Recommender Systems' Data Impacting People Behaviors
The skills the author demoed here can be learned through taking Data Science with Machine Learning bootcamp with NYC Data Science Academy.
Introduction
There are many ways recommender systems impact the way people behave. For companies, they improve retention for users, increase sales of product, provide insight into future trends, and accelerate processes by efficient filtering. For Netflix, there are data sources that say 75% of what their customers stream comes from their recommendation system (McKinsey).
The general idea of recommender systems is that they provide a comprehensive list of products that have received good overall reviews or are highly favored by similar users based on user data.
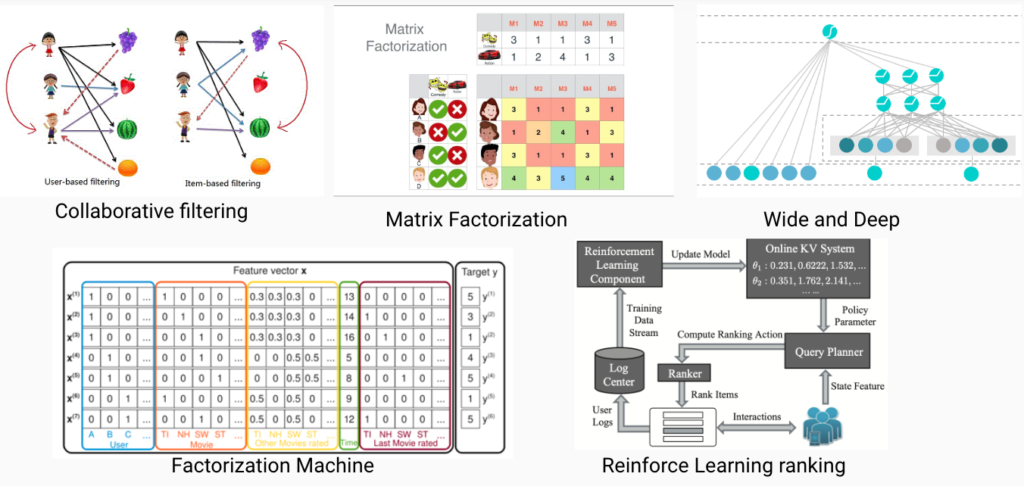
As seen in Figure 1, the trend of recommender algorithms has continued to change.
The matrix factorization model makes a user and item matrix and recommends through content-based or collaborative filtering to create a relationship that was not previously present in the data. This model was brought about and popularized by the 2006 Netflix challenge and remains fundamental to recommender systems today.
A wide and deep model utilizes a wide model for recommending the range of goods and a deep model for recommending detailed items of goods. This type of recommender was brought about in 2016 by Google.
A factorization machine is similar to matrix factorization but can encompass more features, thereby enabling the recommender system to give tailored output based off of other miscellaneous features. This method was invented in 2010 by a Google research scientist.
Recently, RNNs or reinforcement learning methods have been developing for use in personalized recommendations since 2016.
All of these various methods aim to reduce problems that recommender systems can often face. These shortcomings include popularity bias, the cold start problem, and scalability.
Objective
Yelp is a company that was founded in 2004 by two members of the "PayPal Mafia", Russel Simmons and Jeremy Stoppelman. It is a business directory forum that crowd-sources its information.
For Yelp, it indubitably makes sense that their platform is greatly dependent upon a recommender system to drive traffic along their website and generate value for businesses listed on their site. Better targeted users for businesses mean better reviews which ultimately lead to better business value. A Harvard Business School study published in 2011 found that each "star" in a Yelp rating affected the business owner's sales by 5β9 percent (Harvard).
With all of this in mind, we used a dataset that was sourced from Yelp's Open Dataset. This is a dataset that contained nearly 7 million reviews, almost 2 hundred thousand businesses, and much more.
With this project, our objective was specifically to provide restaurant recommendations to users with 10 or more reviews.
We did not want to focus on users with not enough data because it would more or less defeat the purpose of a recommender system if we could just generate businesses with the most reviews or highest ratings. However, we did encompass a base model to also recommend restaurants to users with less than 10 reviews so that any test user passed through could guarantee a list of restaurants to choose from.
Exploratory Data Analysis
Because of the size of this dataset, we took a deeper dive into each aspect of the data. For this project, we only utilized the review.json, user.json, and business.json files in order to build the recommender system.
Figure 2. Data analysis on reviews
These graphs display some basic information for reviews of the dataset. The number of cool, funny, and useful votes that reviews ended up getting came out to be very similar. However, when looking at the distribution of stars, we see that the number of 5 stars was the most prevalent followed by 4 stars then 3. This is important because if one thinks about reviews in general, theyβre subjective and goes into psychology that we could further leverage in future analyses.
Figure 3. Data analysis on restaurants
In the data for restaurants, we saw that the majority of restaurants had review counts less than 30 with a max review count of 8570, thus presented a very right skewed distribution. This is an important because the impact of a few low ratings for restaurants that arenβt as popular are huge compared to restaurants that have a lot of reviews. This discovery is meaningful when coming up with a list of recommendations for users.
Also, the distribution of the star ratings for all of the restaurants are skewed left. This is interesting because it makes you consider the restaurantβs attributes, such as size, social media presence and more.
Figure 4. Data analysis on users
These graphs show the information on users in the dataset. The average star rating distribution by user is very similar to the previously seen distribution of stars for all reviews. Other features also display a very right-skewed distribution.
In addition, the user-specific review count shows a huge right skew, even more than the distribution for review counts for each restaurant, and it was because most users wrote less than 5 reviews.
Figure 5. Review count distribution per category
As seen in Figure 5 displaying the categories present in the dataset, there were so many options that a restaurant could have as a feature. They were not labeled according to more specific encompassing groups such as βcuisineβ. Because of this diversity, there were no clear separating groups and there was sparsity once we flattened out these categories as each individual feature.
Taking a look at the matrix overall, we calculated the ratio of filled reviews (1,386,124) to the combination of user and restaurant (4,115,847,712). We found the result to be a very sparse matrix (only 0.03% filled!!), thereby making it much harder to account for personalization.
Figure 6. Filtering process of dataset
Because the Yelp dataset contained all types of businesses, we extracted all of the restaurants by looking at value counts of categories and intuition in regards to the categories within the step of EDA.
Also, by trying to segment the data further by cities, states, etc., we realized that although this dataset was indeed very big, it did not nearly give a big enough picture for the trends that could come up in different parts of the world. This was because there were a lot of missing states and cities. For example, there were no Los Angeles entries and there were only 13 New York entries.
Taking this into consideration, we had to decide how to focus this project and thus filtered our data to include the entries in the city of Las Vegas only, considering that it was the number two city in regards to the number of reviews and a US city (as opposed to a Canadian city).
Out of the total 600 categories, the number of categories related to restaurants was 177 but we condensed these further into 88 categories for our model.
Some of the restaurant's attributes, such as romantic ambience, would have been useful for learning, but we decided that it was more appropriate to filter according to the user's preferences to certain attributes after the model had ranked the restaurants because the data matrix was already too sparse. Because of a lack of time, this filtering via attributes was not implemented in this project.
Recommender System
Factorization Machine
The first model we applied was a Factorization Machine using the xlearn package. If the model learned through the use of the full dataset, the ability to learn would have been too difficult because of disparities in review counts. Therefore, we filtered for users who had written at least 10 reviews and combined the user, review, and restaurant information to pass into the model.
The field-aware FM model adds another dimension to the latent vector, which allows the model to provide more accurate results. It was used to learn categories in an additional dimension.
Deep Factorization Machine
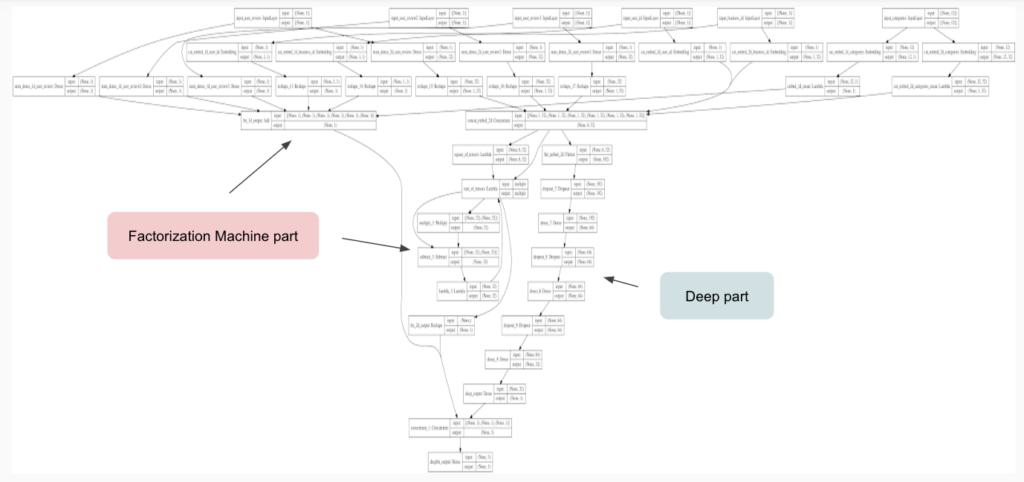
The second model we used was a Deep Factorization Machine (Figure 7). It is a model where input data is divided into an FM layer and a deep layer and the FM is applied to a wide and deep model.
If the same inputs are duplicated into different types of factorization machine models and deep neural network models, FMs can model the interaction of low order features and the DNN can model the interaction of high order features.
Matrix Factorization
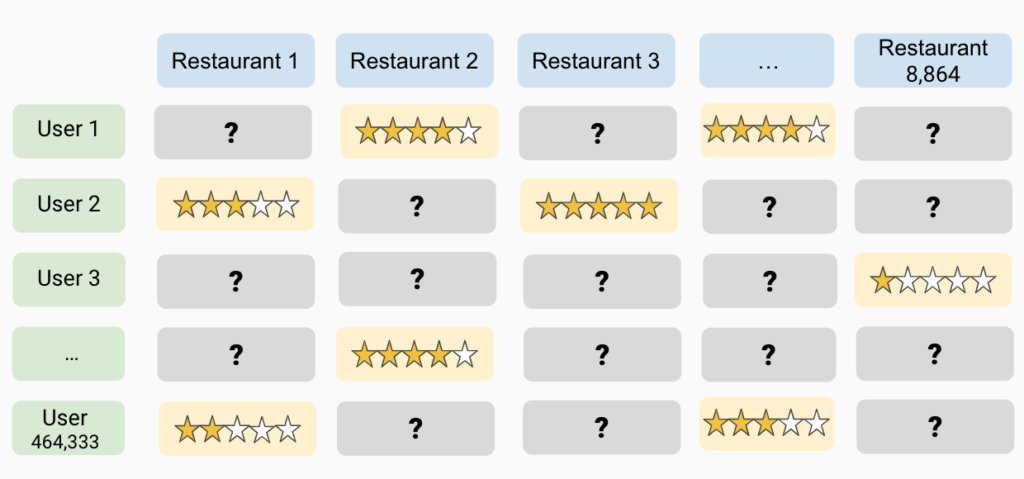
The third model utilized was matrix factorization using singular value decomposition or SVD as seen in Figure 8. This model presents user-restaurant relationships as a matrix and mathematically disassembles the matrix to predict the empty customer's stars. This is implemented using an easy-to-use package called surprise.
Surprise is a Python scikit for recommender systems. It provides various ready-to-use prediction algorithms such as baseline algorithms, neighborhood methods, matrix factorization-based models, and many others.
We used different methods of MF to train the model and measured the RMSE values. Performance was compared between SVD, baseline only, and SVDPP. From these, the SVD algorithm was finally selected to predict the user's restaurant-specific rating.
Data Results
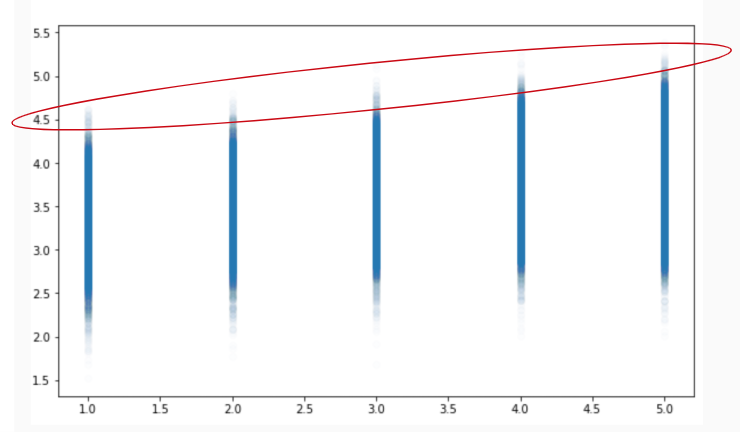
The learning outcomes for all three models were similar. The MF model with Surprise scored slightly higher, but overall the figure was similar. By looking at Figure 9, we see the higher the stars in the restaurant, the higher the predicted scores.
We used the same dataset for all three models, but we applied a 10 or more review count filter to the DeepFM and factorization machine models. In contrast, we supplied the entire dataset to the matrix factorization model. Each score was normalized, then ensembled with each weight to make a final ranking. Data excluded from the DeepFM and factorization machine were taken into consideration by weighting the results of matrix factorization.
Flask App
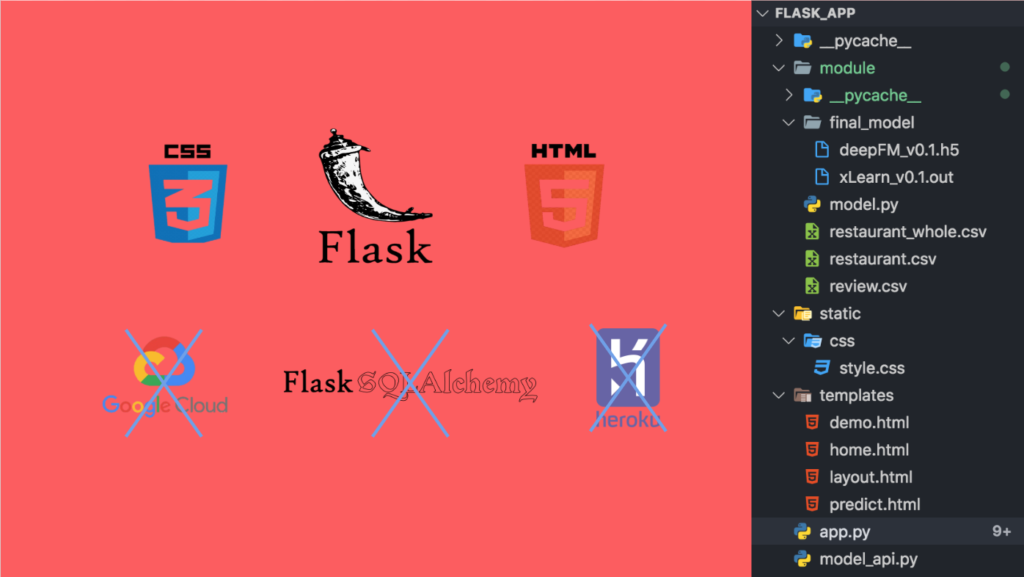
We built and deployed a Flask web application for our demo of this recommender system. Because of a lack of time, we couldnβt upload our data into a database and use Flask SQLAlchemy to send queries in order to output resulting recommendations.
Instead, we first saved our trained model then used it within our app.py file in order to pass in test cases with users already in the dataset. For the front end, we used CSS stylesheets and HTML templates for each of the pages in our app.
The figures shown below are screenshots of our Flask app. For our demo, because of a lack of interpretability when analyzing results of the initial ensembled model we had made, we had to make a new model with all of the weight only applied to categories of restaurants. Through this, there was a small addition of interpretability when explaining how the recommender system outputted its results.
Once again, due to a lack of time, we were not able to deploy our app using services like Google Cloud or Heroku but instead had to settle for our app being deployed on a local server. Because we were fetching results with input data using our local file system, the program took around 6 seconds to output a list of recommendations for a test user.
Conclusion
Recommender systems are so hard to test. Typically, to test in daily operations, companies cannot ask users how the recommendations are perceived so they look through the clickthrough rate of each recommendation. We could only look at the numbers of our results but not much more.
Thereβs a clear concern about data leakage because the test users in our model are users who were present when training the models initially. However, in regards to this, we wanted to build a comprehensive model that took into account many factors holistically rather than merely filtering and spitting out best rated or most popular restaurants. In this project, we targeted specifically recommending restaurants for users that had data already.
Rather than manipulating the data in our local environment, we realized that it would have been more conducive to utilize the cloud or a distributed environment. Doing this would have prevented delays in the processing or storing of our data using our local system, increasing efficiency greatly. Therefore, we realized how important it is to consider the environment in which we work.
Our demo's output of results was not interpretable using our ensembled model. Therefore, just as other companies such as Netflix does, we should train separate models depending on each feature such as categories, reviews, etc. Otherwise, no matter how good the results may be, it is not explainable in any way. Although this doesnβt matter as long as the recommendations drive business value, we realized that this is very important to consider from a data scientistβs perspective, especially in a setting where there is no easy way to test our results.