Data: Finding factors influencing NICU admissions
The skills I demoed here can be learned through taking Data Science with Machine Learning bootcamp with NYC Data Science Academy.
Introduction:
Every year more than 4 million families in the United States visit maternity wards bringing new children into the world. Ten percent of mothers experience complications resulting in newborns being admitted into the intensive care unit (ICU). Using annual data from the Center for Disease Control and Prevention (CDC). Our goal is to understand trends and relationships between pregnancy risk factors affecting delivery complications, congenital abnormalities, and admittance to the neonatal intensive care unit (NICU).
Using a Tableau dashboard, our analysis will show predictive models that provide insight for healthcare policy makers. This application will help systemically vulnerable populations, hospitals and primary care providers to optimize the distribution of limited resources. Through this we can identify preventative measures for patients allowing expecting families to better prepare for the challenges of pregnancy.
The Data set: Extraction and Preparation
The CDC annually publishes information for all births and pregnancy-related events for independent research and analysis. Between the years of 2014-2018 there were approximately four million births. Our team collected data covering over 200 features characterizing parents, methods of delivery, health of the newborn and admittance to the NICU or ICU for the mother which contained approximately 25GB of data.
Our data was configured into a fixed width format which required position encoding along lengths of characters. Each year had a PDF user guide detailing the file layout, encoding of features and detailed technical notes explaining data collection and imputation.
Our application tailored parses to extract each year from the original files into pandas dataframes and saved them into CSV files for manipulation. Each dataset contained approximately 20 million observations. We downsampled each to 5%, which was 200,000 observations from each year.
This factor was decided using a second order classification using mean and standard deviation which observed no statistically significant differences between distributions within numerical and categorical features. From this raw selection we observed a marginal amount (less than 3%) of observations in boolean features were missing or unknown. Where applicable, missing or unknown data for boolean features was ignored.
After extraction and removal of redundant features, the remaining 109 columns were analyzed in depth with respect to NICU admittance.
Data Analysis:
Between 2014 and 2018 the average rate of live birth admittance to the NICU was 8.65%. The graph below shows there is an increasing admittance to the NICU over time. While some of this increase may be due to improvements in record keeping reducing the number of cases labeled unknown, this is a worrying statistic for families due to the emotional, physical and financial cost involved.
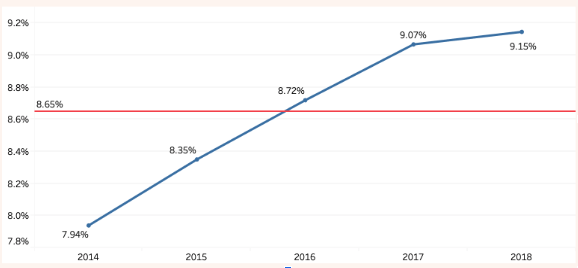
โFigure 1. Yearly NICU Admittance
With this in mind, the remaining features have been divided to consider factors occurring during pregnancy, during delivery, and factors measured after delivery. Each group of features is examined first for relevance to NICU admittance, and then the factors from the first group are re-examined for ties to the most relevant factors in the latter two groups.
Figure 2 shows lower admittance to the NICU of women giving birth aged 20-29, while women over the age of 40 have high admittance. The time series indicates that mothers in their 30s are increasing, while the number of teenage mothers is decreasing. This trend suggests that the rising rate of NICU admittances is likely to continue. Additionally observe that the number of mothers in the highest risk group, those over 40, is two orders of magnitude fewer than the lowest risk group, mothers in their 20s.
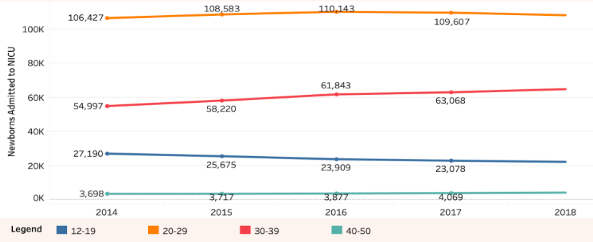
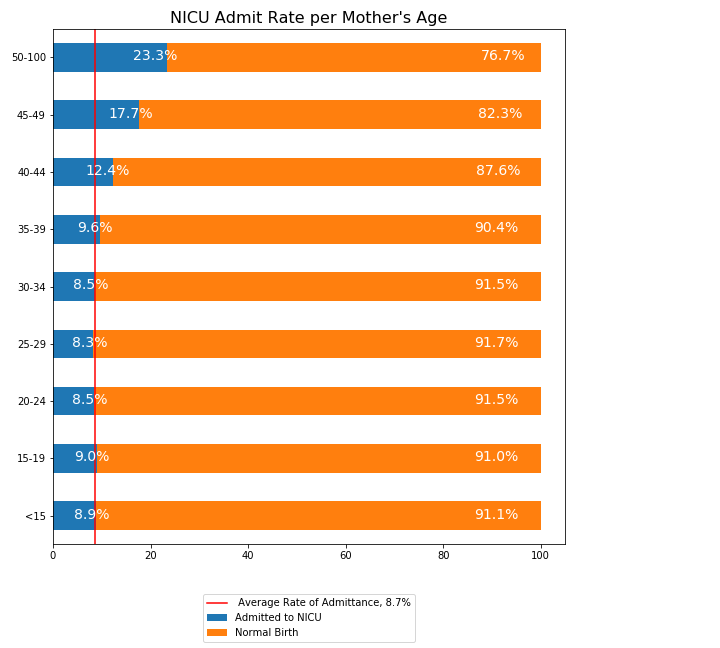
โโFigure 2b: NICU Admittance per Mother's Age
Body Mass Index Data
Following age, the next feature examined which broadly relates to overall health is the body mass index (BMI), calculated using height and weight. BMI is often used as a baseline indicator for long term health risks. Being within the โnormalโ BMI range has a slight protective effect against NICU admission, while any level of obesity is associated with an up to 3.4 % increase, as shown in Figure 3b. It is worth noting that being under or over the ideal range had no significant effect, and no data relating to whether dietary or nutritional needs are met is collected by the CDC.
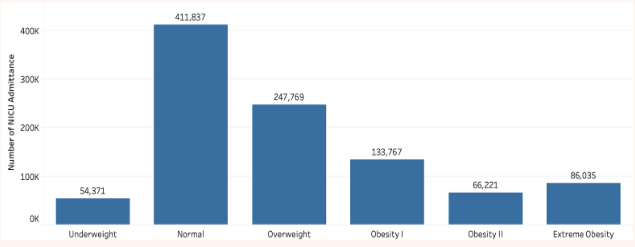
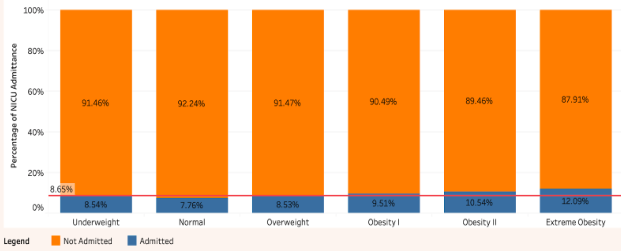
In figure 4 below, observe that there does not appear to be a great risk posed by smoking before becoming pregnant. However, smoking during the first two trimesters of pregnancy slightly increases the risk of admittance to the NICU to 9.78%, and smoking during the third trimester further increases the risk to 15.61%. While getting smokers to stop smoking in general is a desirable community healthcare goal, it appears providing alternatives to cigarettes to women as they enter their third trimester is of particular importance.
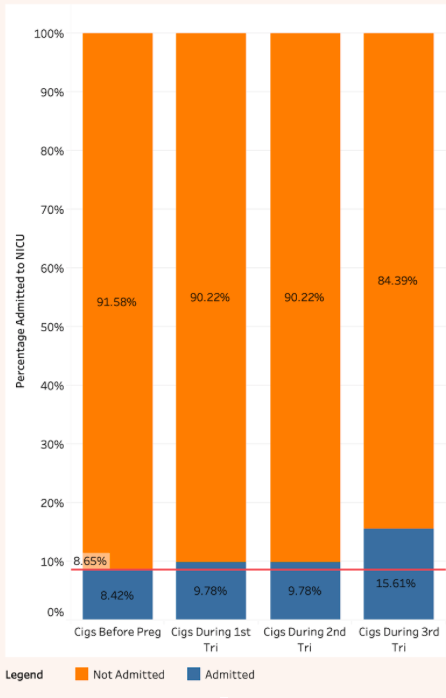
โโ4 : Smoking Risk by Trimester
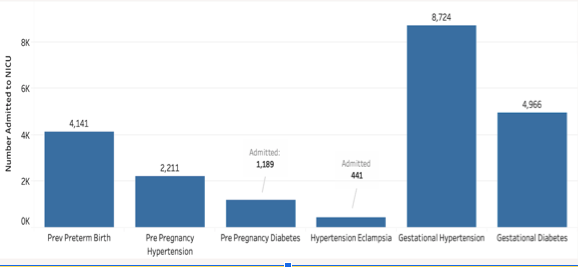
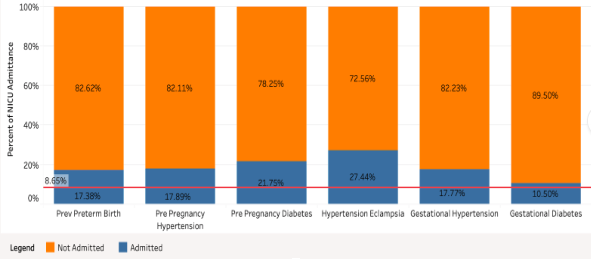
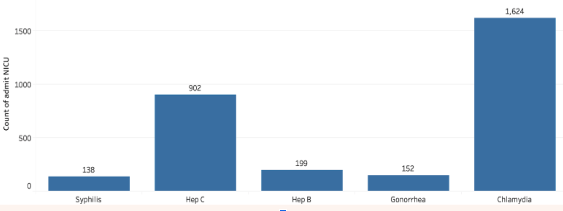
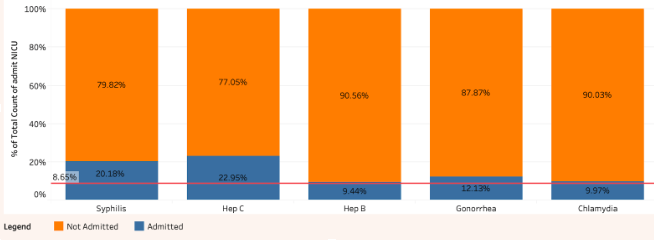
โ6b : Percentage of Motherโs Medical History Infections
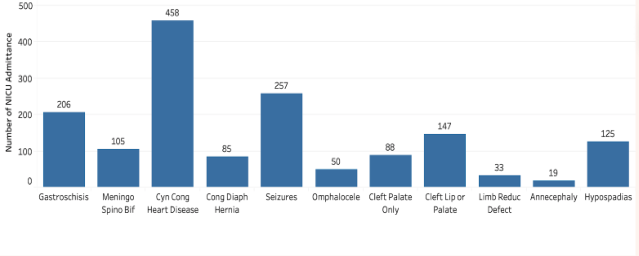
Figure 7b : Count of Infant Congenital Factors
Pre-Existing Conditions Data
We compared over thirty pre existing conditions and they mostly remained constant each year showing little trends increasing or decreasing. One feature we saw increasing was multiple births which has been going down over time. Our goal is to find which features contributed most to an infant being admitted to the NICU. We also want to create a model that could help predict admittance. For this we decided to use two basic classification models to test performance.
Regression:
Having developed some intuition from our graphical analysis we were able to identify some features that were more predictive of NICU admittance. We wanted to validate these assumptions of feature importance and classification using a machine learning model.
As an initial simple predictor, we trained a Logistic Regression model to predict admittance to the NICU. Since Logistic Regression can only classify between two classes, we used the one-vs-all method to create three sub-models, one for each of the possible outcomes(โYesโ, โNoโ, โUnknownโ), that predicts the probability that a given baby will fall into each category(eg โYesโ vs. โNot Yesโ, โNoโ vs. โNot Noโ). After calculating three probabilities with these models, the model uses outcomes with the highest probability to select the features it has found to be most important
Mother Data
However, the results of this model proved not very predictive or reproducible. In Table 1, the results are reported in a matrix showing the percentage of predictions against the actual admittance data. The sensitivity, or ability of the model to predict NICU admittance in cases where a baby was indeed admitted to the NICU, of the resulting model is about 20%. This indicated that the model heavily favored predicting the majority class โNoโ regardless of the underlying data.
Repeated attempts to test the model on different test subsets of the data yielded inconsistent feature rankings. This instability in results indicated that the relationships between our dependent and independent features were likely not linear and reinforced the suspicion that the model was biased towards the majority class.
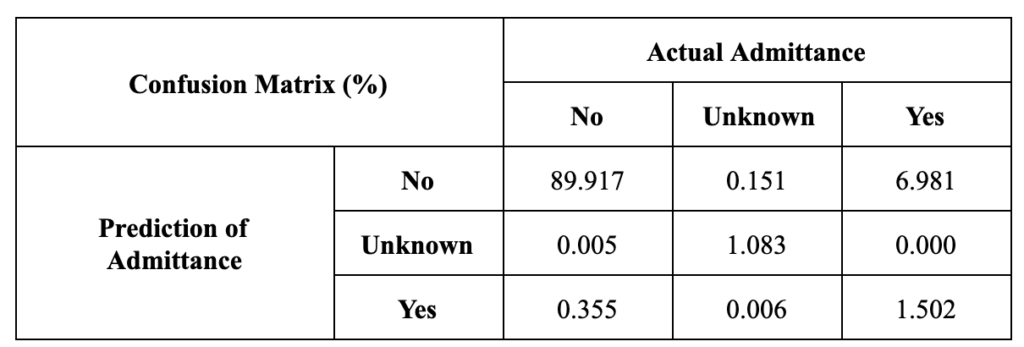
Random Forest:
Our regression model proved to have volatile results and we wanted to try a model that was more robust. We used a random forest classifier because we found the data did not have a linear trend. We initially performed the random forest classifier on a subset of our down-sampled data frame. This was necessary due to the large size and high cost of a random forest.
Our results provided a model with 92% accuracy but after looking at the confusion matrix, we found our model was overfit and only chose the option not to admit to the NICU. We decided we wanted to create a new down-sampled data frame that had equal amounts of observations admitted to the NICU and not. After training this model we found it had 84% accuracy but our modelโs sensitivity was correctly predicting 80% of cases that should be admitted to the NICU.
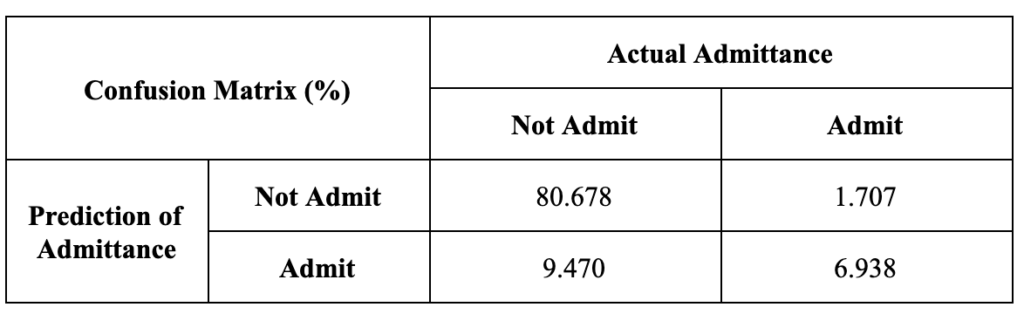
Our top features selected by the model correspond with hospital standard practice of admission due to low birth weight, preterm and late-term birth and serious health conditions. This model proved to be more robust and fairly accurate given the complexity of features.
Conclusion:
Our analysis and modeling suggest that 10 features are likely targets for high NICU admittance. BMI and smoking while pregnant are 2 conditions that highly contribute to NICU admittance that can be addressed before and during pregnancy. Women over 40 have over twice as much risk of being having issues and women 20-30 years of age have the least risk.
The random forest model had an 80% sensitivity for predicting a child's risk based on the mothers health conditions of admittance. Our tableau application neatly displays conditions that should be considered higher risk that might contribute to the child being admitted to the NICU.