Predicting Fraudulent Health Insurance Claims
Introduction
Healthcare fraud is a large and pervasive problem in the US healthcare system. The National Healthcare Anti-Fraud Association estimates up to $300 Billion in fraud of a total of $3.6 Trillion in insurance reimbursements throughout the US healthcare system. This fraud harms not only insurers but also individual citizens, the ratepayers for insurance companies, and the taxpayers that support the largest healthcare program in the country, Medicare. And it can take many forms.
Providers can bill for medical services that never took place and duplicate billing for services that were performed only once. A provider can โunbundleโ a claim with several diagnoses and procedures from a single medical visit into a set of claims spanning several office visits - to grab that sweet "facility fee" for each visit. Or they can upcode the severity of the diagnosis or procedure to charge the insurer more.
Because of these many forms and the size of the problem, many states require insurers to have anti-fraud detection units in addition to criminal investigations by the Federal Bureau of Investigation. However, by the False Claims Act, the insurance companies can recover their money if fraud is shown, and the Act also allows punitive charges to be imposed in civil court.
Our project was to develop a machine learning model that could help insurance companies identify potentially fraudulent providers, reducing the number of random audits required and focusing resources on providers more likely to be fraudulent while minimizing the number of investigations into innocent providers (false positives.)
Data description and feature engineering to help detect fraud
There are four parties in any healthcare transaction. An insurance company that is paying the majority of the cost. Providers (e.g. hospitals and clinics) that are the businesses requesting reimbursement from the insurer for services performed on the patient.
There are physicians who actually perform the services and who are often employees of the providers, but in some cases may also be providers if they are a solo practice. Finally, there are the patients (or beneficiaries) who receive the services and who pay premiums to insurers for coverage. They will also pay a deductible to the provider, often a fraction of the total charges for service, and determined by the patientโs insurance policy.
In our dataset there are5410 providers that were labeled as either potentially fraudulent or not, with about 10% of the providers labeled as fraudulent, making this an imbalanced classification problem. The data provided were a little over 550,000 claim records, with reimbursement, procedures and diagnostic codes and attending and operating physicians, among other information. There were also demographic and medical information records (e.g. chronic illnesses) for the patients.
We first noticed the majority of claims had patients above 65, with a sharp increase in claims after 65 years of age due to automatic enrollment in Medicare. This enrollment trend was reflected in the average number of chronic illnesses, which spiked at 65 also. We also observed that one gender (presumably women) was over-represented at ages above 85.
Network Analysis on fraud
To understand fraud networks, we must first gauge their size and extent. The below graphic shows the number of providers operating for a given number of states. The y-axis is on a log scale and looking at the count of providers operating in 1-3 states reflects the obvious. Most providers (clinics, hospitals) are local. They operate in one state or - if located on a border - might service one or two more.
However, as the given number of states a provider operates in increase, the relative proportion in the number of providers between โNoโ fraud and โYesโ fraud decreases: Fraudulent providers are overrepresented in large medicare provider operations that span several states.
Plot showing the count of providers by the number of states in which it has associated beneficiaries.
We examined network relationships between the providers, physicians, and patients, by creating an R Shiny tool for visualizing each state's provider-patient-physician networks on county levels. What we found was striking. Shown below are typical examples of a provider-patient network and a provider-physician network - in that order. The edges between each actor and a provider is weighted and sized according to the total reimbursements from all claims filed between the two. The legend is provided as well:
Actor | Shape | Color |
Provider | Square | Fraud |
No Fraud | ||
Unknown (Test) | ||
Patient / Beneficiary | Circle | |
Physician / Doctor | Triangle |
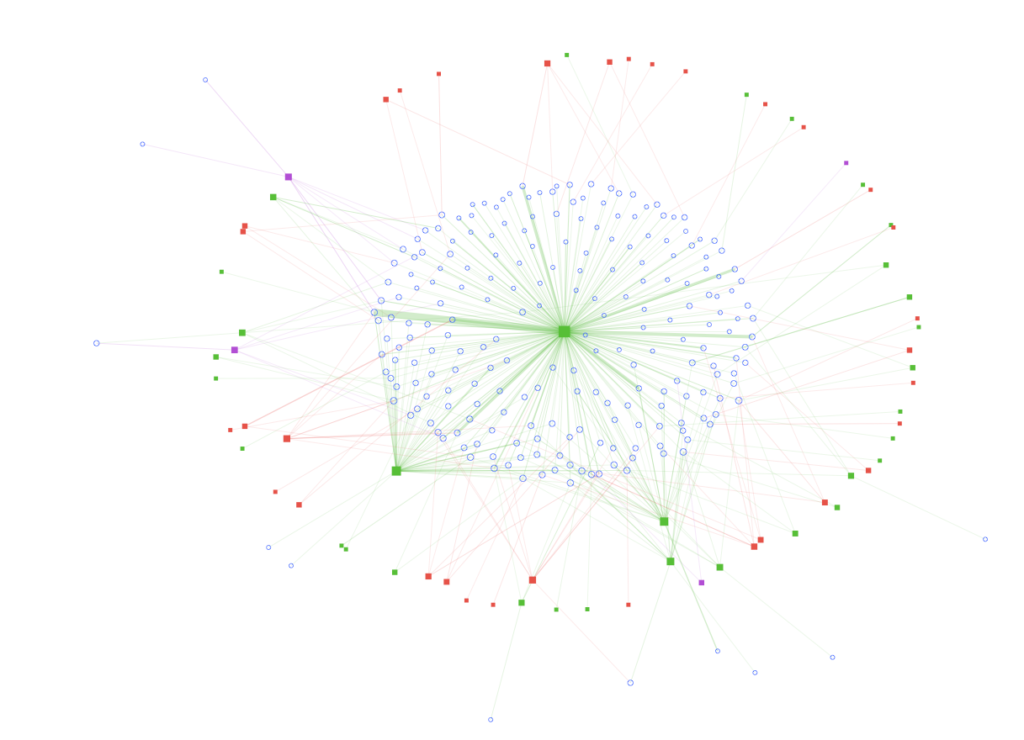
Provider-Patient Network for New Jersey, County 300
The providers in our medicare dataset - across states and counties - were much more likely to be linked to one another through shared beneficiaries than through shared physicians. This can be shown more clearly through a bipartite projection. A bipartite project takes a network with two types of actors (Provider-Physician/Patient) and projects onto a single type - establishing links through shared relationships from the full graph. Below, therefore, is a network of Providers linked together if they filed claims for the same Patient.
The above is likely a general hospital and its links to various smaller clinics. Below offers a stark contrast: A completely isolated network of providers - since there is no claim filed with the same doctor for two different providers.
Network Feature Generation
Having gained fluency in manipulating network graphs with the igraph library in R, we moved on to analyzing the graphs through various metrics. There is a veritable menagerie of centrality, connectivity, and adjacency metrics that one could consider in application to network analysis. For our case, we limited it to four metrics for each type of projected bipartite graph (Provider-Patient-Provider, Provider-Physician-Provider). The four were:
- Degree: How many other providers is Provider X connected to?
- Betweenness: On how many shortest paths between providers does Provider X lie on?
- Average Nearest Neighbor (ANN): What is the average number of nearest neighbors for all providers directly connected to Provider X?
- Eigenvector Centrality: A measure of the influence of Provider X (Google's PageRank is based on this metric).
In total then, we had eight new features that varied in their predictive strength in our models.
Duplication Networks of Fraud
As the next step in our analysis, we used networks to explore a class of fraud that's has a network structure from the start: duplication of claims. Though each claim ID is a primary key in our dataset, the provider, physician, and beneficiaries are not. Thus - especially in the outpatient data - we have a multitude of claims that seem to have been duplicated. To find these claims, we cast a wide net: we identify - as duplicate - claims with the same beneficiary having the same three diagnosis codes.
We filtered out the many all null entries which likely represented claims associated with patient visits to outpatient clinics with no diagnosis or procedure performed.
Largest duplication network found in our data - corresponds to NY-NJ-CT provider networks
Market Basket Analysis
We also performed a market-basket analysis, to determine what frequent combinations of chronic illnesses and specialties might be associated with fraudulent providers. We did find that certain sets of chronic conditions did associate with each other. For example, we found that diabetes and ischemic heart disease were strongly associated, which mirrors reality where diabetes is recognized medically as a strong risk factor for coronary artery disease (i.e. ischemic heart failure.)
We also found associations of diabetes with kidney disease, which again is observed medically due to the degradation of the vasculature by diabetes. Interestingly, we also found an association between fraudulent providers and the proportion of their patients that had diabetes and ischemic heart disease.
Connection diagram from Market Basket Analysis showing connections among patientsโ chronic illnesses
Other provider characteristics that were predictive of the likelihood of fraud were the number of days it took to resolve the claim (which closely tracked the length of stay for in-patient claims.) One characteristic from this attribute was the predictive strength of a providerโs range from a maximum to a minimum number of days that a claim lasted.
Fraudulent providers had a wider range of claim durations, with claims lasting only 1 day to claims lasting to the maximum observed (35 days for in-patient, 21 days for out-patient.) On the other hand, non-fraudulent providers were much more consistent in their claim duration.
In-patient claim duration range, with fraudulent providers in red on left
We also observed this same trend of the fraudulent providers having wider ranges in the percentage of the claim that was covered by insurance. We also observed that the average charges per claim and the average per-day charge of the claim (the total charge divided by the duration of the claim) were higher for fraudulent providers, on average.
Per-day charges average for providers, with fraudulent providers in blue on right
Cost Metrics: Model Evaluation and Selection based on Potential Fraud
If we assume that no model is perfect, we acknowledge that misclassification occurs and that each type of misclassification carries a cost. In this project, the two possible misclassifications were failing to catch a fraudulent provider (false negative) and falsely accusing a provider of fraudulence (false positive). The cost of not identifying a fraudulent provider is to let the theft of reimbursements continue and serve as an enticement for others to commit fraud.
Alternatively, the cost of falsely accusing an innocent provider of fraud is certainly reputational damage, but also extra investigative costs and possibly legal costs as the provider fights the designation. We believed this was a balancing act, and attempted to develop a model using realistic costs for investigations and legal disputes and to measure the amount of money that had been claimed by fraudulent providers by comparison.
We attempted to maximize the number of claims (as a fraction of total claim dollars) and the ratio of the amount of money identified to the investigative expenses. This penalizes both false positives since they represent extra cost and false negatives since they reduce the number of claims identified for recovery. Statistically, we optimized models based on F1, the harmonic mean of precision and sensitivity.
Types of models
We examined multiple types of models, including unsupervised learning/classification, logistic linear regression, decision tree models, and boosted decision tree models. The unsupervised classification models performed poorly, with reasonable identification of fraudulent providers but with 3 times as many falsely accused providers as fraudulent providers. We did not pursue this further. We show a summary table of the models evaluated on our cost model below:
The table tracks right in terms of model performance according to our cost metrics. The first, logistic regression with a lasso penalty had the highest recall but misclassified 12 percent of non-fraudulent providers as fraudulent - taking up the majority of our investigative resources. The second contended is the Multi-Layer Perceptron - an implementation of a simple neural network - which lowered the False Positive rate at the expense of fewer true positives.
Final Model
We found the boosted tree models gave us the best results. Logitboost - a variant of AdaBoost that maximizes the binomial log-likelihood directly - gave great performance with respect to the final profit metric. Combining it with an MLP classifier through a logical AND operation boosted the results even further - driving down the resources allocated to investigating false positives to 13 percent - and delivering a final profit metric of 5.93.
Our final model had a ratio approaching $6 of total reimbursements identified from fraudulent providers for every $1 in investigative costs.
Finally, we note that while we identified only 60-70% of the fraudulent providers, we identified about 90% of the money billed by these providers, an indicator that our model was effective at identifying providers by size, with smaller providers more likely to escape. The additional funds that could be recovered from these providers would offer diminishing returns on the further investment of investigative resources. We have bigger fish to fry.
Conclusion
We have developed a set of models that are relatively effective at the identification of fraudulent providers, with metrics that take into account economic cost/benefit that approximate real tradeoffs. These models offer an attractive return on the investigative money invested, potentially allowing the insurer to reduce premiums for its policyholders. We found through multiple measures that fraudulent providers are likely to be larger and have broader networks, though some measures such as the proportion of deductibles as a fraction of total charges might be less dependent on provider size.
Given these characteristics of the dataset and the models as they were developed, we were able to identify 90% of the claims made by fraudulent providers while maintaining reasonable investigative costs.