Marketing Analytics Combine Master/Slave Accounts by Python
The skills I demoed here can be learned through taking Data Science with Machine Learning bootcamp with NYC Data Science Academy.
LinkedIn | GitHub | Email | Data | Web App
Before I started working as analyst, I thought master/slave are only words for describing slavery in 18th Century, or for the sexual relationship in modern age. However, my thoughts are proved to be wrong. In the business world, it is a terminology to show the relationship about one main subscriber account to the related accounts under the same subscriber (company/individual.)
For engaging the clients better, company wants to categorize the dataset which contains these types of accounts: Master, Slave and Single Corporate Account. The original dataset we had from CRM system only shows the ID of Slave account, the ID of its master, and the other account type.
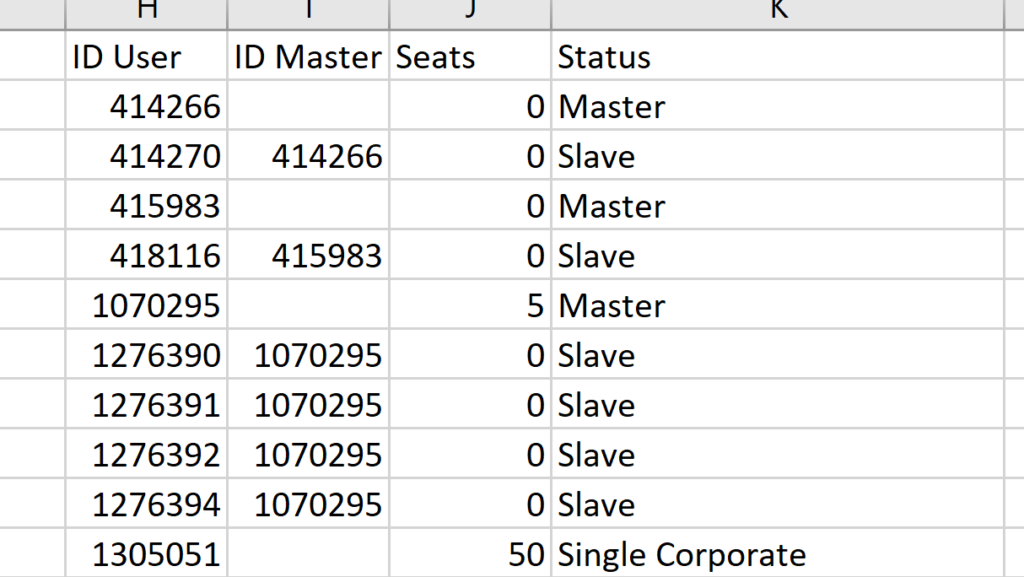
Our goal is to convert the above sheet into one categorized dataset --- it can show master and its slave accounts in one row, and the number of seats under this account.
In this way, we can clearly see the ID and number of Master/Slave Account, and also know those rows with missing data determine the single corporate accounts left.
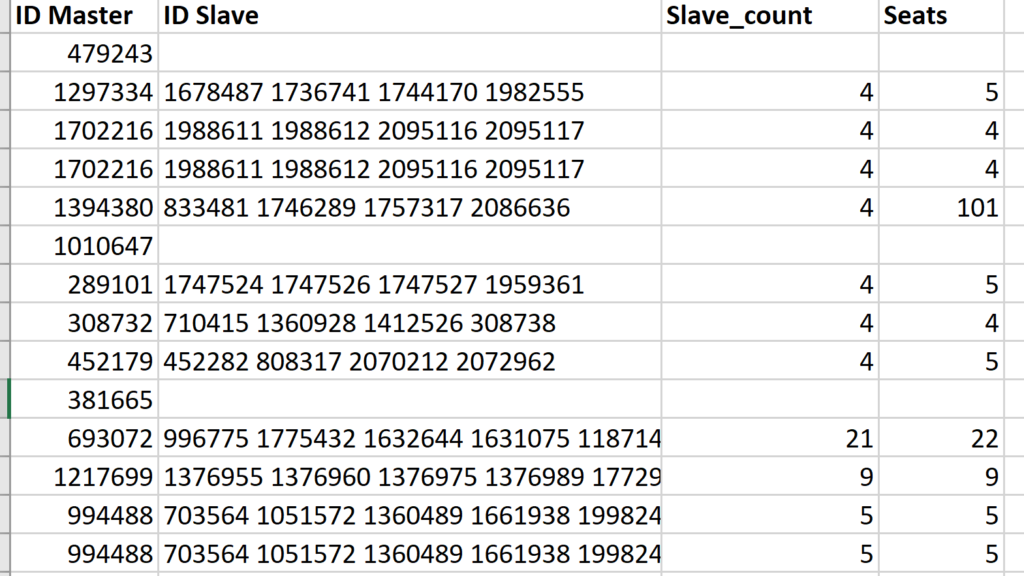
Normally, if use the functions in excel, it will take few hours to format it. But python can solve this in 2 minutes.
And here is how I write the codes to make it real.
#import module for dataframe and matrix import pandas as pd import numpy as np
#change the dataset here path = 'MaterSlave.xlsx'
#open the sheets in the excel file df = pd.read_excel(open(path, 'rb'), sheet_name='Master+Slave') df_Master_seeds = pd.read_excel(open(path, 'rb'), sheet_name='Master')
#identify the size of dataset df.shape #identify the columns df.columns
#identify the user status df = df[(df.Status == 'Master') | (df.Status == 'Slave')] df_Master = df[df.Status == 'Master'].copy()[['ID User','Seats']] df_Slave = df[df.Status == 'Slave'].copy()
#convert the datatype of "ID User" df_Slave['ID User'] = df_Slave['ID User'].astype('str') df_Master = df_Master.rename(columns={'ID User': 'ID Master'}) df_t = df_Slave.groupby('ID Master', as_index=False).agg({'Seats': 'sum', 'ID User': lambda x: ' '.join(x)})
#count how many slaves in one master account df_t['Slave_count'] = df_t['ID User'].apply(lambda x: x.count(' ')+1) #change the datatype of "ID Master" df_t['ID Master'] = df_t['ID Master'].astype('int64') #see the new dataset df_t.head()
#see the master seats df_total_seats = df_t.merge(df_Master, on = ['ID Master']) #calculate the totle seats df_total_seats['Seats'] = df_total_seats.Seats_x + df_total_seats.Seats_y df_total_seats.head()
#drop unnecessary columns for seats of different status and only leave the necessary one be in the dataset df_total_seats.drop(['Seats_x', 'Seats_y'], axis=1,inplace=True) #Rename the column name df_Master_seeds = df_Master_seeds.rename(columns={'Master ID': 'ID Master'})
#merge the sheet one and sheet two df = df_Master_seeds.merge(df_total_seats, on=['ID Master'], how='outer')
#export the dataset df.to_csv('Master_Slave_result.csv', index=True)
When you open the dataset 'Master_Slave_result.csv', you can see the result is as same as the second picture.
This join method can be used in most of the cases on marketing analytics. As long as you want to organize the information of user account, or identify the subscriber changes, this blog can help to make your life easier as an account manager/analyst.