NLP Recipe Search Engine
The skills the authors demonstrated here can be learned through taking Data Science with Machine Learning bootcamp with NYC Data Science Academy.
Introduction
According to the USDA, the average US family of four will waste $1,500 of food every year. This is important because the median family in the US has an income of $79,900, according to the Department of Housing and Urban Development. This means that for the median family, nearly 2% of their income every year is lost to food waste. M
uch food is wasted because people donโt know how to use up what they have when planning their meals. They also often revert to the recipes they already know and repeat the same meals multiple a times a week until they grow bored and lose motivation to cook. While there is no shortage of recipes online, it can be difficult to find the one that draws on what you have on hand.
To address that problem we wanted to make a recipe recommendation engine that allows users to input the ingredients they want to use and be given a selection of recipes. Our goal with this project was to create a recipe recommendation engine that would be able to take in a list of ingredients and output a list of recipes that used the ingredients. This would allow people to keep things new in the kitchen, as well as use their ingredients before they spoil.
Exploratory Data Analysis
The dataset contains over 2 million observations of recipe data downloaded from RecipeNLG, originally downloaded from various web resources and web-scraped. It includes six columns: the title of the recipe, the ingredients needed, the directions for making the recipe, the link to the recipe, the source of the recipe (whether it was downloaded or web scraped), and the Named Entity Recognizer (NER) of the ingredients list without stop-words and lemmatized.
The distribution of the number of ingredients shows a somewhat normal distribution with the peak around seven ingredients. It also exhibits a slight right skew, with some recipes containing 20 or more ingredients.
The distribution of the number of lines of direction has a peak at five lines of directions and is also right skewed. This is not surprising because the directions can have many lines and some do, but they must all have at least one line of directions, which is the natural floor cutoff.
When comparing the number of ingredients with the number of lines of directions, their previous distribution is seen without any spots of anomalies. In this heat map, the darker shading represents areas that show up more frequently. The darkest area of the heat map shows that most recipes have six to eight ingredients and four to six lines of directions.
After completing the initial EDA, it was necessary to perform certain data cleaning. This cleaning included removing errors in the dataset-- more specifically the directions column โ removing stop words, punctuations, and lemmatizing words. The main error found in the directions column was related to a Unicode error which caused the degree symbol, ยฐ, to print as โu00b0โ.
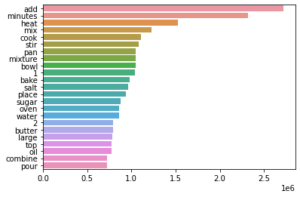
Most common non-stop words
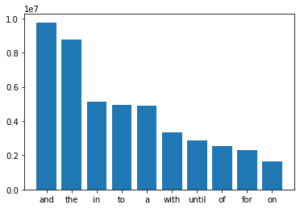
Most common stop words
In order to analyze the most frequent word combinations, bi-grams and tri-grams were used and plotted. The top bi-grams consisted mainly of stop words, while tri-grams were more related to oven utilization, time management, and food seasoning.
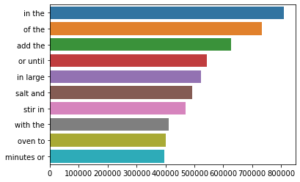
Most common bi-grams
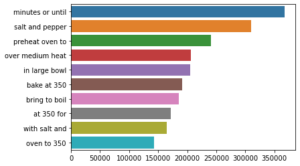
Most common tri-grams
Recipes in the dataset corresponded to different food types, cooking methods, and preparation. Word clouds were generated to observe the most common words in each of the datasetโs columns, title, ingredients, and directions. The larger the word in the word cloud, the more frequent the word appears in their respective column.
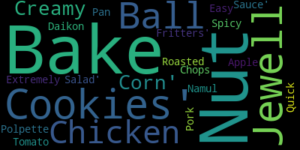
Word cloud of title feature
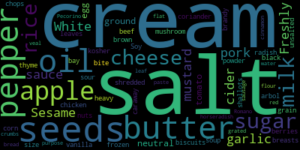
Cloud of ingredients feature
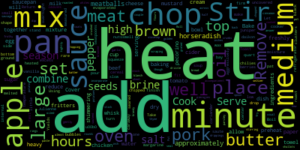
Word cloud of directions feature
NLP Models
Following EDA and data cleaning, model selection and building were required. Regarding model selection, two NLP models were chosen, Top2Vec and Gensim (LDA). Top2Vec is known for detecting topics in text data and generating embedded topic, document, and word vectors. Its advantages for this project are the automatic number of topics chosen and the built-in search engine Top2Vec offers. In terms of its disadvantages, Top2Vec results in many outliers and could result in too many topics.
Latent Dirichlet Allocation (LDA) - Gensim
Gensim, or Latent Dirichlet Allocation, is a generative statistical model that explains a set of observations through unobserved groups in which each group explains why some parts of the data are similar. LDA, compared to other NLP models, is easier to interpret due to the lower number of topics and one document can contain several different topics. Regrettably, LDA could easily produce overlapping and/or incoherent topics.
To begin modeling, a sample of 10,000 entries of the cleaned directions column was used. Once the initial LDA model was created, a default output of 20 topics was outputted. The visualizations built from the LDA model are shown below. The intertopic distance map plots the 20 different topics created by the model.
As observed, clusters can be seen with a certain overlap between topics. The top 30 most salient terms are the most informative words concerning a specific topic. The higher the saliency score, the more valuable it is for identifying particular topics. Each wordโs corresponding bar refers to the frequency in the sample set.
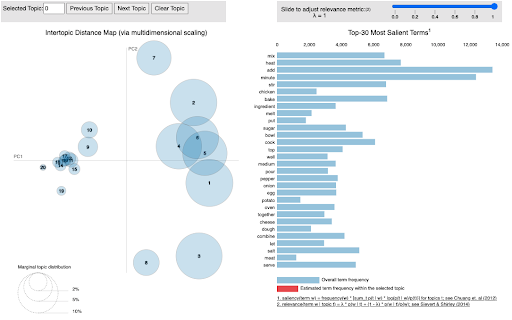
Intertopic distance map and salient terms
While the initial LDA model provided some clarity on the sample set, it was necessary to optimize the LDA model to search for the best number of topics. The coherence plot is a line plot that contains the number of topics on the X-axis and the modelโs coherence score on the Y-axis. Coherence measures the score topics by measuring the semantic similarity between high-scoring words.
To find the optimal number of topics, we set out the number of topics on the X-axis range from 0 to 100. The coherence plot represents a graph with the number of topics and their respective coherence scores. The optimal model chosen was eight topics due to the drop-off of coherence after 8.
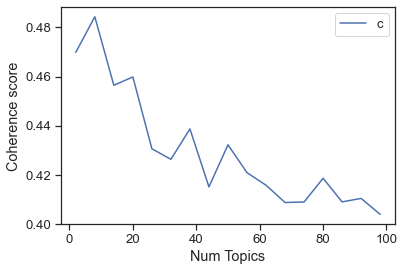
Coherence score plot
Once the optimal model was chosen, a new intertopic distance map and salient terms were generated. New salient terms were defined and a clear segmentation of topics can be observed.
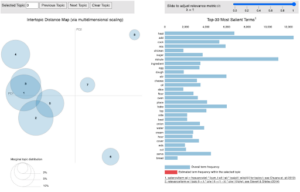
New intertopic distance map based on eight topics and salient terms
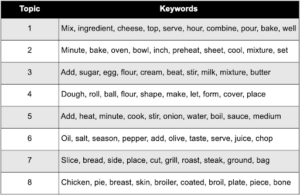
Top keywords corresponding to each topic
Regarding the eight topics outputted by the model, the keywords table demonstrates keywords associated with each topic, helping describe and categorize topics.
A benefit of using LDA is the modelโs ability to assign each recipe in our sample dataset to a certain topic. Document_No refers to a specific recipe entry, Dominant_Topic refers to the topic the model assigned the recipe to, and the Topic_Perc_Contrib shows how correlated or close the recipe is to the topic, based on a value from 0 to 100.
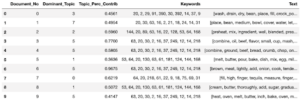
Table of individual recipe topics
Top2Vec
As with the LDA model, we used the same 10,000 samples from our data set to create a model using Top2Vec, another unsupervised learning method. Top2Vec takes the texts and turns them into vectors, which it then clusters by similarities, then assigns topics to the clusters. There were two main reasons for us to use Top2Vec. The first is that Top2Vec automatically determines the number of topics, which in our case turned out to be 96. The other is that Top2Vec has a built-in search function, which is a great feature for creating a user interface that could present search and recommendation results.
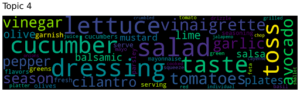
Word cloud of most salient terms in Topic 4
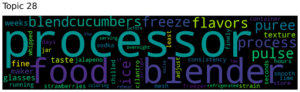
Word cloud of most salient terms in Topic 28
Once the topic vectors are created, document and word vectors are created, with the word vectors being compared to the topic vectors using cosine similarity score. For this scoring, words and documents are converted into vectors, and their similarities are compared. The maximum cosine similarity score is 1, meaning that the vectors are exactly the same and have the highest similarity, and cosine scores of 0 mean that they are unrelated. A cosine similarity of 0.5 or higher is considered to show strong similarity. These topics can then be used to create word clouds so that the topics themselves can be visualized.
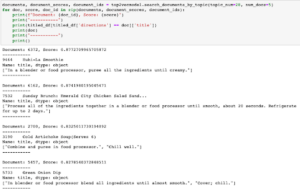
Topic 4 is a word cloud based around salads
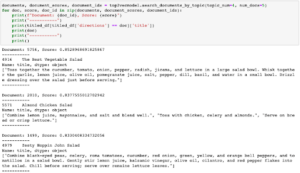
Topic 28 is for smoothies and blended foods
Examples of Top2Vecโs search function in which the recipes returned are most closely associated with the topic.
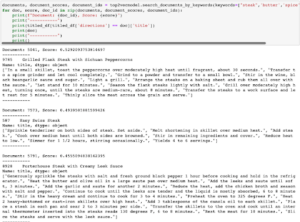
Searching our Top2Vec model using a list of ingredients
As mentioned previously, one of the main appeals of using Top2Vec was the ease of searching. This allowed us to build a basic search interface by using Dash in order to allow an end user to search the model we built.
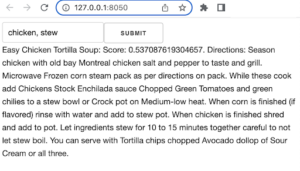
Dash app looking for the highest score by searching through recipes that include both chicken and stew
Conclusion
In conclusion, unsupervised learning used for NLP is effective on the dataset. Our NLP models can suggest the top five recipes based on the ingredients and underlying topics. This helps people find the recipes that they are more interested in more efficiently.
This model can be used to support a recipe search engine and can be recommended for many uses. For example, keywords can be entered and the search engine using this model in the background would populate the most relevant recipes. This model is also helpful in helping find which recipes and categories are surprisingly related.
Perhaps there is an underlying relationship between gazpachos and salads, and this model could bring that information to light. This model could also be used by businesses to recommend additional products based on the recipes that their customers are cooking. For example, businesses could suggest buying birthday candles or champagne to someone who selects a cake recipe.
In our next steps, we would like to expand our analysis to all2 million observations in the dataset. This would require us to work with big data on platforms such as Google Collab or Databricks. We would also like to expand our LDA query model to keep tuning it for improvement and update it with new data points.
Having already started a Dash website for this project to host live recipe searches, we would like to have the site go live.. In the site, it would be nice to add common search filters, such as โbreakfastโ and โvegetarian.โ Lastly, we would like our model to learn from user behavior, so whenever a user searches for recipes they could see if- if they made it and liked the recommendations.