Reddit Controversy Sentiment Analysis
The skills the authors demonstrated here can be learned through taking Data Science with Machine Learning bootcamp with NYC Data Science Academy.
GitHub RShiny
Who cares about controversies on Reddit?
The benefit of controversies is that they challenge both the actors and the witnesses to clarify their values. The two hot-topic US controversies highlighted by national news this month---Dave Chappelle's 'The Closer' and Joe Manchin's rejection of climate action policy in Congress---have been no exception.
Though many US citizens have been unaware of or silent about these topics, a substantial force have been speaking out. People have been voicing their values in the form of street protests, legal actions, dissent in work spaces, phonebanking to voters in relevant states, and social media organizing. Others have written blogs, news articles, and lengthy social media posts. Still others have voiced their opinions via online platforms for civil (and often uncivil) discourse. One such platform is Reddit, which Wikipedia calls "an American social news aggregation and discussion website."
This project sought to use sentiment analysis via natural language processing (NLP) to explore:
How do Reddit users feel about the selected controversies?
Do Reddit users generally care more about one than the other?
This query was answered in the form of an RShiny app, a prototype of a tool that could provide stakeholders with interactive insight regarding how text creators (here Reddit users) feel about a given topic.
Stakeholders of this study include: anyone invested in public opinion of policies passed by Congress; anyone who would like to know public opinion of Netflix and its products; Joe Manchin and Dave Chappelle, whose reputations are discussed on this platform; US citizens who are curious to know how their opinion is reflected by Reddit users collectively.
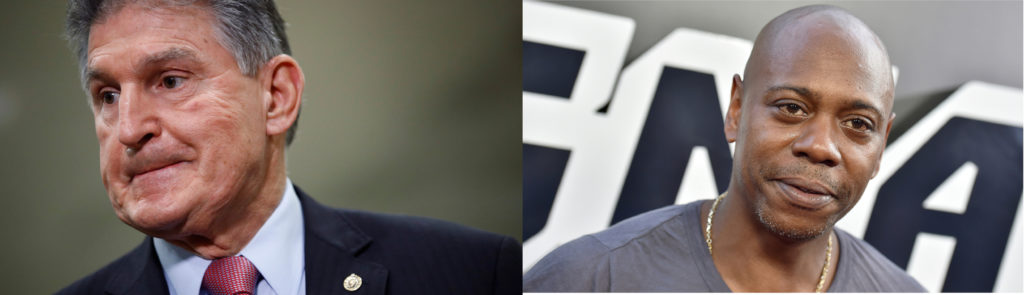
The Controversies
- On October 5th, 2021, Netflix premiered Dave Chapelle 's hour-long stand-up comedy show special 'The Closer' (2021) on it's streaming platform. Within a few days of it's release, viewers of all backgrounds became vocally critical of the harmful jokes Chappelle made in the show, jokes expressing anti-trans/lbtq, anti-asian, anti-semitic, pro-transphobic, pro-racist, and pro-mysogynist sentiments. In an escalating battle for Netflix to discontinue streaming Chappelle's special, Netflix employees have been protesting with political action in various forms. These include staging a walkout, leaking profitability data to Bloomberg News, and filing a federal labor charge
- Over the past month, senators in Congress have been negotiating an infrastructure bill that is a key part of POTUS Joe Biden's 'Build Back Better' agenda. One of the key actors in these negotiations has been Joe Manchin, who effectively blocked ambitious climate- and social-action policy from being passed into the House of Representatives. Manchin's private shares in coal brokerage Enersystems and receiving large donations from coal, oil, and gas corporations has called into question his motives as a public servant in Congress, making him a controversial figure.
What is sentiment analysis?
Sentiment analysis is an in-depth investigation of emotion in text. It is often used to assess public opinion of a company or its product via reviews, news, social media or other sources. A plethora of tools exist to collect the text and derive emotion-related information (in the NLP world called "valence"). These tools have a wide range of algorithmic complexity, from hand-built lexicons (dictionaries of words manually assigned sentiment-valence value) to sophisticated machine learning models that derive domain-specific word embeddings for a given niche of language; from binary measures of positive and negative valence to expanded emotional palettes that include โdisgustโ, โsurpriseโ, โfearโ, and โtrustโ.
Put simply, for sentiment analysis words are given a numerical value representing the valence (strength of emotion) they convey, then these numbers are aggregated to suggest a gestalt emotion of the text.
Text Mining and Sentiment Analysis Tools
For this study, RedditExtractoR, a simple-to-use R package designed specifically for scraping topic-specific data from Reddit, was used to collect Reddit threads (conversation boards) related to the selected controversies. RedditExtractoR gathered text data from the thread title, thread content (here called โpostโ), and comments. It also gathered relevant information about each thread and comment, such as the subreddit in which the thread was posted (topic-specific forum within Reddit), the number of upvotes or downvotes the thread or comment received, the date of posting, as well as the relationship between comments (whether a comment was a response to the comment before it).
Text items were analyzed using social-media-trained sentiment analysis tool VADER (Valence Aware Dictionary and sEntiment Reasoner). This sentiment analysis package provides a net score for positivity, negativity, neutrality, as well as a compound score that considers the interaction of the three preceding. This tool was simple to use and accounted for most slang words and expressions used exclusively in social media.
Pre-processing: Word Clouds
A popular and relatable product of NLP is the word cloud plot, where frequently used words in the analyzed text are clustered together. Font size and color is determined by the frequency of the word's appearance.
Dave Chappelle Controversy Word Cloud | Joe Manchin Controversy Word Cloud
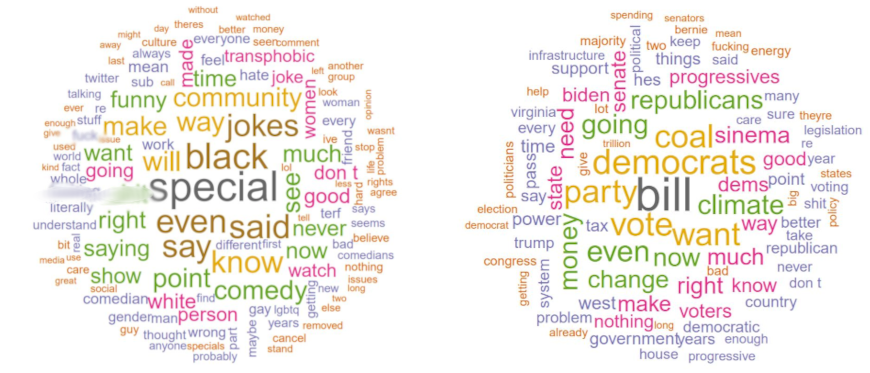
Exploratory Data Analyses (EDA)
The results of this query were sparse due to the amount of time dedicated to exploring tools for NLP and the creation of an engaging RShiny app. This prototype is a living, breathing dashboard that will be completed shortly. The plots below contrast the levels of positivity and negativity of text data relating to each controversy.
Titles texts
The histograms below suggest that a little over half of the titles for the Joe Manchin controversy showed no positive valence and some negative valence. This is in contrast with the Dave Chappelle controversy, for which over half of the titles had positive valence as well as negative.
As seen in the scatterplot, a highly neutral thread title about the Joe Manchin controversy aggregated over 200% of upvotes compared to the Dave Chappelle controversy. Conversely, the Dave Chapelle controversy had a few highly upvoted thread titles that cumulatively had much more discussion than the Joe Manchin controversy threads (as measured by the number of comments, visualized with data point size). The thread with the greatest number of comments (of the Dave Chappelle controversy) also had stronger valence, both positive (~0.31) and negative (~0.4). Further analyses is required to determine the relationship between emotional valence and text popularity
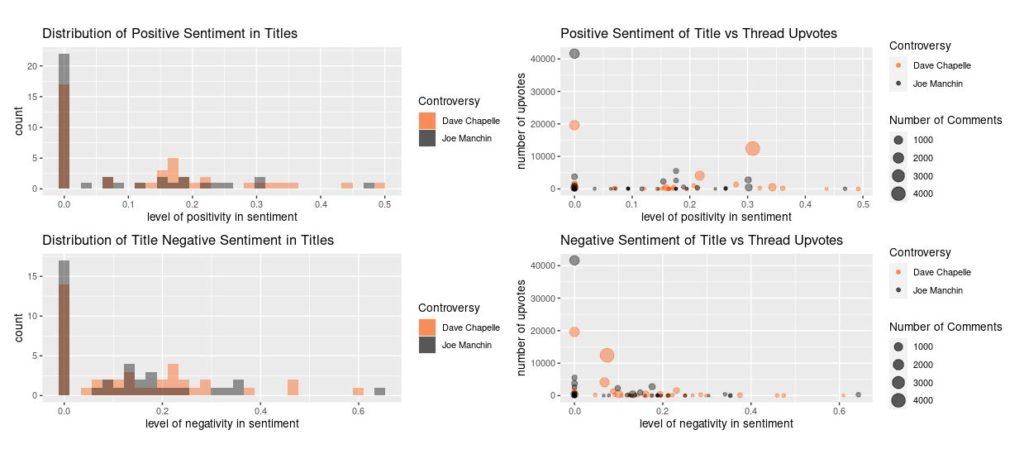
Distribution of sentiment is shown by histograms (left);
Scatter plots depict the relationship between thread popularity and title text valence (right)
The time series plots below illustrate the emotional valence of the thread titles over the past month. The lines are a product of the range of sentiment expressed on a given day. Therefore if a title or number of titles published on a given day reflect a range of positive or negative sentiment, which produces the vertical line effect.
The bottom chart suggests a slight decrease in the negative valence of titles over the last two weeks before this data was collected, for both controversies. Both charts reflect the delayed onset of titles relating to the Dave Chappelle controversy, which first appeared on October 7th compared to Joe Manchin controversy's presence since the beginning of the month.
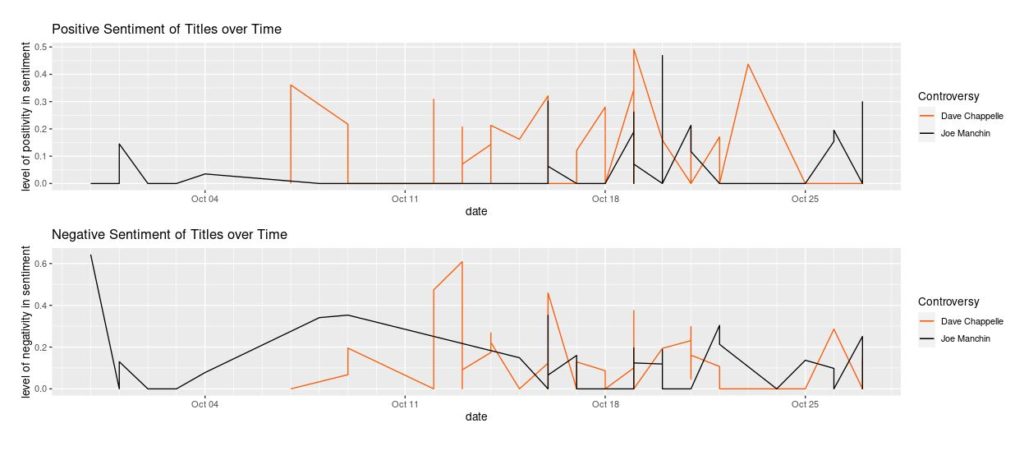
Post texts
In contrast with the title texts, post texts demonstrated much higher valence generally, both positive and negative. There were also drastically fewer post texts, which created a comically sparse time series plot (below). The reason for this is that titles are required to open a thread, whereas post text is not. However, post text is often where the author of the thread extrapolates upon their opinion regarding the subject of the thread, which explains the high levels of valence in this type of text.
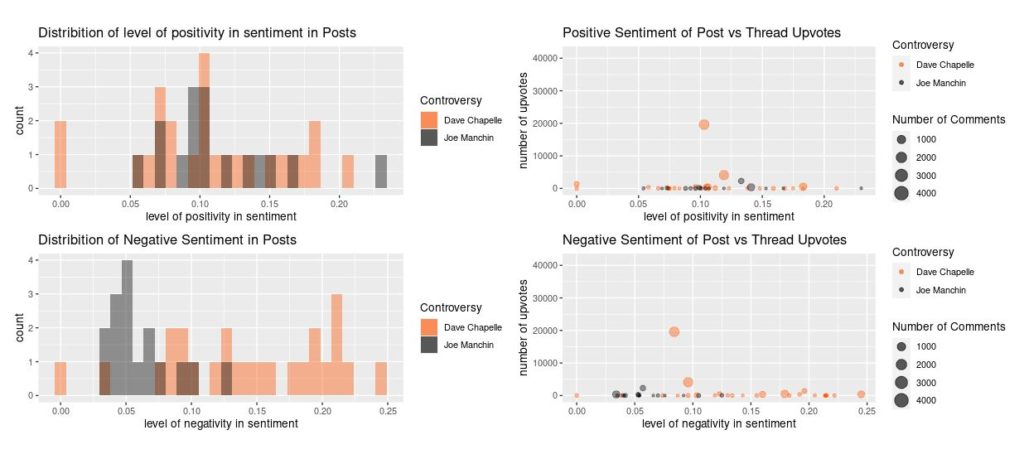
Distribution of sentiment is shown by histograms (left);
Scatter plots depict the relationship between thread popularity and post text valence (right)
Contrastingly, while posts from both the Dave Chappelle and Joe Manchin controversies showed similar distributions of positive valence, the negative valence distribution reveals a broader and more extreme negative valence for Dave Chappelle controversy posts compared to Joe Manchin posts. The time series below reflects this pattern, as well.
The scatter plots show that of the threads with post text, two Dave Chappelle controversy-related threads received significantly higher attention, as measured by both upvotes and number of comments. These popular posts both had similar ratios of positive to negative valence, a pattern that might be worth investigating in the context of other controversies.
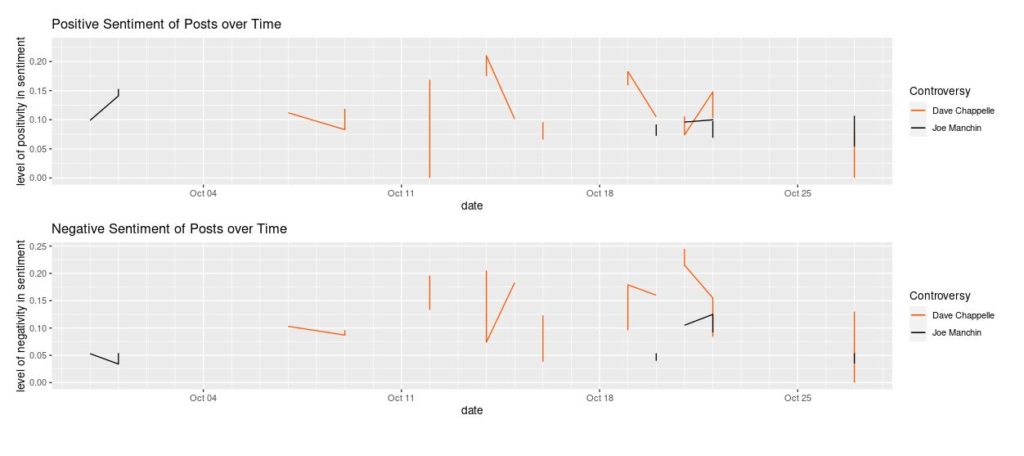
Comment texts
Comment texts are ambiguated by the many argumentative and off-topic discussions that eddy from the thread. There are distinct patterns in distribution due to sheer quantity of text. Again, text popularity and valence seem unrelated.
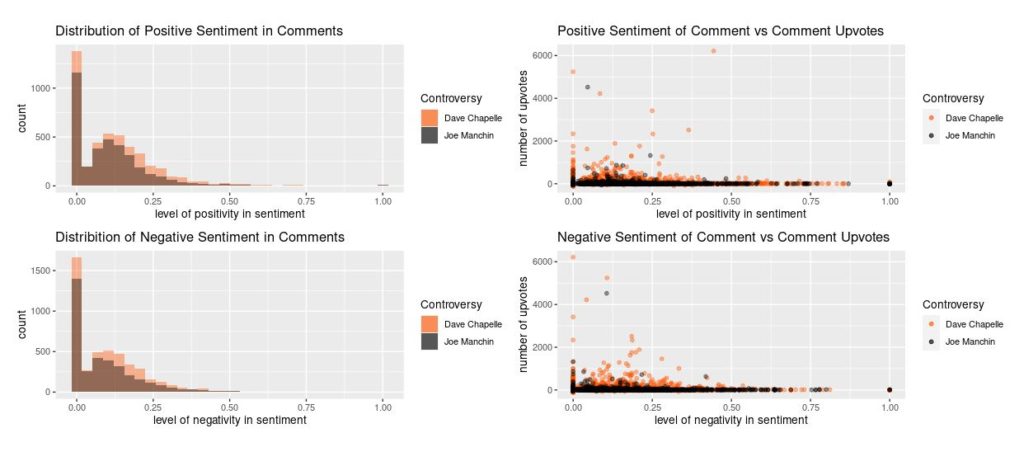
Distribution of sentiment is shown by histograms (left);
Scatter plots depict the relationship between thread popularity and comment text valence (right)
One pattern worth noting is the momentary disappearance of valence with the Joe Manchin controversy upon the beginning of the Dave Chappelle controversy. It's possible that these trends might be related (for example, if commentators of the Joe Manchin Controversy were temporarily prioritizing conversations about Dave Chappelle). However, there is not enough information to draw any conclusions.
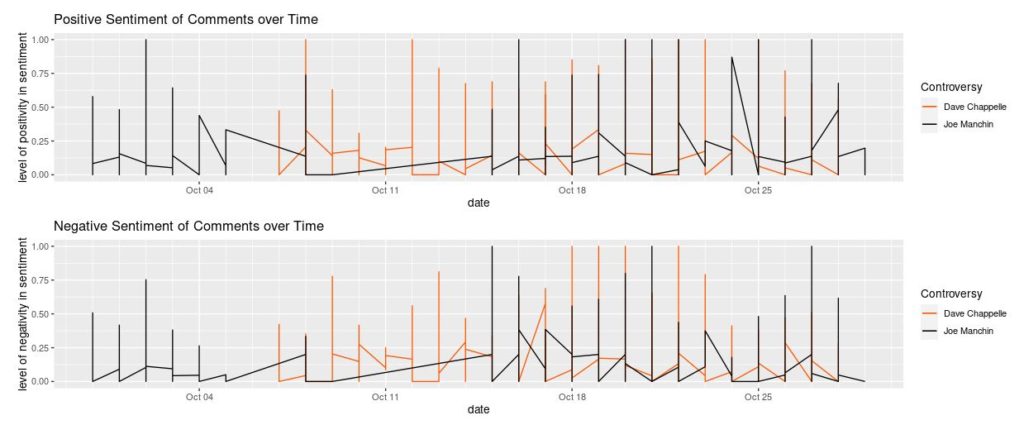
General Conclusions
Both controversies had highly comparable counts of all texts (generally the Dave Chappelle controversy had slightly more, at 59 threads and circa 4,600 comments compared to Joe Manchin controversy's 40 threads and around 3,500 comments. A few interesting patterns and phenomena were revealed, but deeper analysis is needed before any impactful conclusions can be drawn.
Future Work and Final Remarks
Due to the niche use of language within individual subreddits, sentiment evaluations in this project are subject to a potentially large margin of error.
Luckily, solutions to this issue exist. A project by William L. Hamilton, Kevin Clark, Jure Leskovec, and Dan Jurafsky , Inducing Domain-Specific Sentiment Lexicons from Unlabeled Corpora (2016) contains code that could be adapted to create unsupervised machine learning models that automatically generate and update individualized lexicon dictionaries for every subreddit scraped. This would be useful both for improving the accuracy of the data presented, as well as to open the possibility of a self-updating app that relays the progression of sentiments over time.
Additionally, these models could incorporate algorithms inspired by packages like syuzhet (among others) that currently have limited valence sensitivity but offer a broader range of emotional information (surprise, disgust, fear, anger, etc) or the ability to pull up the topics associated with high-valence language.
It should also be noted that even if we had collected all the online data available in various forms and analyzed using the most sophisticated domain-specific word-embeddings and lexicons produced by NLP machine learning algorithms to accurately hone the domain-specific sentiment values, the resulting data would still not account for the offline conversations and actions that might powerfully sway our understanding of gestalt collective opinion.
That said, this project provides a promising prototype of a tool that can be used to connect its users to the sentiments of a given text-source by providing an interactive data dashboard that visually reflects a sample of public opinion regarding a controversial subject.
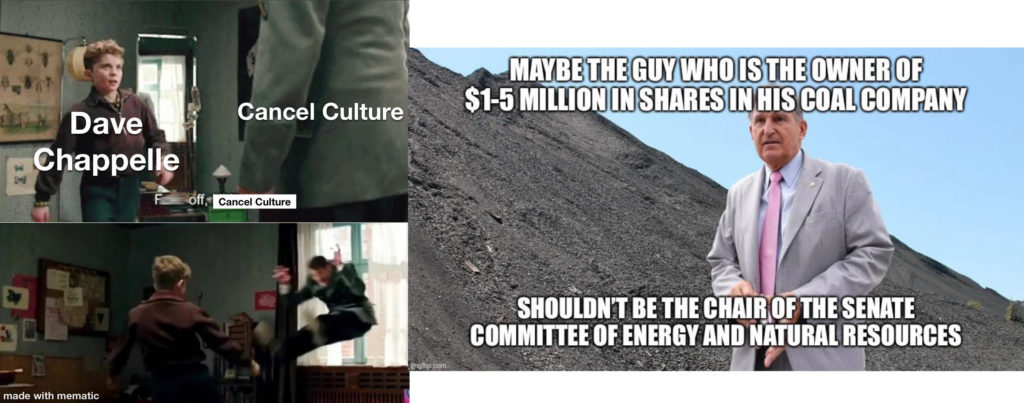
(Dave Chappelle controversy (left), Joe Manchin controversy (right)