Building a Titanic Classifier with End-to-End Machine Learning Pipeline
In this blog post, I’ll guide you through the creation of an end-to-end machine learning pipeline using the Titanic dataset. This project was developed as part of a technical interview, focusing on data exploration, preprocessing, model selection, evaluation, and deployment. Additionally, the project ideation includes a fully automated model training and selection pipeline that streamlines the processes of model optimization, training, and comparison. The aim was to build a scalable and reusable pipeline that could be easily deployed using Docker.
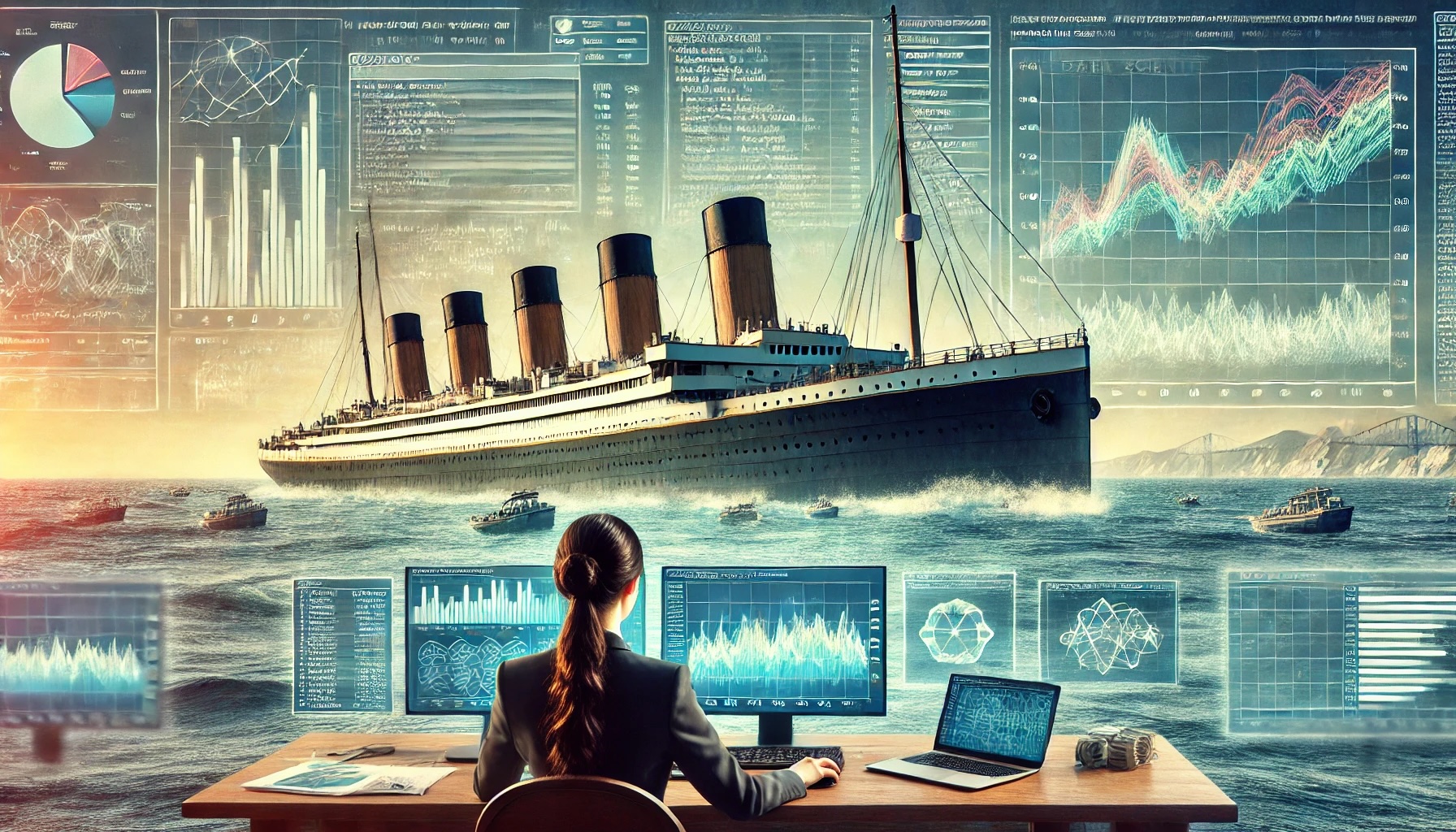
Image 1: A blend of the past and the present: The Titanic sails through calm waters, while modern data visualizations and code surround a dedicated data scientist, seamlessly merging historical elegance with the power of today's analytical technology. This image was generated using DALL·E.
Dataset Overview
The Titanic dataset is a well-known benchmark for binary classification problems. It includes information about passengers on the Titanic and their survival status, with the objective of predicting whether a passenger survived based on the following features:
- PassengerId: Unique ID for each passenger
- Survived: Whether the passenger survived (0 = No, 1 = Yes)
- Pclass: Passenger class (1st, 2nd, or 3rd)
- Name, Sex, Age: Demographic information
- SibSp, Parch: Number of siblings/spouses and parents/children aboard
- Ticket, Fare: Ticket number and fare paid
- Cabin, Embarked: Cabin number and port of embarkation
The dataset contains 891 rows and 12 columns, and is slightly imbalanced, with 60% non-survivors and 40% survivors.
Exploratory Data Analysis (EDA)
Missing Value Imputation
- Age: About 20% of the data for "Age" were missing. To impute these, I used the median age based on the passenger class. This way, passengers in the same class with similar conditions had comparable age values.
- Cabin: 77% of the "Cabin" values were missing. Given the large number of missing values, I imputed these with "U" for unknown and later used this information in feature engineering.
- Embarked: Only <1% for "Embarked" were missing, which I filled using the mode, as it’s the most frequent port of embarkation.
Visualizations
Using histograms, bar charts, box plots, and correlation heatmaps, I analyzed the relationships between various features and survival. Some key insights:
- Pclass and "Sex" showed strong correlations with survival, with passengers in 1st class and females having higher survival rates.
- Age distribution indicated that older passengers were more likely to survive.
- Fare showed a right-skewed distribution, with higher fares generally associated with higher survival rates.
Variable Processing
Encoding Categorical Variables
For features like "Sex" and "Embarked", I applied One-Hot Encoding, converting them into binary numeric format. These features have no intrinsic order, so this method ensured that they were appropriately handled in the modeling process.
Ordinal Encoding
I used ordinal encoding for "Title" and "Deck" features since both have a natural ranking.
- For "Title" (extracted from passenger names), I mapped social rankings (e.g., Mr., Mrs., Miss) to numerical values based on their importance.
- The "Deck" feature, derived from the first letter of the Cabin number, was encoded to reflect different ship levels. Deck A was ranked higher than Deck G, which was closer to the lower decks of the ship.
To ensure robustness in the event of unseen categories during inference, I implemented a mechanism that assigns new categories to a default value of zero, preventing errors during model deployment.
Processing Continuous Variables
The skewness of continuous features like "Age" and "Fare" was assessed to check for asymmetry in their distribution.
- "Age" had a slight right-skew with a skewness value of 0.54, so I left it untransformed.
- "Fare" had a skewness of 4.79, indicating a significant right skew. To handle this, I applied a log1p transformation, which normalizes the data and reduces the impact of extreme values. This transformation was preferred over the standard log due to the presence of zero fares.
Feature Engineering
To improve model performance, I created several new features:
- FamilySize: Combined the "SibSp" and "Parch" features to capture the total number of family members aboard.
- IsAlone: A binary feature created to indicate whether a passenger was traveling alone.
- Title: Extracted from the "Name" feature to categorize passengers based on their social title.
- Deck: Derived from the "Cabin" feature to represent the deck level.
Feature Selection
I used a Random Forest classifier to perform feature selection. This method ranks features based on their importance in improving the model’s performance. After analyzing the feature importance and correlation plots, I dropped several columns, including "SibSp", "Parch" and "IsAlone" as they either had low importance and/or caused multicollinearity. This reduced overfitting and simplified the model.
Model Selection and Training Pipeline
I developed a model training and selection pipeline designed to automate key processes, including model optimization, training, and evaluation. The pipeline efficiently handles hyperparameter tuning through random search and generates comprehensive performance summaries. Additionally, the pipeline automatically saved two versions of each model: one trained only on the train set for model selection, and another trained on the full dataset for inference.
I tested multiple models—Logistic Regression, Random Forest, and XGBoost—using cross-validation to evaluate accuracy, precision, and recall. Given the dataset's slight imbalance (60% non-survivors and 40% survivors), I emphasized metrics beyond accuracy, focusing on precision and recall to capture the model's performance on the minority class (survivors). Random Forest demonstrated the best performance, achieving a cross-validation accuracy of 84.3% along with balanced precision and recall.
The dataset was split into 70% training data and 30% test data for robust model evaluation. Model selection was based solely on performance metrics from the train set, and only the selected Random Forest model was evaluated on the test set to prevent data leakage and ensure unbiased evaluation.
Evaluation
The final Random Forest model was evaluated on the test set to assess generalization. Given the slight class imbalance, I used additional metrics like precision, recall, and F1-score to evaluate the model’s performance on the minority class (survivors). I also plotted the ROC AUC curve to assess model performance across different thresholds. Using Youden's J statistic, I determined the best threshold to balance false positives and true positives. This threshold was saved and will be used during inference to ensure consistency.
Production Pipeline and Docker Integration
The entire production pipeline was designed to automate data preprocessing, model training, and inference. Key components include:
- Data Preprocessing Scripts: These scripts handle feature transformations, imputations, and clean data preparation for the model.
- Configuration: Hardcoded values such as the best threshold, model paths, and final model features are stored in the config folder for easy access during execution.
- Model and Ideation Code: The optimized models and notebooks containing exploratory analysis and inference logic are saved in separate directories for easy reference.
- Unit Testing: The tests folder includes unit tests for the pipeline’s key functions. These tests ensure the pipeline operates as expected at every stage.
- Shell Script and Dockerization: The run_titanic_pipeline_and_tests.sh script automates the pipeline’s execution. The project is fully containerized using Docker, enabling seamless deployment across environments.
Project Execution
This project is fully containerized using Docker, ensuring that all dependencies and configurations are consistently managed across environments. You can initiate the Jupyter Notebook and run the pipeline and unit tests effortlessly. Docker allows for a seamless setup, ensuring that the project remains reproducible and scalable.
Conclusion
This project demonstrates the end-to-end process of building, evaluating, and deploying a machine learning model using the Titanic dataset. The integration with Docker ensures the pipeline is scalable and deployable across various environments without dependency issues. By focusing on feature engineering, robust evaluation techniques, and appropriate metric selection, the model performs effectively, even when facing a slightly imbalanced dataset.
For more details, explore the complete project and production pipeline in the GitHub repository.