The Shift in Data Science Jobs
The skills I demoed here can be learned through taking Data Science with Machine Learning bootcamp with NYC Data Science Academy.
Introduction
I was one of those who were genuinely convinced that the industry was shifting and becoming more data-driven. I could see the surge of data science related job postings and startups. My computer screen was constantly flooded with email newsletters and YouTube advertisements shouting about the age of data.
I was sold. I believed in that vision and I vowed to be a part of this modernization. But this was 2012. I was in the beginning of graduate school, and hell was about to break loose. I put my head down and by the time I saw light again, it was 2019. Here I am now, an aspiring data scientist, keeping my vow.
Just about a month ago, I packed my bags and joined this data science bootcamp. I still believed in the importance of a data science skill set. Throughout my graduate school career, in my isolated dungeon, I could see glimmers of data science. There would be discussions about using machine learning or AI to automate lab processes and analyze data. I was hopeful.
But now that I'm out and roaming about in broad daylight, I hear chatter about how the data market has become saturated, or the window of opportunity is rapidly closing, if not already closed. Did I miss it?
This project is a completely self-serving one. At this point, I've read and heard about all sorts of forecasts about what's next for the likes of us-- some seemed bright, some gloomy. Here, I'm taking the newly acquired skills and opportunity to see for myself what is truly out there.
Quick disclaimer and data sources
I first declare my innocence. Among the websites I scraped, LinkedIn was a "victim". Apparently, it's against the user terms that one signs off when becoming a member (yup, I have no recollection of that). I, unknowingly, scraped it until I got a suspension notice. I just wanted to collect data on the skill set of currently hired data scientists, the average time per job appointment, and education level.
I'll still be presenting the data I found here as this is in no way violating anybody's privacy. (To be clear, it's legal to scrape any public data, specifically LinkedIn's, as ruled by a court decision in Sept 2019 [1]). But for those of you who are inspired to do the same thing, be warned: doing so incurs the risk of getting your account banned.
The other websites I scraped include: Glassdoor (www.glassdoor.com/index.htm, for job postings and salaries), Levels (www.level.fyi, for compensation info for FANG companies), and Angel List (www.angel.co, for startup info). The code used for web scraping and data processing is in:
https://github.com/auscheng/MyWebScrapingProject_JobsLandscape
The Salary Perspective
One of the questions I've had for a while is: startups or non-startups, which pays more?
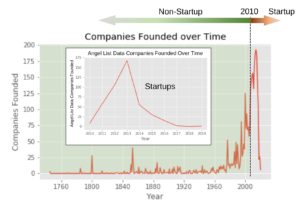
Scheme for categorizing companies as startup vs non-startup. 2010 is chosen as the year that separates the two. It's interesting to note the plummet in companies founded after 2013. The discussion for this is beyond the scope of this work.
The definition of a startup is hazy. In this study, I wanted to compare startups and non-startups from a salary perspective. To do so, I decided to use companies listed in Angel List as startups and to use all companies founded before 2010 (from Glassdoor and Levels) as "non-startups". The latter requirement is based on the fact that Angel List only started tracking startups in 2010. The decision to use 2010 as the threshold is quite arbitrary but also a quick and easy one that will make do. In this scheme, companies like Uber and Facebook are considered as non-startups.
For a while, I along with many others have always misconstrued startups as small young companies. To demystify this perception, I extracted the sizes of startups and non-startups and below are pie charts that show them:
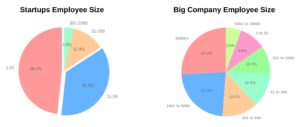
On the left is a chart of relative proportions of startup sizes and on the right is for the non-startups.
It is quite clear that both startups and non-startups feature small and large sizes. There certainly are startups that are large. This is just a caveat for kicks. What I am more interested in is if there is a correlation between company size and salary for data-related jobs. Take a look below:
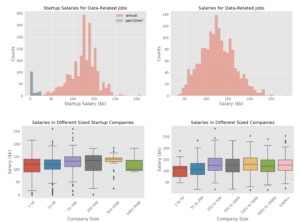
Two row shows the salary distribution for startups (left) and non-startups (right). The bottom row breaks down the salary distribution to different company sizes.
The median of the salaries for data-related jobs (data analysts, data scientists, data engineers etc.) are around $120k as often advertised. There is not much statistical significance among the differences between salaries of different sized companies. The peak on the left for startups (in dark gray) is likely part-time/weekly salaries. There are definitely cases where startups pay no salary but instead compensate with equity. However, the equity overall is disappointingly small:
Unless you really believe in your startup becoming a unicorn (which is an insanely small chance even if you think the product is ground breaking), it may wiser to stick to the more consistent market price for a data scientist.
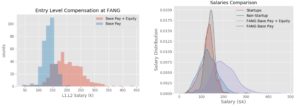
Compensation of FANG companies (left) and salaries comparison of startups, non-startups and FANG companies (right).
FANG companies here refer to Facebook, Amazon, Apple, Google and Microsoft (I know, it's not the right acronym). The base pay of these companies also follows the market price of $120k, but these employees get rewarded with equities that push their salaries off the charts to almost $200k! It's no wonder FANG has become the dream job for young grads. The salary comparison really shows the difference between these companies (One major flaw here is that I don't have any info on equities for companies outside of FANG, but I don't expect their equities to be valued greater than those from FANG).
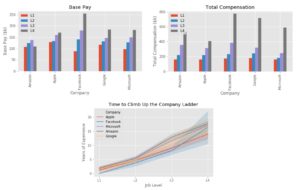
FANG salaries and years of experience required for the different levels. The top left shows the base pay, the top right shows the base pay and equity combined. The bottom row shows the years required to reach the different job levels. Note that each company has different types of job levels but they are mapped to a standardized job level so that comparison can be made across the companies.
Looking more into FANG, we see that loyal employees definitely get rewarded handsomely. In 5 years, on average an employee will be earning roughly $200k and in 15 years, over half a million. Crazy. In contrast to the idea of loyal employees, look at the behavior of employees around the US and you will be quite appalled.
Ignoring the large counts near 0 years as they are likely due to short temporary positions or summer jobs, the average time per job appointment is below two years, which is a lot shorter than the average duration of about 4 years for all jobs in the US [2]. The high frequency in job change can be particular to data-jobs but can also be because the people sampled are mostly millennials who have a reputation for job hopping and are costing the US about $30 billion dollars annually [3].
The most popular geographic regions for data jobs are, unsurprisingly, San Francisco and New York. Silicon Valley here includes all the major suburbs around San Francisco such as Palo Alto, Cupertino, Redwood City and so on. New York overtakes San Francisco for non-startup job postings. Boston, where I was most recently based, is also high on the list and this can be explained by the dominant biotechnology and pharmaceuticals. The big academic setting is definitely also a big engine for startups. The sad news is that the cities I mentioned aren't exactly the friendliest.
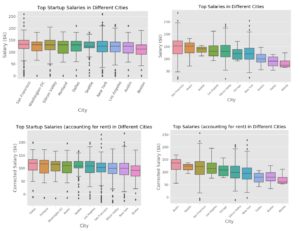
Salary distribution for different locations. Top row shows the raw salaries. Bottom row shows the salary minus the median one-person rent for the respective city.
California and New York definitely win in terms of raw salaries but their respective high living expenses put them to lower spots. New York suffers and gets categorized to the lower tier. The same goes for Boston. Texas cities are an unexpected bonus, benefiting from a competitive market salary of data jobs as well as much lower living cost.
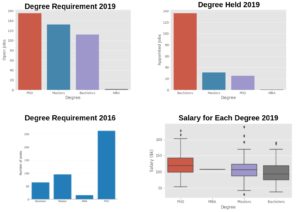
The left column shows the degree requirement posted by open jobs in the respective years. The top right show the degree employees actually have in the data industry. The bottom right shows the salaries for the different degrees according to the open job posts. Graph from 2016 is taken from an old blog post from NYC Data Science Academy [4].
The Education Perspective
A comparison between the number of PhD's hired in 2016 and 2019 suggests that industry is adjusting to the idea that a PhD is not essential to data science. Or rather, it could be that data science is now perceived as more of a skill that can be acquired and mastered through practice instead of purely through educational degrees.
From my observation and experience so far, a PhD, for the most part, is definitely unessential to understanding and executing data science. Looking at the salaries for the different degrees has been a reality check for me: the competitive advantage of having a PhD over other degrees is marginal at best. But, as a proud PhD candidate myself who had survived extremely grueling work hours and acutely demoralizing work, I do think PhDs offer very valuable intangibles.
For the most part, however, skill is what matters. To accentuate the point, data science jobs are overwhelmingly dominated by bachelors holders. This goes to say that as long as you can prove your ability, with or without an advanced degree, pedigree doesn't matter.
I do want to point out that various levels of data science jobs exist. Here I am simply talking about entry to intermediate levels of data science. An examination of high-level data science jobs will surely yield different results.
The Language Perspective
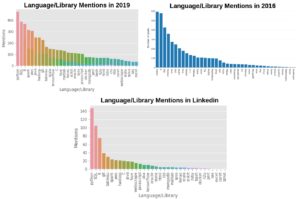
Top row shows the mentions (popularity implied) of the coding languages or libraries in the open job posts. The left corresponds to the year 2019 and the right is for the year 2016. This top right graph is taken from a previous cohort blog post [4]. The bottom graph shows the number of mentions based on the resume of currently hired data scientists.
Finally, I'd like to look at how the popularity of different languages or libraries have changed over the years and if there is any discrepancy between what companies want and have. Python remains a strong candidate. The most notable change here is that SQL has overtaken R from 2016 to 2019. We also see that SQL seems to be much more highly valued than R based on the number of mentions in LinkedIn. Brush up on your SQL!
Bonus section: mention of buzz words in job descriptions. Take a look and guess what the next big thing is!
My Conclusion?
The money is good, education doesn't matter. Just prep and apply.
References
[1] https://www.forbes.com/sites/emmawoollacott/2019/09/10/linkedin-data-scraping-ruled-legal/#2155e18a1b54
[2] https://www.thebalancecareers.com/how-long-should-an-employee-stay-at-a-job-2059796
[3] https://www.gallup.com/workplace/231587/millennials-job-hopping-generation.aspx
[4] https://nycdatascience.edu/blog/student-works/web-scraping/glassdoor-web-scraping/