Identifying Patterns in Healthcare Fraud
The skills the author demonstrated here can be learned through taking Data Science with Machine Learning bootcamp with NYC Data Science Academy.
Healthcare Fraud in the U.S.
The US healthcare industry is one of the largest in the country. In fact, in 2020, healthcare spending accounted for almost 20% of the countryโs GDP. It is an unfortunate reality that fraudulent activity is an increasing issue, especially within lucrative industries often targeting vulnerable populations. And in healthcare, although they make up a relatively small percentage, fraudulent claims carry a very high price tag. In fact, it is estimated that losses due to fraud add $100 billion to annual cost of healthcare in the US. Healthcare fraud is undoubtedly a serious issue that requires attention, as the impacts from fraud can cause major losses to insurance companies and more importantly to individual patients.
Types of Healthcare Fraud
What are the types of fraud committed by providers?
- Double billing: Submitting multiple claims for the same service
- Unbundling: Submitting multiple bills for the same service
- Phantom billing: Billing for a service visit or supplies the patient never received
- Upcoding: Billing for a more expensive service than the patient actually received
About the data
- Inpatient records: over 40k records, includes patient/provider/ doctor IDs, date of claim, date of admission, diagnosis & procedure code, cost/reimbursement, etc
- Outpatient records: over 500k records (with the same features as inpatient records)
- Beneficiary records: over 130k patients with demographic information & associated chronic conditions
- Providers with fraudulent flags: list of all provider IDs indicating potential fraud
Distribution of Potential Healthcare Fraud in Claims
Providers with fraudulent flags only made up about 9% of all providers. But these providers were responsible for a much higher share of the claims comparatively, especially within inpatient claims; we can see that theyโre responsible for over 50% of these claims.
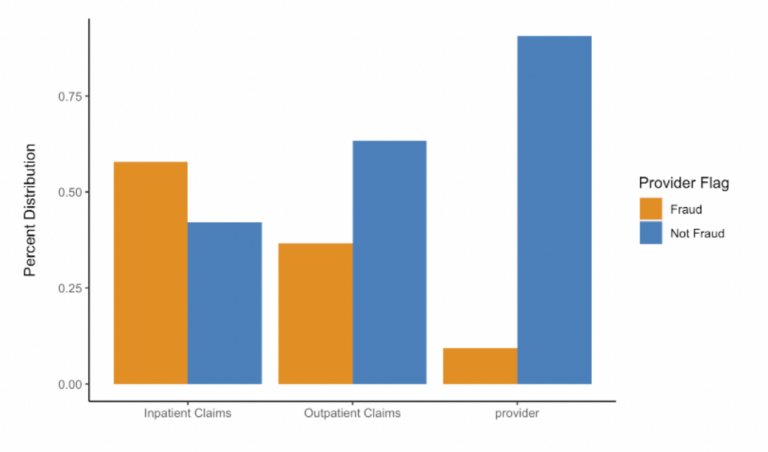
In addition to the distribution of patient claims, when we look within each individual claim; providers that are flagged as fraud on average make more diagnoses per claim and add more procedures per claim (so this may be indicative of signs of phantom billing or upcoding where patients are billed for a service they did not receive). With more diagnosis and procedures billed per claim, the financial impact is inevitably higher for claims associated with fraudulent providers; their average claim reimbursements are about twice as high compared to non-fraudulent providers.
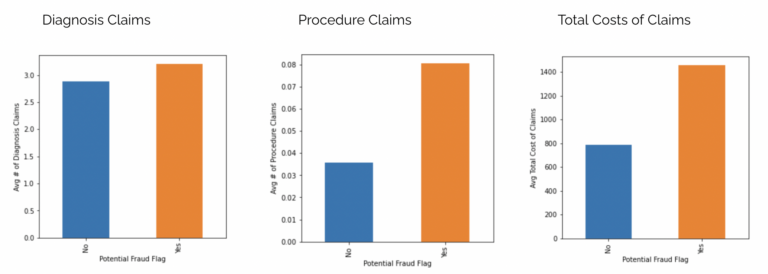
Patient Analysis
Age
Next, I analyzed patient data to see if I could find any trends that would indicate whether or not there are certain populations that are more susceptible to fall victim to healthcare fraud. And if there were, that would not only be beneficial information for insurance companies for better fraud detection training but also for patient awareness.
Looking at the patient dataset, there are large volumes of claims for the ages 66-90.
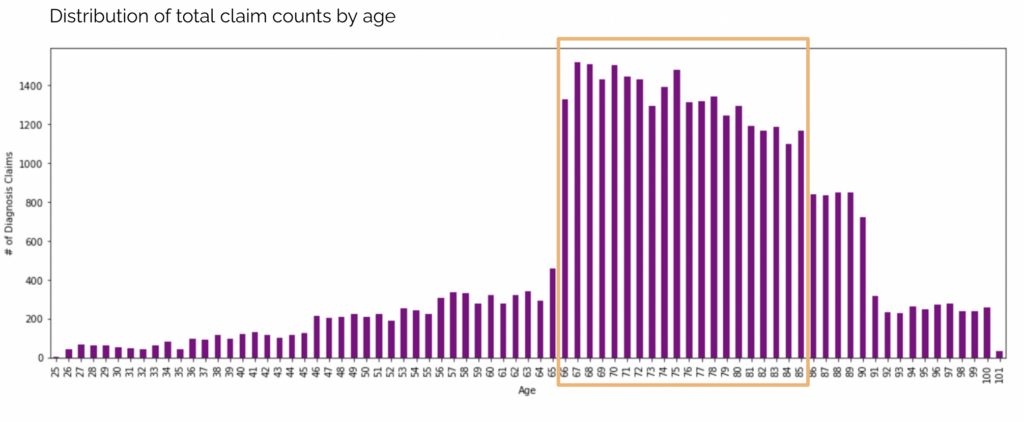
Considering the fact that there is a high spike in claims for the medicare age group along with the perception that the elderly are an easy target for fraudulent activities, I wanted to see if there was any indication of providers targeting this group.
Are older populations at higher risk of being targeted for fraud?
We learned earlier that fraud providers file higher reimbursements per claim, process more diagnosis codes and procedures codes (with greater impact on inpatient claims), so could this be due to longer hospital stays?
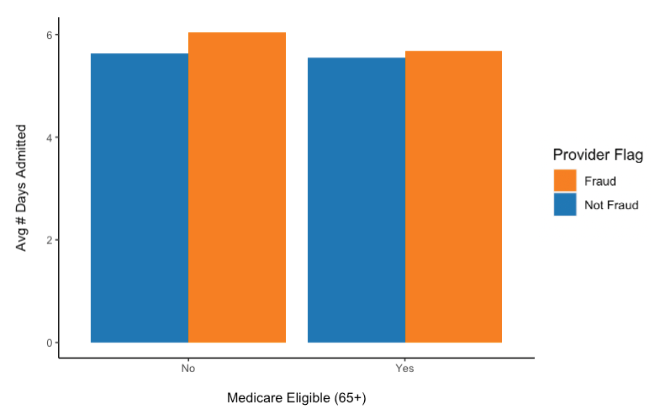
The comparison above does not show a significant difference amongst the average number of days admitted between fraud and non-fraud providers in either age groups.
Do fraudulent providers have higher distribution of older patients?
Next, I wanted to see if fraudulent providers target older patients, by either duplicating their claims or faking their data. If so, there would be a higher distribution of older patient claims for these fraud providers.
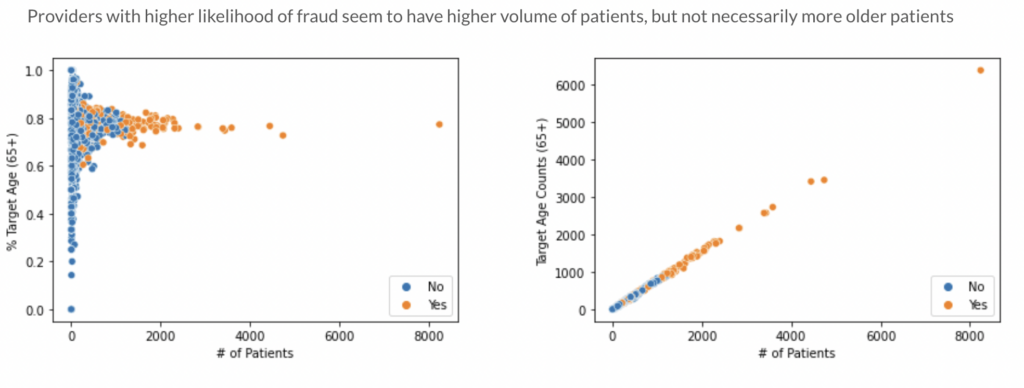
Referring to the plots above, the left chart demonstrates a scatterplot that marks each provider and the total percentage of 65+ patients they have. It seems for non-fraud providers, the distribution varies amongst providers. You have some that have few older patients and some that have higher percentages. For fraud providers, they seem to have more concentration in the higher percentages.
However, aside from having a higher proportion of older patients, the stronger indicator seems to be overall volume of patients. The more patients a provider has seems to be an indicator of fraud here.
The chart on the right looks at the same relationship but instead of percentages, it is portrayed in counts. And this view seems to support our analysis that providers with the most patients (more than 1,800) are flagged for potential fraud. Having higher than normal patient counts could be an indication of faked or duplicate claims.
Chronic Illnesses
The next patient population I looked that was patients with chronic illnesses. I wondered if a patient having multiple chronic illnesses made them more susceptible to fraud? This could make it easier for providers to charge for more services along with higher reimbursements.
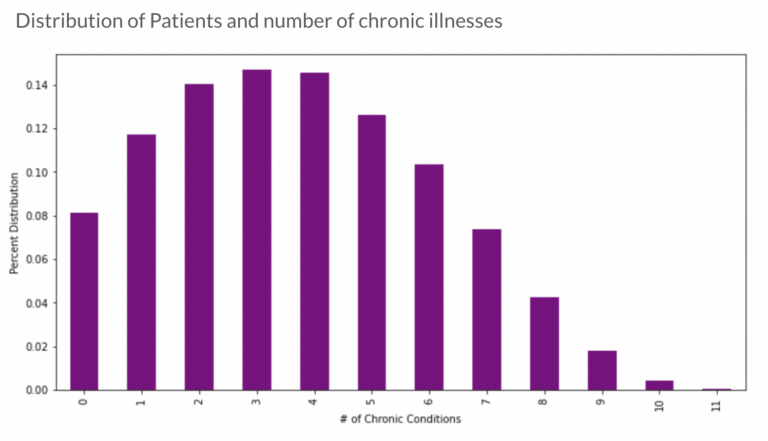
From the patient dataset, there seems to be higher distribution of patients with 2 or more chronic illnesses.
Are people with more chronic conditions targeted?
Looking at the comparison of average costs for patients by number of chronic illnesses, it appears that fraud providers charge more per claim across the board but having more chronic conditions does not necessarily mean that the difference will be greater for these patients.
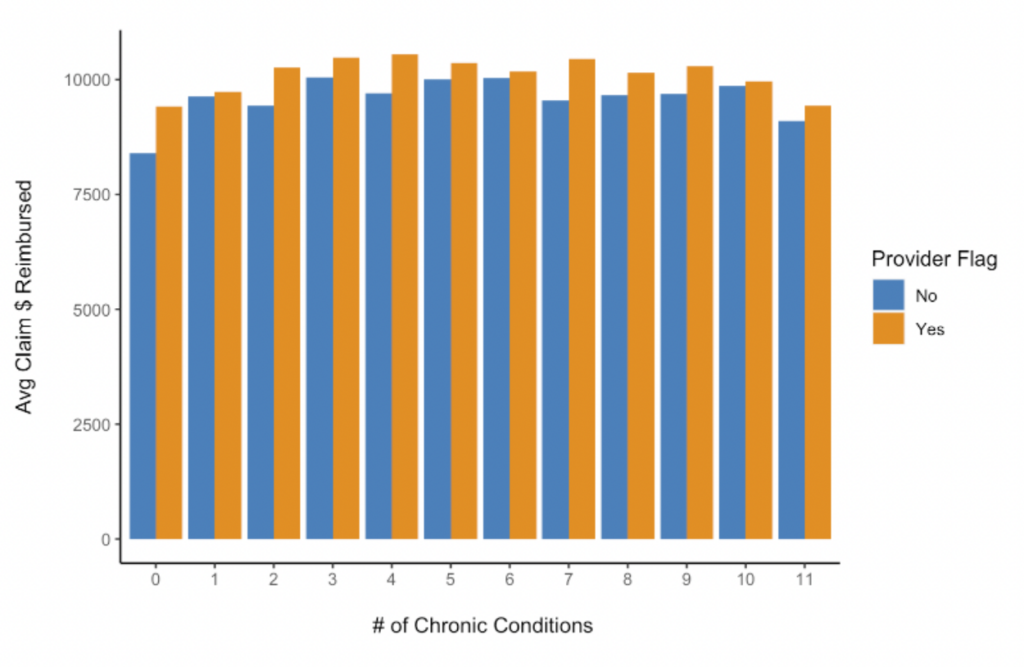
Provider Analysis
Signs of duplicate claims
Lastly, I looked into provider focused trends to see if there were some characteristics amongst fraudulent providers that could be extrapolated, which would be useful information for insurance companies to be aware of.
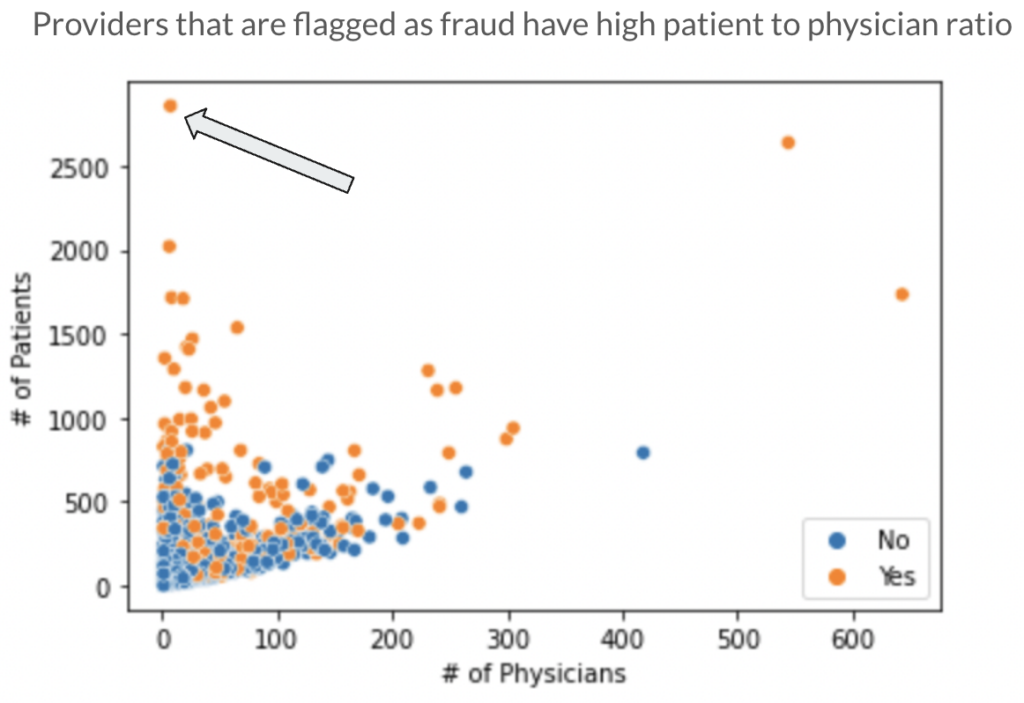
Above, we see the relationship between the number of patients and physicians for each provider. The providers that have a relatively small number of physicians but very high patient count are more likely to be fraud. This might be an indication of providers who duplicate their patient claims. Using the mark indicated by the arrow as an example, a provider with less than 20 physicians that have over 2,500 patients seems highly suspicious.
Double Billing
I then looked for a different type of duplicate claims.ย Specifically, instances where a provider charged a patient twice for the same amount.ย For example, if provider A charged patient B $5,000 in two separate claims. There could be valid reasons for a situation like this if the patient had the same procedure done twice, or if they had two different procedures that happened to cost the same amount. But it also could be an indication that the provider is faking patient claims, particularly if it occurs frequently.
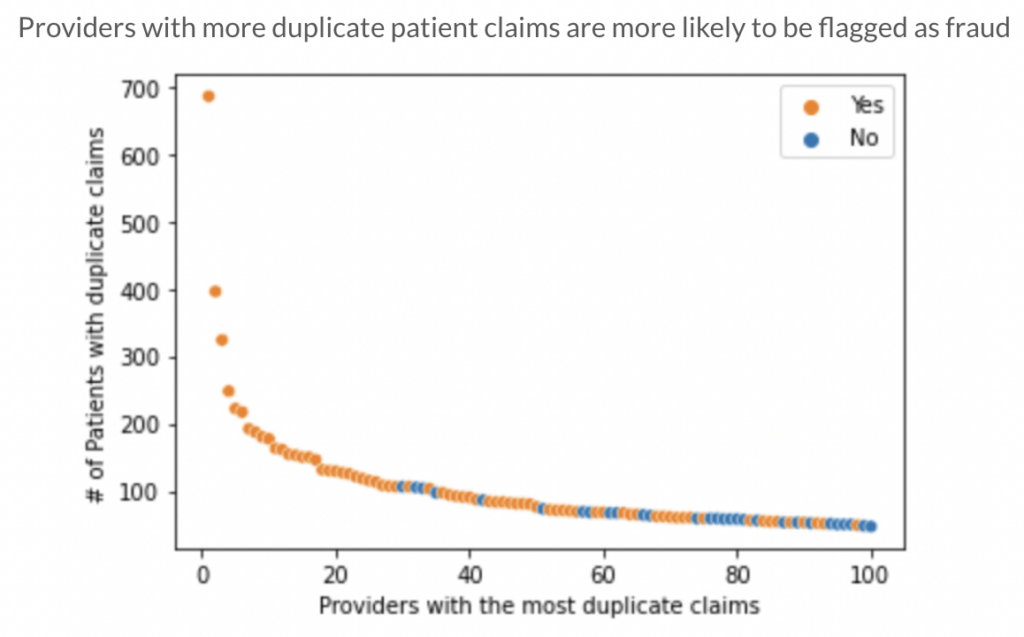
The chart here shows the top 100 providers who had the most duplicate claims of this type. The x axis here is by rank so the left most point is the #1 provider with the most duplicate claims who had almost 700 different patients with duplicate claims.ย The potential for fraud here is obvious. It is unlikely that a single provider has a reason to duplicate claims on 700 different occasions, particularly when most providers hardly ever have duplicate claims.
The providers with the most duplicate claims (ranked in the top 30) are all flagged for potential fraud. As the rank decreases along the x axis, we start to see more blue points, signaling that the potential for fraud decreases with less duplicate claims.
Conclusions
- There is strong evidence of potential fraudulent providers, processing more claims/diagnosis/procedures, thus leading to higher claims amounts.
- There was no clear indication whether there are specific populations that fraud providers target, there is likely a more sophisticated approach so that they are not flagged as easily.
- One of the strongest indicators of fraud were high patient volumes, providers with more patient claims and patients had higher likelihood of being fraud.
- Providers who also have high counts of duplicate claims where the same amounts are billed to a patient more than once, are also likely to be fraudulent.
Next Steps:
As next steps, I would like to explore the additional features included in the patient data set. To start, looking at diagnosis codes to identify how providers may duplicate or upcode claims in order to help insurance companies & patients for a better ability to spot fraudulent activity.