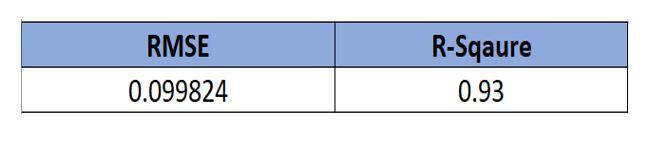
Bur exploring the feature-importance of the ridge model, it is not convincing that most important feature of this model will meet the business value.
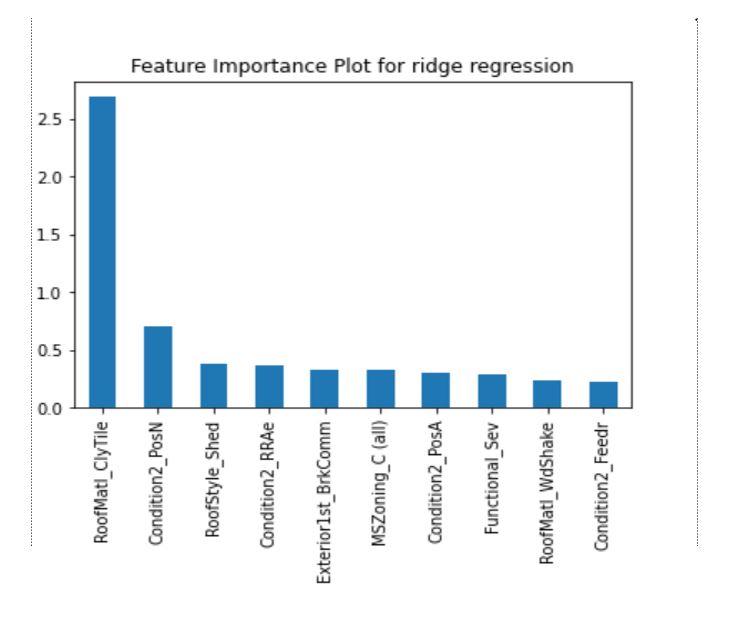
As a next step, XGBoost is analyzed thoroughly through grid search to get the best parameter value of max depth and n_estimators. XGBoost is a tree based ensemble machine learning algorithm which is a scalable machine learning system for tree boosting. XGBoost stands for Extreme Gradient Boosting.
It uses second order partial derivatives as approximation of loss function to provide more information about the direction of the gradient and the way to get to the minimum of the loss function. That algorithm smoothens the final learnt weights and thus avoids overfitting. Grid search resulted best max_depth as 3 and n_estimators as 100. Using these values as parameter, the best model performance achieved as RMSE of .0616 and R-Square as .97. The feature-importance bar chart also resulted very realistic in terms of business features of a house sale.
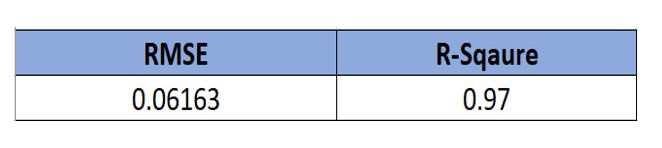
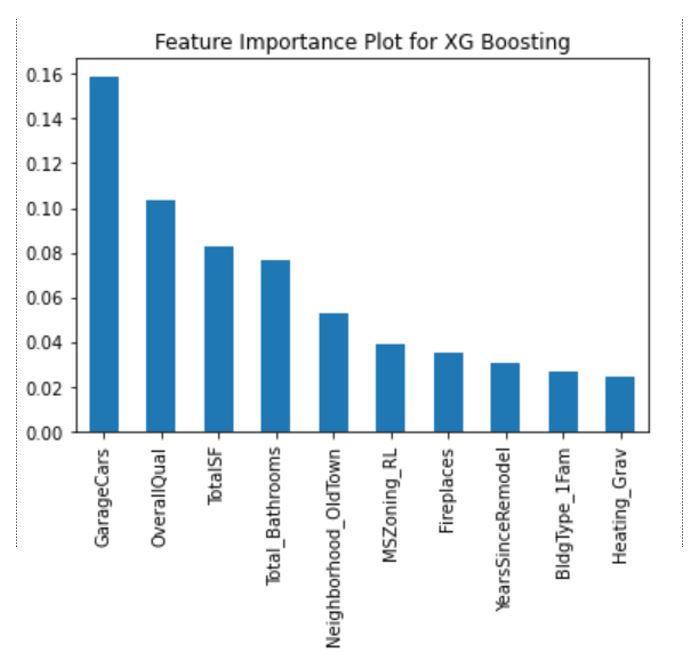
In realistic way, it's completely understood and accepted that Car Space in Garage, Overall Quality of the house, Total Square foot, total bathroom numbers, remodeled, neighborhood convenient to business places, advantage of railway commute - all features are significant in a house sale price for a homeowner which have been predicted by XGBoost efficiently with highest speed. Comparing the selected model behavior and performances , XGBoost is chosen as the best model for training the data set because of :
- Lowest RMSE
- Highest R-square
- Best computation speed
- Realistic Feature Importance
Prediction
XGBoost Model applied to test data for sale price prediction of 1459 houses and accordingly predicted sale prices are listed. Here is a snap-shot.
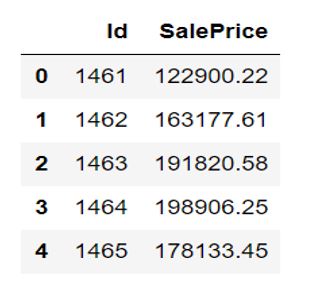
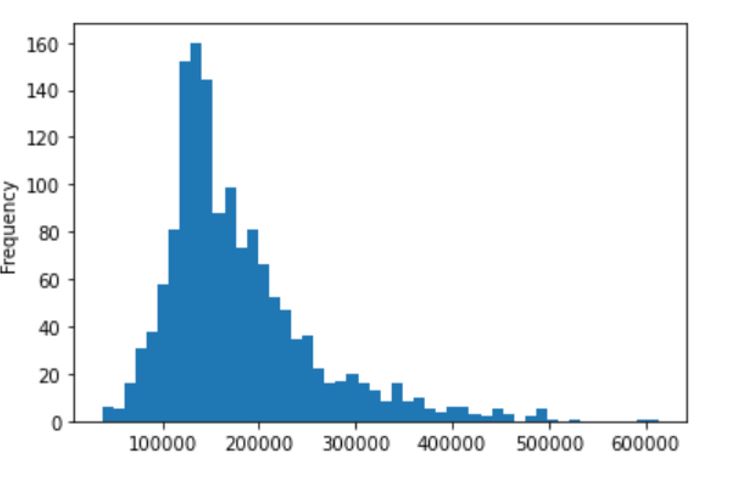
The histogram of predicted sale price of the 1459 houses can clearly show how effectively the prediction is done. Average predicted sale price is $178653.35, which maintains the same trend of train data.
Conclusion - In summary, it's aimed at answering two main questions in this project: will the tree-based model outperform the regularized linear model on predicting house price of the given dataset? The result shows though Ridge accuracy is good but the significant house features are not satisfactory like XGBoost model. The XGBoost model has the potential to perfectly predict the sale price having most important features identified for a homeowners to look to add value of their homes.
For Python Code Reference, please click here .
The skills I demoed here can be learned through taking Data Science with Machine Learning bootcamp with NYC Data Science Academy.