MTA Light at the End of the Tunnel? Assessing Performance
Project GitHub | LinkedIn: Niki Moritz Hao-Wei Matthew Oren
The skills we demoed here can be learned through taking Data Science with Machine Learning bootcamp with NYC Data Science Academy.
For millions of New Yorkers who depend on the subway to get where they need to go, delays have become a way of life. Decades of mismanagement have taken their toll on the MTA, culminating in a series of serious incidents in 2017, including a fire and a derailment that injured scores of commuters. Eventually, the issues became too hazardous to ignore. The MTA responded by hiring a new president, Andy Byford, in January, 2018. Byford, who transformed Toronto's formerly lackluster public transport network into an award-winning system.
There is little doubt Byford did a great job in Toronto, but New York's system presents a unique set of challenges that would prove extremely daunting to pretty much anyone. With 472 stations to Toronto's 75, New York's system is older, larger, and runs 24/7. Any competent statistician would be quick to point out the tendency of regression toward the mean; perhaps Byford's success in Toronto was a one-off.
The Data
Curious to find out for myself, I decided to tap into the MTA's data to see whether Byford is really worth his salt. To help visualize any salient trends, I built a Shiny dashboard in R, analyzing six performance metrics: on-time performance, additional waiting time on platforms and trains, major incidents, service delivery, and elevator and escalator availability in the stations.
On-Time Performance
On-time performance, defined as the percentage of journeys ending within five minutes of their scheduled duration, has unquestionably increased during Byford's tenure, starting in January 2018:
MTA Extra Time on Platforms and Trains
In 2018, passengers could expect to spend an average of 1.6 additional minutes on the platform and 1.6 minutes on the train compared to their scheduled journey times. Those metrics have also improved considerably under Byford. Now, platform and onboard wait times are now less than 1.2 and 0.8 minutes, respectively:
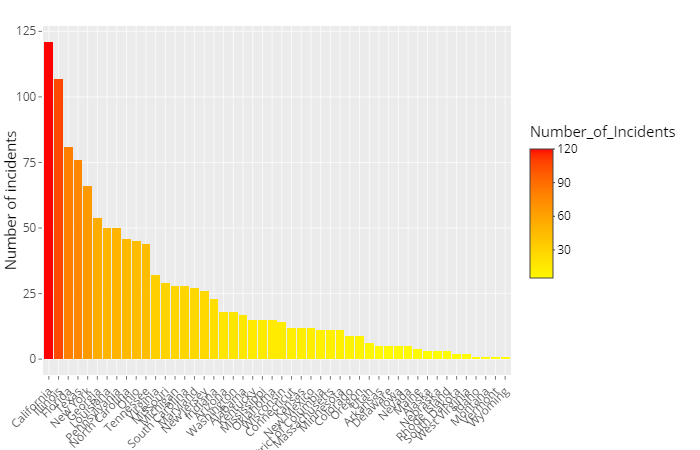
Elevators and Escalators
2019 has been a particularly difficult year for the subway's escalators. The proportion of functional escalators typically hovers between 93% and 95%. However, in March 2019, the MTA saw something of an escalator apocalypse, with over 8% of its escalators going out of service:
MTA Serious Incidents
2018 and 2019 saw a gradual decline in the number of serious incidents impacting subway service:
Signal failures have not seen a substantial improvement during this time. This is not a surprise, as New York lacks modern signalling systems on all but a few lines.
MTA Service Delivery
The data show a marked improvement in service delivery (the number of trains run compared to the schedule):
In the above chart, the blue area represents trains run, with the red representing the deficit between the schedule and reality. The red area has grown noticeably thinner over time. At the start of Byford's tenure in January 2018, the MTA ran 3,893 fewer trains than expected. In January 2019, that number decreased to 1,854.
Conclusions
Though not every metric has shown dramatic improvement, fixing the city's troubled subway system was never going to be easy. Based on preliminary results, it seems Andy Byford has been delivering on his committment to improve service. While New York's subway continues to underperform compared to most other cities, even the most jaded and cynical New York straphangers will concede that subway service is improving, and the data backs it up.