KnoWhere: An App for Automatically Profiling your Commute using Smart Phone Sensor Data
Introduction
The commute is a great equalizer. Regardless of location or income, nearly 95% of working Americans use a car or take public transit to go to and from work. In the grand scheme of things, a one-way commute does not take very long. Yet, when repeated hundreds of times each year, time spent commuting ends up being a sizable part of our lives. A one-hour commute repeated twice a day, five days a week, over 52 weeks adds up to over three weeks doing nothing but commuting, each year. All of this time spent commuting inevitably plays a role in our happiness and well-being. While necessary, the average commute is at best tolerable and at worst miserable.
Knowing this, our team set out with one guiding question: What if we could use data science to make commutes more enjoyable and more useful?
We created KnoWhere, an app that for automatically profiling your commute using smart phone sensor data.
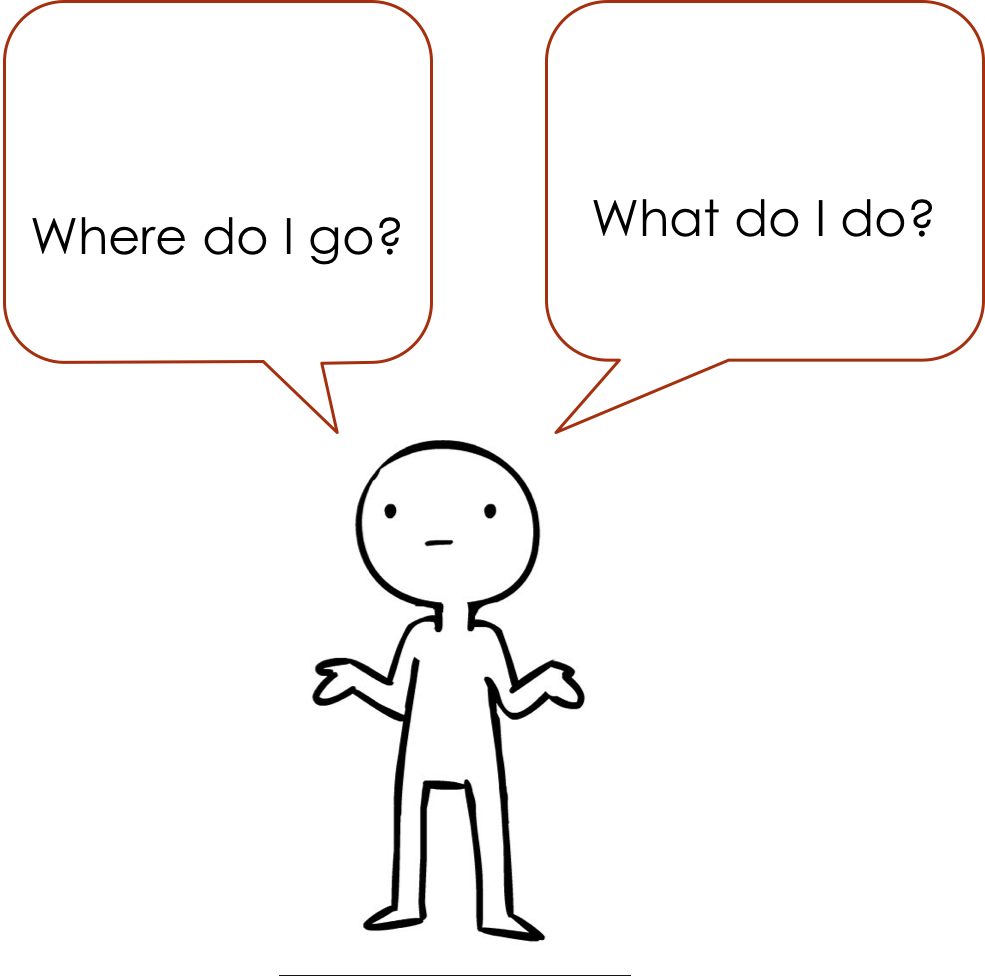
The central questions we are answering for users. We take a data-driven approach to improve businesses, so why not use the same approach to improve our lives?
The two main questions we wanted to answer for users are "Where do I go during my commute?" and "How much time do I spend on different modes of transportation?" Currently, a user who wants to answer questions like these has two basic options: rely on a corporation like Google to track you (see Google Timeline), or manually log their days with pen and paper. However, doing this manual work daily is cumbersome... and let's be honest, unrealistic! Automatic collection of data is far more efficient and doesn't require tedious and error-prone manual logging. Instead, we utilize smartphone sensors and data science to automatically collect and classify user commute activities.
While a variety of other apps on the market fill a similar niche, KnoWhere has a few unique features:
- We utilize low-power, intermittent sensing, so your battery life is saved for everything else your phone does that makes your commute suck less.
- You can and track your commute and location without selling your data to a corporation
- KnoWhere is, at far as we know, the only open-source application that can classify train travel, making it an ideal choice for transit riders.
How We Built it: The Frontend
The purpose of the frontend was to be a vehicle for the advanced modeling. It was designed to be intuitive, easy to use, and fun, while still providing a wealth of information the user could not find elsewhere. The technologies used were Python Flask for the backend, AngularJS+Bootstrap for the frontend, Leaflet for mapping, and Google Charts for graphs.
Essential components of the front end are user selection (included as part of the prototype but unnecessary after login implemented); date-range selection; a map to visualize the user's path; distance-traveled panel; locations panel; the commute activity panel; and the "fun" panel that tells the user how fast their commute would be if they rode a randomly generated animal. Who wouldn't want to know the time it takes to ride a bear to work?
How We Built it: Database
A major issue with our data was that every sensor returned different data. Some sensors returned strings, and others returned decimals. Even more complicated, some returned only one value while others returned multiple. The altimeter, for example, returned both a barometer pressure reading and a relative altitude, while the GPS returned measured latitude, measured longitude, latitude accuracy, and longitude accuracy. A standard MySQL database could not hold all the data without being extremely sparse.
To resolve this issue, we decided to use MongoDB as our database. MongoDB is a free and open-source database program based on NoSQL. Documents stored in a MongoDB database are structured similarly to JSON, which are very similar to Python dictionaries. Further, unlike standard SQL databases which must follow a relational structure, MongoDB has no such restrictions. The documents stored in a collection don't even need to follow a standard structure. They only need to follow JSON structural rules which store information in dictionaries (key-value-pairs) or lists of dictionaries. This open structure was what we needed since not all sensors returned the same number of data points. Finally, because our analysis code and website were all written in Python, which uses dictionaries and lists similar to JSON, this made MongoDB the perfect choice to fit our needs as reading and writing new data could be completed using Python objects.
To ensure that everyone on the team could access the data at any time, we launched an Amazon EC2 instance and ran MongoDB as a service. EC2 is Amazon's Elastic Cloud Computer service available under their Web Services (AWS). It allows users to quickly bring up a virtual server for use as extra computing power, or as a simple server that doesn't require the user to physically maintain. While many servers of differing sizes are available, to keep costs low, we selected a t2.micro server running Ubuntu. While this limited us to only 1 CPU and 8 GB of storage, we felt that since it was only running a database service, we would not need much computing power or space. Indeed, after over 1.5 Million entries in our database, we still had not used even 1 GB of our allotted space
How We Built It: Classifying Activities Using Machine Learning
Collecting data was performed using the Gathered app for the iPhone and the Sensor Kinetics Pro app for Android platforms. Both apps had significant disadvantages. Neither allowed for the consistent, reliable collection or for easy-to-process data. The sensors did not record at every time step and changing the frequency of collection only worked for some sensors. Data collection could be improved by building a custom app. This procedure is the next step in the development path for KnoWhere. Reported data is recorded at 5-second intervals for all sensors.
Activity monitoring was accomplished using hierarchical clustering for location determination with the barometer and GPS sensors. The agglomerative clustering algorithm with the number of clusters set to two was used to determine home and work locations. The cluster that occurred predominantly between 1-6 am was set to home, and 8 am to 5 pm as the work cluster.
The sensors used for commute activity classification were the accelerometer and magnetometer. The values for these are in x,y,z format and, as previously noted, recorded at ~ 5-second intervals. These features were engineered by first taking the magnitude of the x, y, and z values to arrive at single accelerometer and magnetometer values. A windowing procedure was then used to transform these into 35 features. Windows were set at 2,5,7,10, & 15 intervals. New columns were added corresponding to the mean, standard deviation, and maximum in the window.
These new features were then used in the modeling.
Activity classification used the random forest algorithm. Many other techniques were attempted, including a neural network using Keras, a gradient boosting machine, SVM, logistic regression, and extra random tree classifiers. We also used other methods including stacking methods and voting classifiers. None of these achieved higher accuracy than the random forest method. Hyperparameter optimization with the skopt package was used to find the optimal values for the algorithms. The values converged rapidly.
The results showed a 60% accuracy in test sets after fitting. The major failings were the inability to classify when the person was in the elevator and standing while on the train. We believe these failings would be fixed with more and higher quality data.
Conclusion
We have designed and built a system for automatically classifying the users home and work schedule, and common action on their commute using machine learning combined with a database and web-based frontend. Our platform should be extended to provide suggestions on how to improve end-to-end travel, based on a user's preferences and desired outcome for their commute. We believe that sensor data is a useful tool for describing a user's actions, and what KnoWhere begins to do by profiling a commute can easily be extended into other areas. Just like the long slog of our commutes, we know that we can improve our lives... bit by bit, day by day, over and over again.