Python Shows Factors Influencing University Retention Rates
Introduction
It is often stated that the responsibilities of an institution are to strengthen their academic credentials, provide an enriching experience for their students, and ensure that their students succeed. An Office of Academic Success is one that focuses on these factors, and tries to ensure continuing completion and retention rate. Retention rate is defined, by the Department of Education, as the proportion of those students who returned from one Fall semester into the next. But why is retention rate important? Schools with higher retention have higher completion rates, which means more income per student and encourages federal funding. A high retention rate also indicates academic stability, and therefore would seem more appealing to prospective students. Students are given an opportunity, it is therefore expected that they see this opportunity through.
The Question: The Struggle to Achieve Retention
Universities attempt to use predictive analytics to determine which students need the most assistance. This usually comes down to trying to guess which factors most influence retention rate, and then attempting to provide assistance to these students. The students in most need of assistance are often classified as "risk" students. Usually, the goal is to boost accuracy and quality of data, which can then be implemented to determine the appropriate means to act. Where would one start with getting this data, and what factors influence retention?
Data: A Case Study and College Scorecard
The easiest way to actually see factors influencing retention is to look at a university system itself. So, scraped 2016 data from the Texas University System was used as an example case study. This includes basic student-by-student data focused on GPA, aid received, and basic background of the students (such as if they were in-state or out-of-state). More crucially, students were defined as either having returned sophomore year (Retained) or not (Not retained).
College Scorecard is the Department of Education's publicly available dataset of over six thousand universities. It covers "transparency" of the universities. This includes retention, debt and repayment, earnings, and more. It treats retention as a proportion, and also divides it between full-time and part-time, and those retained from less-than-four-year institutions and four-year institutions. For the purposes of this study, the type of institution was combined. While College Scorecard does cover 1996-2022, the most recent Fall cohort (2021-2022) was used. If you would like to see what the actual variables mean, here is the technical documentation. All of the information on how this was found can be found on Git.
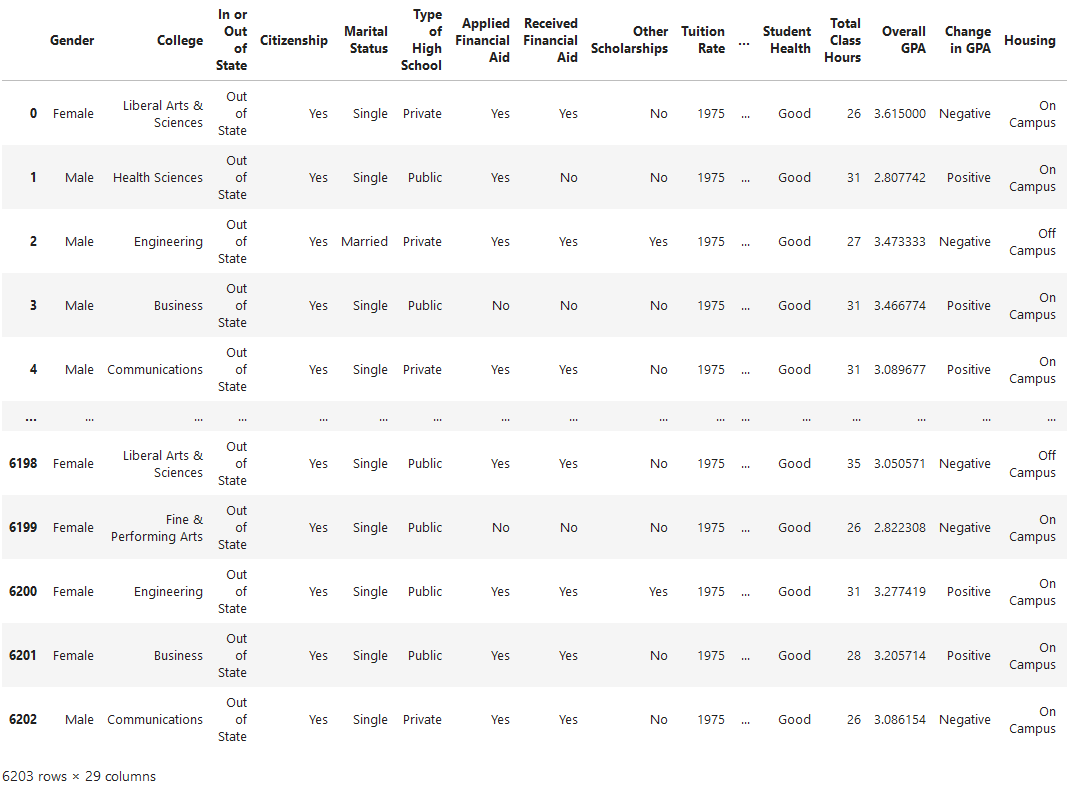
Texas University System Data
Analysis: Texas University System Case Study
Educational Factors: GPA
Usually, an early measure of student success is through academic success. The assumption therefore is that, if a student is doing well, then they are more likely to come back the following year. In the case study, the university system reported both high school GPA and overall GPA of the students by the end of the Fall semester. GPA is often used as a "per-calculation" on if and when to act on students who might possibly need assistance in regards to maintaining retention. To actually test this, the data was divided between "high school GPA" and "overall GPA" and then "retention" was used as the categories. It was also grouped by gender to see if there was further possible variation.
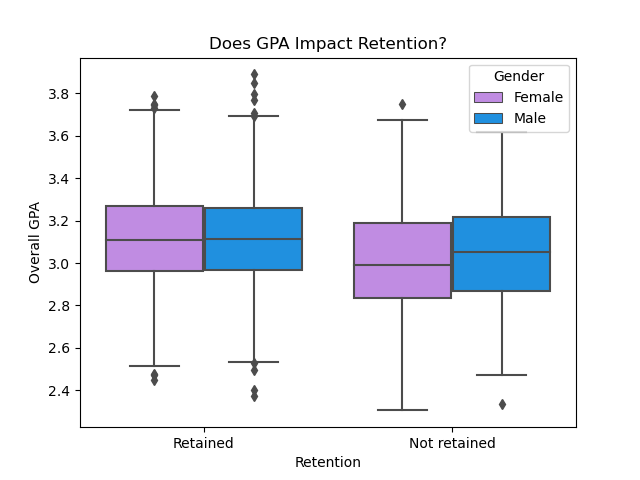
Overall GPA and Retention
Here, we can see a slight difference between the overall GPAs of those retained and those not retained. More specifically, the actual mean difference is about 0.09 using a t-test. According to the same test, this difference is also statistically significant (p-value < 2.2e-16). Further, it would seem that females in the retained category tend to have a higher GPA than when they are in the not retained category. Does this actually mean anything, though?
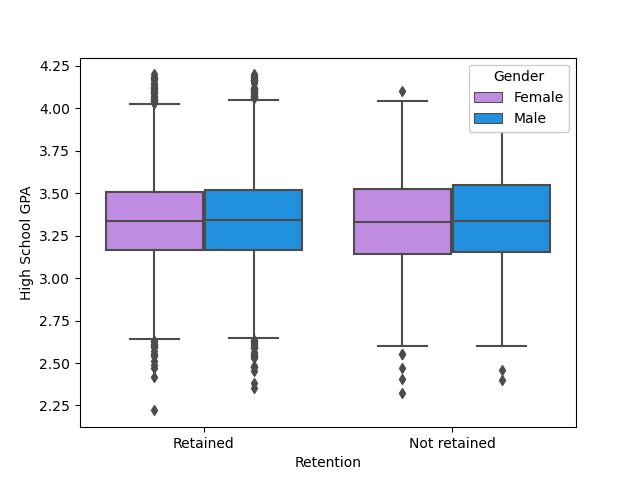
HS GPA and Retention
Alternatively, when we see HS GPA, it shows us that the GPA measure might be misleading. The retained category clearly shows how offset the results are by the outliers, which is likely from advanced placement courses that HS students took. Usually, above a 4.0 GPA is unable to be achieved at a standard university. Yet still, even in the retained category, there are so many low GPA numbers to offset the high GPA numbers that the mean is centered around the median (of around 3.34). This is notably higher than the overall GPA, yet the result is not statistically significant in comparison to the not retained category (with a p-value of 0.8433).
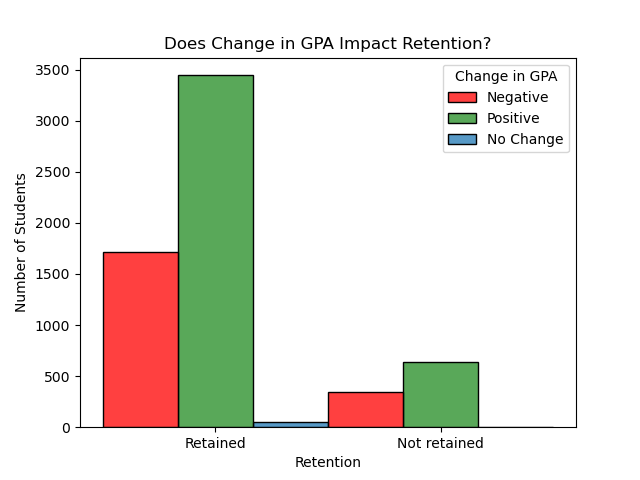
GPA Rate and Retention
To better demonstrate the effect GPA has on retention rates, the "direction" of the GPA over a student's first year was taken and categorized as either moving in a negative or positive direction, or having not changed at all since starting the Fall semester. While here it would "appear" that most students who were retained had a positively changing GPA, those who were not retained also had positively changing GPAs. In fact, because the p-value was 0.2266, there was no significant association between the two categorical variables. There was little difference in what was observed and what was expected (it was actually close to zero, meaning almost equal, with an x^2 of 2.969).
The Greatest Burden?: Debt
GPA stability is usually at least a "factor" in determining student success, but GPA itself is usually the result of multiple other factors that could also be influencing retention. Instead, usually the largest factor in determining whether or not someone even attends college is if they received financial aid. TUS did not provide the "amount" of financial aid provided, but it did provide whether if someone's application for financial aid was successful.
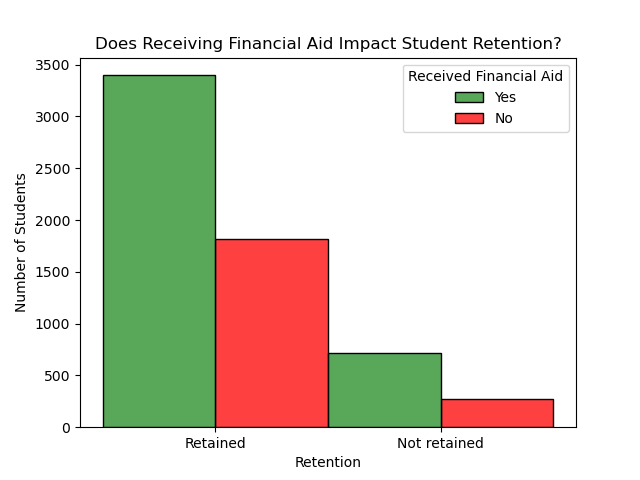
Receiving Financial Aid and Retention
The idea is that debt cause individuals to struggle with academic stability, and therefore results in lower retention rates. Here, if a student was actually approved for a loan, it would be expected that they would have an easier time dealing with the managing the various financial burdens associated with attending colleges. Right away, it's clear most students that receive financial aid are more likely to return the following year. In fact, there is a statistical significance between the variables with a p-value of less than .05 using a Chi-squared test. The problem is that this is a categorical variable that does not measure degree.
Analysis: Comparing the Case Study with College Scorecard
Academic Achievement and Receiving Loans
College Scorecard is an institution-level dataset, so it gives a broader look at the actual influences on student retention. The two main factors from the Texas case study, loan receiving rate and GPA, can be somewhat merged with the College Scorecard with a few caveats. First, College Scorecard measures retention and rate of receiving loans as ratios, not categories. Secondly, there was no GPA measurement for College Scorecard, and the only per-calculation that could be provided would be SAT scores.
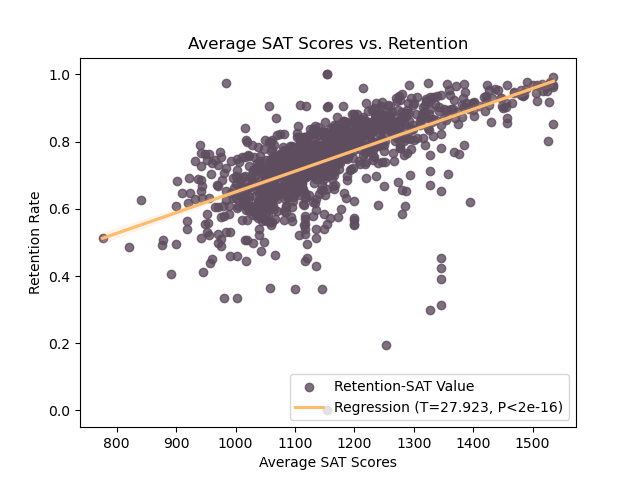
SAT Scores and Retention
Since the Texas case study showed a statistically significant difference in means between those retained and those not retained, it is not surprising that there is a positive relationship between SAT scores and retention rate. This relationship is not only significant, but a regression model was used to demonstrate the relationship between the variables and the strength of said relationship. However, there are multiple issues to this. The first is that these SAT scores were "supplied" by the universities themselves, and most universities are not required to provide SAT scores to begin with. Secondly, universities often take SAT scores into account during the application process. This is not the same as GPA. Therefore, it is expected that the university is already accepting students they feel would do well at their school so they can maintain non-risk students. Does this mean this data can be dismissed entirely? Not likely, because it is somewhat representative of "entrance GPA", yet it was shown that change in GPA may not matter much.
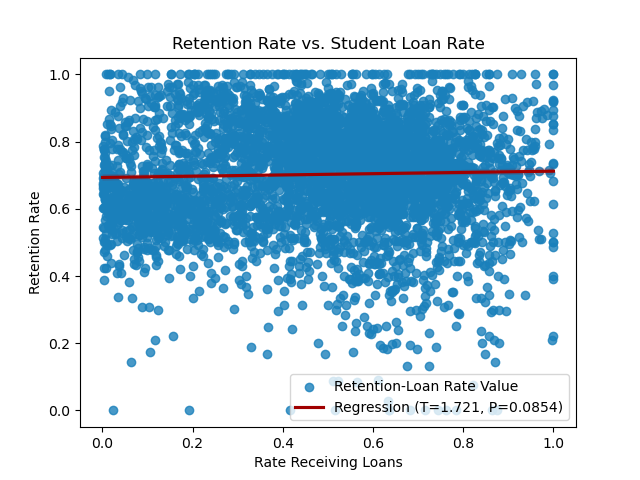
Student Loan Rate and Retention Rate
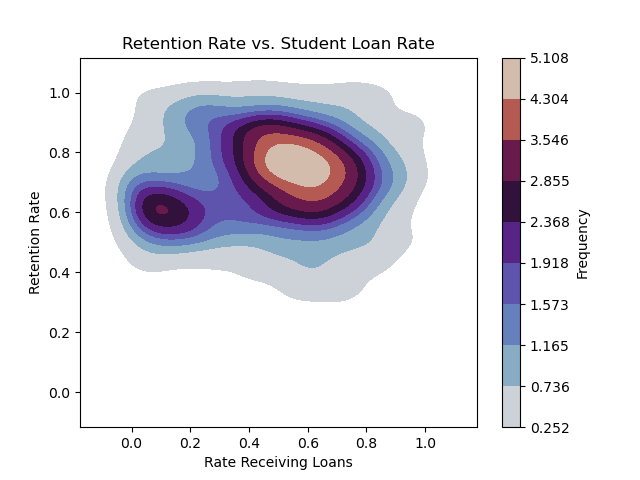
Student Loan Rate and Retention Rate (Heat Map)
Loan receiving rates here and retention seem to contradict what the data from the TUS provided. For one, the size of the error has now changed significantly compared to the SAT scores. More importantly, this graph is no longer statistically significant, as shown by the two offsets in the heat map, which causes a near horizontal line of best fit. In fact, this is so insignificant that it suggests near-even distribution and receiving loans as a non-influencing factor in retention. The t-value shows almost no difference relative to variation in the data, with only a slightly positive direction of the slope. Does this suggest the TUS was an outlier?
Poorly Equipped with Poor Outcomes
An alternative answer rather than just a flat-out "no" to the previous question would be to suggest an alternative way to measure debt. College Scorecard had the median accumulated loan debt by all student borrowers, separated by graduates and undergraduates. For one reason why this wasn't used, most first year students can't be compared to undergraduate debt since they have not paid that full cost yet. The second reason why this measurement wasn't used will be discussed in the next section.
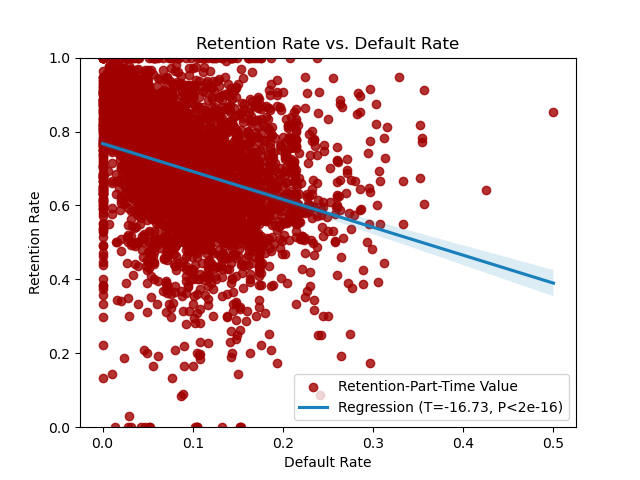
Two Year Default Rate and Retention
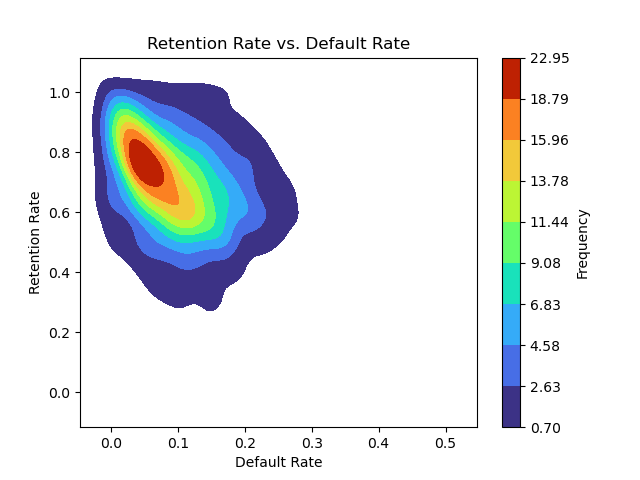
Two-Year Default and Retention (Heat Map)
Default rate is the percentage of borrowers who defaulted on their federal loans after two years. In doing so, they may lose access to federal aid. It is used as an "institutional accountability metric". In the first year, most students are under loan deferment, so defaulting on loans wouldn't apply to them. However, if the previous cohort was defaulting and there was also a trend in low retention going along with it, then it tells us two things. One is that loan receiving rates may not matter, but the ability of a student to deal with loans does, and different institutions have different aid programs. Two, we can see from the heat map that most students do not default and therefore have a high retention, but when they do start defaulting it's statistically significant in the negative direction.
Costs Rising? An Unexpected Trend
Median debt was not included because tuition costs would be a more accurate depiction of the financial burden of first-year students. This was divided into both in-state and out of state tuition costs per semester, defined as the "price charged to attend".
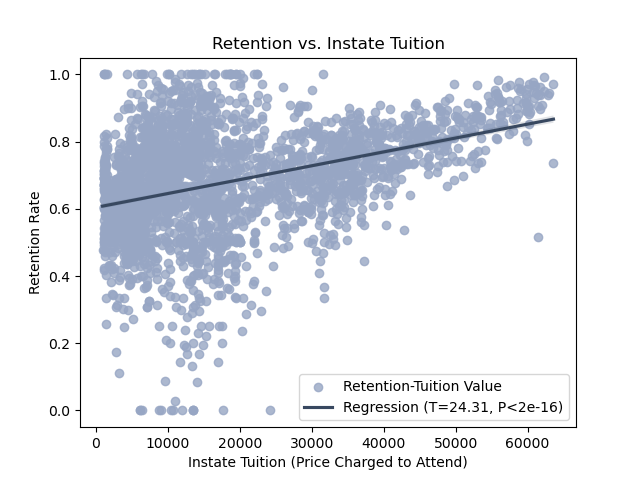
Instate Tuition Costs and Retention
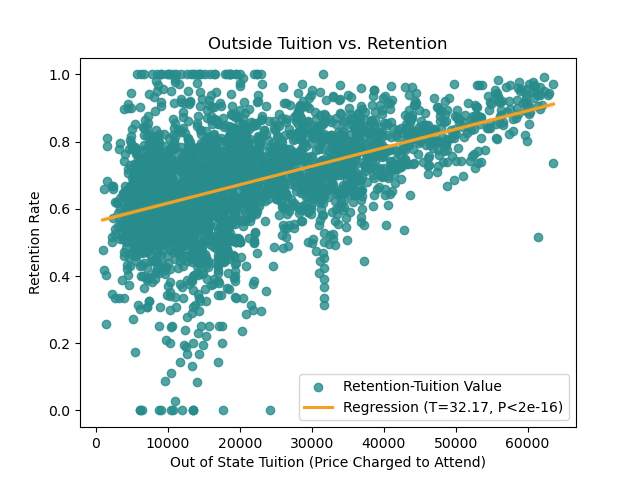
Out of State Tuition Costs and Retention
Default rate showed us that institutions not equipping their students well enough to deal with their financial burdens are more likely to have lower retention rates. These graphs, however, show us that higher tuition rates lead to higher retention rates. Is this contradictory to the default rate assumption? Maybe not, because we know that if a student is spending a lot of money on a university, this is an investment for them that they would like to see through. These universities are also more likely to be better equipped to deal with poor retention rates. The better question, then, is if tuition influences default rates? A simple analysis of this actually did reveal that, in a weighted regression, lower tuition rates were associated with much higher default rates, so we can conclude that maybe it's not the cost of the university harming retention, it's financial outcome.
Easing the Burden: Income
The largest factor influencing financial burden would be an individual's ability to pay for it. It was curious to see that tuition had a positive influence on retention, but what about actual income?
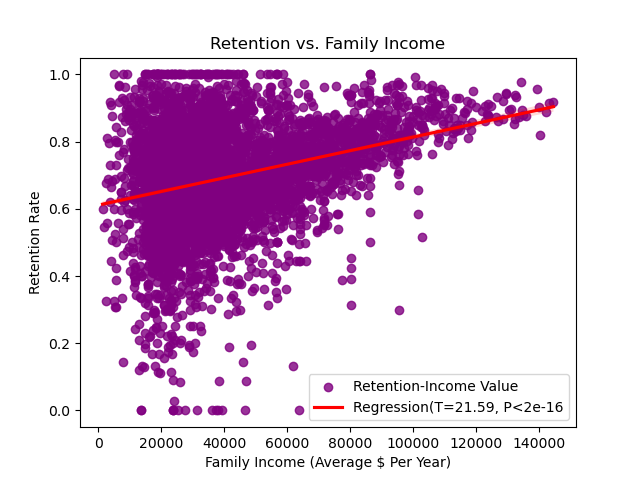
Family Income and Retention
Here, most of the range is centered around the national annual income in 2021 (around $45,000), but for those that have more than that income, the trend clearly goes in a positive direction. However, this result should be taken with caution, as income means the ability to afford a better college, which we saw before led to a more clear higher retention rate. That could mean income in itself isn't leading to a higher rate, rather it's just leading to a better college, which is allowing for a higher retention rate. Individual income was also examined, but most individuals attending college make the same income, so the graph had no direction to it.
Is it the Facilities or the Cost?
College Scorecard divided retention into full-time and part-time retention. While what has been analyzed has so far been first-year, full-time undergraduates, there is the suggestion that part-time students are less likely to come back to the university their sophomore year.
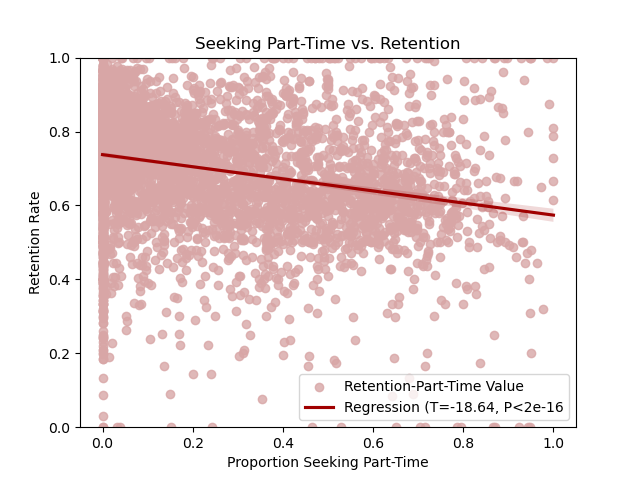
Percent as Part-Time and Retention
From the graph, it would appear that if the institution has a larger percentage of students that are part-time then they have lower retention rates. The automatic blame for this is that part-time students are less likely to have access to the facilities and programs designed to maintain retention. This includes academic clubs, outreach programs, or even being on the university grounds to begin with. A significant reason why someone would choose to be part-time is because of other burdens outside of school, or even cost-reasons (although sometimes part-time pays the same as full-time). These distractions for them may seem to matter, then, more so than actual cost in relation to retention.
Easier for Some?: The First Generation
First-generation students are often focused on in the "per-calculation" in determining "risk" students. These are the students that have had no parents who had attended college. The reason this is a focus is because, if a parent attended college, they can make up for an institution lacking in facilities to assist a student that is lagging behind. Parents of non-first generation students also tend to be wealthier, and may already know which colleges have higher retention for students.
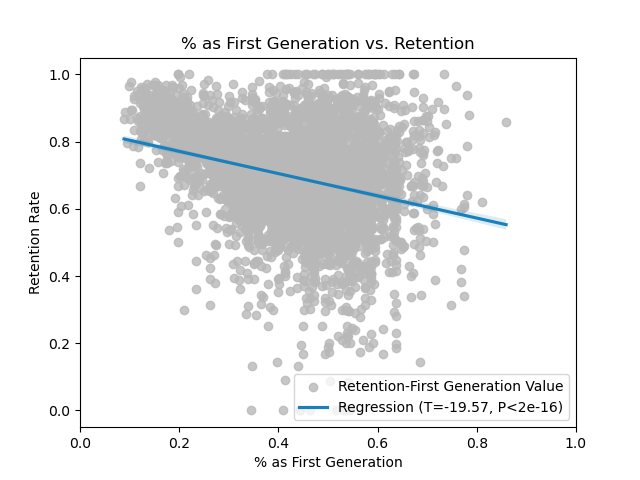
First Generation Students and Retention
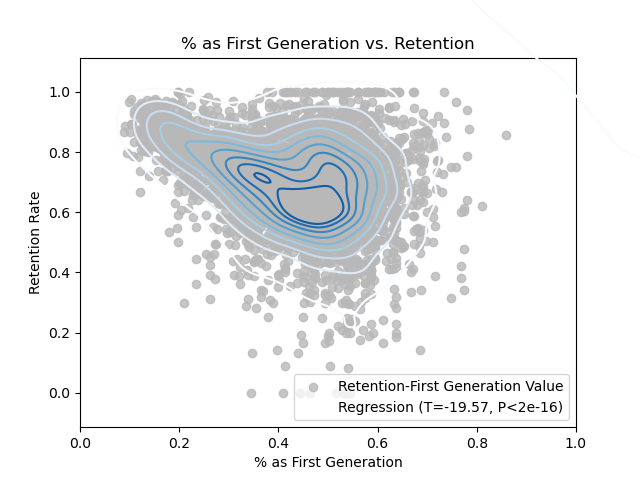
First Generation and Retention (Density)
For one, it's shown from the density plot that most colleges have a decent percentage of students that are first generation. However, this also shows two things. One, those colleges that have few students that are first generation tend to have very high retention rate, and, when there is this lack of uniformity, the retention spreads out in a general negative direction. However, because multiple colleges seem to also have a high retention at the same percentage of first generation when others would have low retention, it can be suggested that the first generation starts mattering less when it reaches 50%. This could be explained by the first generation students being able to use the school facilities are talk with their peers to make up for where their parents would have lacked.
A Final Factor: Age
Most students that go to college are usually those that are younger. The reason is typically because they just graduated high school, or because they don't have the typical responsibilities of later adulthood.
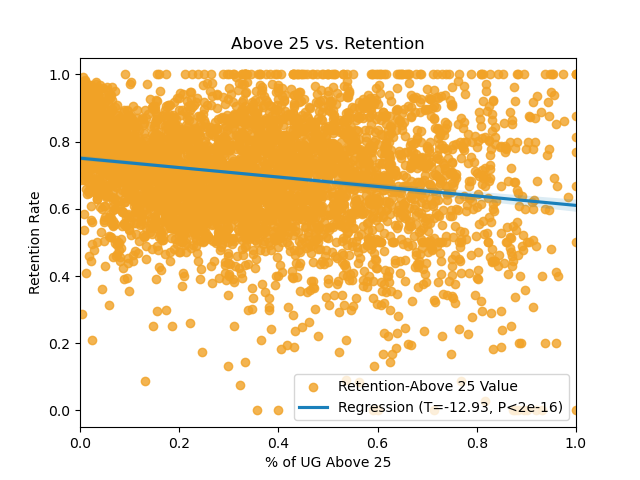
Ages Above 25 and Retention
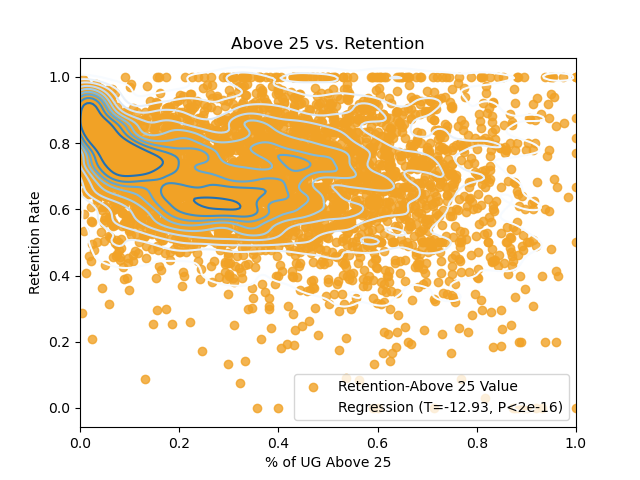
Ages above 25 and Retention
The "above 25" is an interesting variable because College Scorecard figures that most students who enter into college are 24 and below. Here, we can possibly see why as there is a downward trend in retention between those who are 25 and above and retention rates. The density shows a cluster at the less than 20% with a high retention rate, then a clear downward trend, and then it spreads out haphazardly. It should be critically focused on that this does not mean "age", just "25 and above", so a university could technically just have 26 year-olds and still qualify for the metric. Still, this is telling that those that are older typically have more responsibilities and become overwhelmed with such, no longer able to attend college. Or, the opposite could be true. They could have more opportunities and could be more likely to transfer, which College Scorecard doesn't take into account.
Conclusion: What Can Be Done?
Predictive modeling is used to make business decisions based on trends. The goal is to make enhancements to current systems by finding the factors influencing what we are measuring, in this case retention. Here, universities can look at the factors influencing retention in order to provide better services to their clients and develop more means to engage with them by: providing stronger communication, enhancing their per-calculation/risk models, and create a more enriching experience. We've established that first generation, low-income students who are part-time are probably more likely to be at risk. Academic success "may" be an influencing factor, but "placement" might be the more important factor, since change in GPA wasn't statistically significant. In addition, a university's ability to deliver on goal outcomes may be more of an influencing factor on retention than its actual debt burden. These factors usually compile with each other. In other words, a sense of belonging and a feeling of stability and comfort in knowing that a student can secure a higher-paying job to pay off their investment, may be more important than the investment itself.
Future Works: Issues and Suggestions
There were several issues when formatting this data. First, the College Scorecard data was very large and had to be trimmed. It is usually ill-advised to lose data in calculations, but the Scorecard had multiple NaN's, which were dropped instead of having means replacing them. Further, there were also the "Privacy Suppressed" values, which also had to be dropped. Transfer rates are often subject to such "suppression", and colleges aren't obligated to provide such rates. That means that many of these results could be distorted by higher transfer rates in certain colleges. A transfer doesn't necessarily mean they aren't progressing to a sophomore year. This could especially apply to part-time students. Much of this data also had a lot of spread, which suggests a lot of error and more emphasis on weighted regression instead of linear regression.
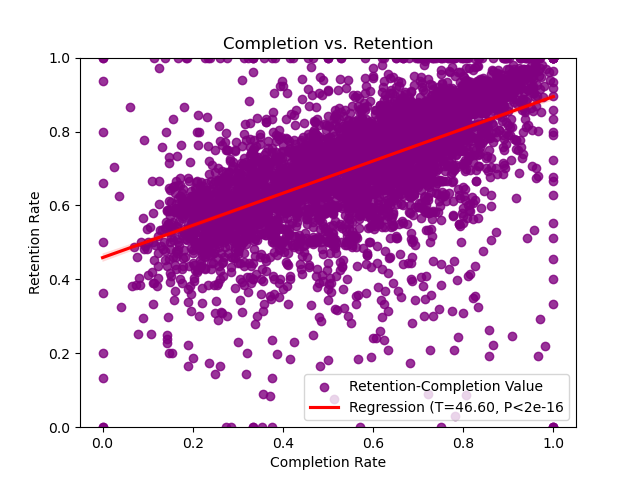
Completion Rate and Retention Rate
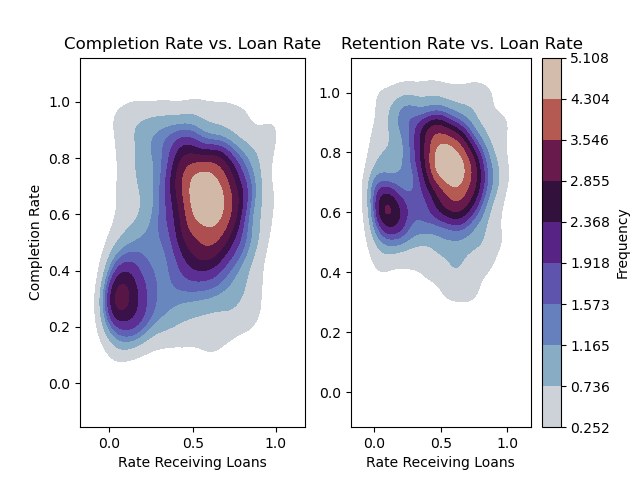
Completion Rate and Retention Rate (Heat Map)
College completion is whether a first time, full-time student completed their academic goals within a set amount of expected time, usually 150%, but College Scorecard does measure 100% and 200%. It is correlated with retention rates, where the variables can be switched and if one increases the other increases at an institution. In fact, they are so correlated that, as shown in the heat map, many of the results are the same. It would be interesting to see if the factors that affect retention also affect completion, as the ultimate goal of a university is to have students graduate.
Retention was, as mentioned before, divided into part-time and full-time. Seeing the actual difference with part-time retention as the variable being measured would also be interesting, as it might be able to pinpoint how much time being invested into the institution actually affects retention. For this data, schools that are less-than-four-year institutions and four-year institutions were combined. Grouping the data instead by L4 and 4Y institutions might help better understand the influencing factors. One could go further with this by grouping institution type (EG public and private), as well as degree type offered (which the TUS also had a variable for).
This data could be separated into quintiles or similar to better view the results and prevent clustering, at the risk of reducing the data. Most significantly, College Scorecard stores data for multiple years, not just the most recent one. Taking a time-series analysis to see how the trends in retention have changed overtime, and where those factors come from, such has how it has been impacted due to external factors like the coronavirus epidemic, would be an important next-step. Other case studies would obviously help as well, since the TUS is just one type of school in a certain region. Still, this could be a stepping-stone for developing the attributes of first-year students that have a higher or lower probability of returning for their sophomore year.