YouTube Sentiment Analysis for 2018 Oscar Nominee Trailers
Project GitHub | LinkedIn: Niki Moritz Hao-Wei Matthew Oren
The skills we demoed here can be learned in NYC Data Science Academy's Data Science with Machine Learning bootcamp.
Overview
My Shiny project is on sentiment analysis on YouTube comments on movie trailers of Oscar Best Picture Nominees in 2018. Sentiment Analysis is a commonly used data analytic practice to trace how customers are feeling about certain products by analyzing their choice of words.
Through the analysis of Youtube comments, I could find several interesting correlations between popularity and Oscar nomination. In order to analyze movie fans' comment, I conducted multiple data experiments that included: simple numerical EDA, sentiment analysis, word cloud, and bigrams. As I walk you through my project, I will explain in more detail what each observations demonstrated about these movies.
Data
This dataset was provided by Promptcloud in Kaggle to study YouTube comments posted for the Oscar-nominated movie trailers. Datasets have all the comments posted till 6th March 2018 that include timestamps, comment_texts, likes, has_replies, and number_of_replies. Through observation of the dataset, I was able to analyze popular sentiments on movies from multiple angles, including comment counts, frequently used words, transition in popular sentiments according to a timeline, etc.
Observations & Analyses
In order to find out the direct correlation between the number of comments and Oscar nominations, I started with a simple Exploratory Data Analysis.
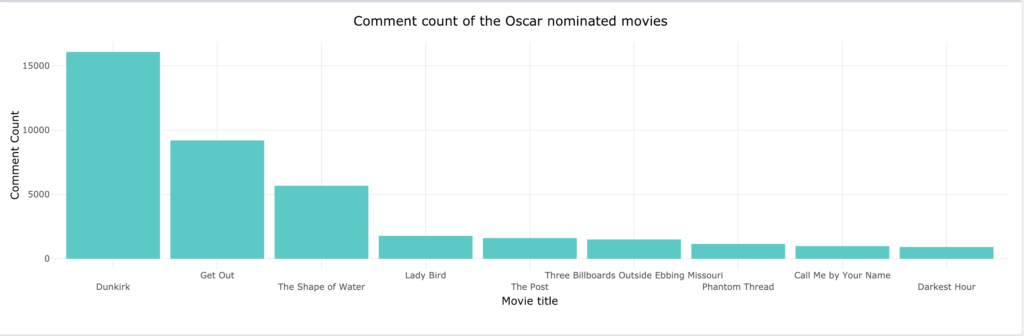
Comment count of each movie trailers reveals an interesting relationship between popularity and Oscar nominations. Dunkirk evidently has the highest count of comments by 16,059, got 8 nominations and won three (Best Sound Mixing, Best Film Editing, Best Sound Editing). Get Out got 4 nominations and won one(Best Original Screenplay). However, the actual winner of the Oscar Best Pictures award, The Shape of Water, dominated with 13 nominations and won four (Best Picture, Best Original Music Score, Best Director, Best Production Design).
With the premise that the comment count represents the popularity of the movies, it is quite clear that the popularity of the movies and actual nominations and winning of the Oscar have a certain degree of correlations between them. However, we can also see that The Shape of Water, although it ranked third in the comment counts, was nominated for the biggest number of Oscars. In other words, the popularity of each movie and the number of Oscar Nominations/Winning it get is not directly correlated.
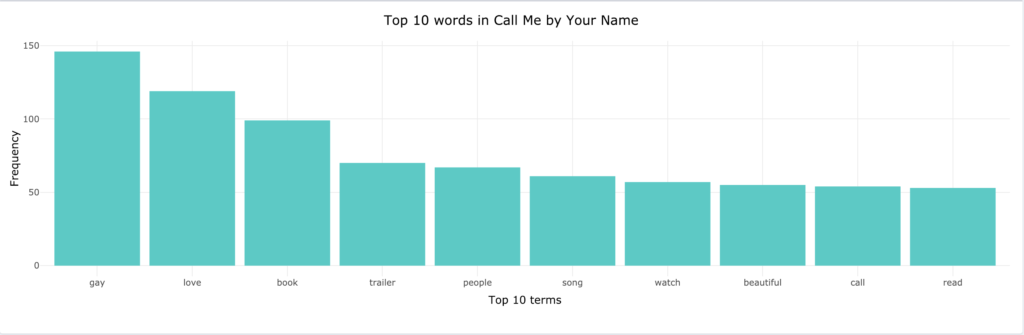
After looking into comment counts, I analyzed the top 10 words used in each of the movie trailer comments. Although it was not the case that these words communicate the correlations between popularity and the Oscar nomination of the movies, the top 10 words used in comments communicated key information about the movies. From these visualized data, we can deduce that Call Me by Your Name is a movie about homosexual love, and it was adapted from a novel.
In fact, the movie was nominated for and won the Best Adapted Screenplay award in the Oscar 2018. For Phantom Thread, we can see that the top three words used in the comments are Daniel, Day, and Lewis, which adds up to the name of the leading actor. Considering the fact that Daniel Day-Lewis claimed that it will be the last movie he will ever film, we can infer that many of Daniel's fans left comments. In a nutshell, we see that visualized data of the top words used in comments communicate important information about the movie.
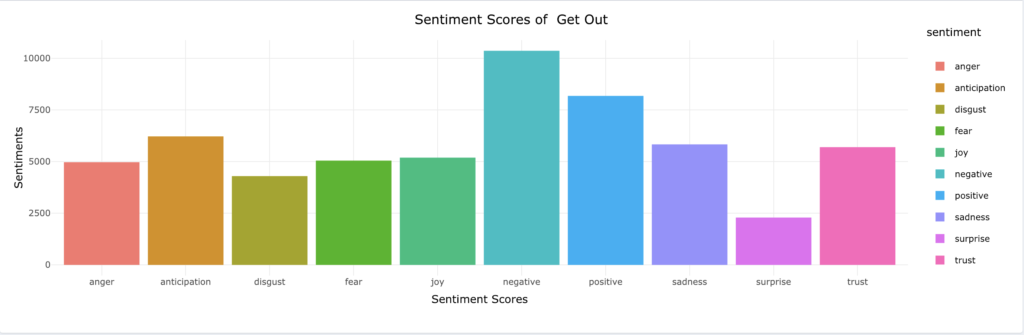
After analyzing data numerically, I conducted a sentiment analysis using lexicon "nrc." Such sentiment analysis demonstrates that the number of nominations in Oscar is not directly correlated with the positive sentiments within the YouTube trailer comments. Although Shape of Water had 13 Oscar nominations and won 4 including the Best Picture, it had 2850 negative comments following 3527 positive ones.
On the other hand, negative sentiments scored the highest in Get Out, whch had the second biggest comment counts of all Oscar Best Picture nominees and had 4 nominations. As I looked into the nrc sentiment analysis, it was obvious that neither popularity nor the number of Oscar nominations had direct correlations with the sentiments shown in the comments of the movie.
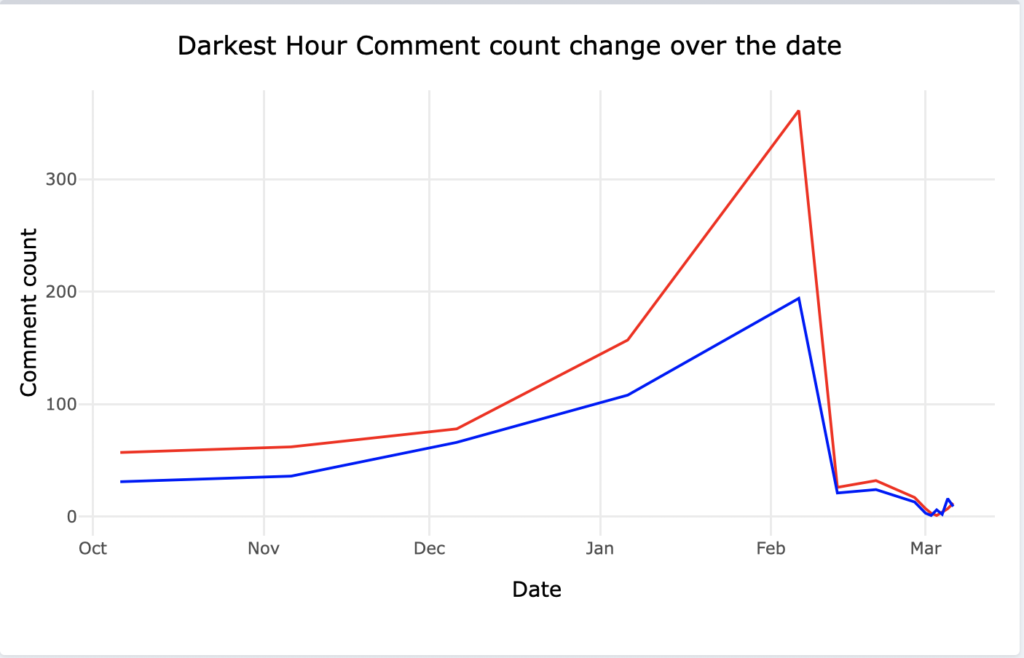
As I analyzed the data with the numbers of comments to analyze the data, I looked into the sentiments of the comments changed over time. After getting 3 nominations including the Best Picture in January 23rd, the gap between the positive and negative comments in the trailer of Call me by Your Name widened. Since there was a number of negative comments about homosexuality, we can deduce that the nominations of Oscar awards had a positive impact on people who had negative sentiments toward the movie.
Such change in sentiments shows how nominations in Oscar awards have an influence over peopleโs sentiments toward the content of the movies.
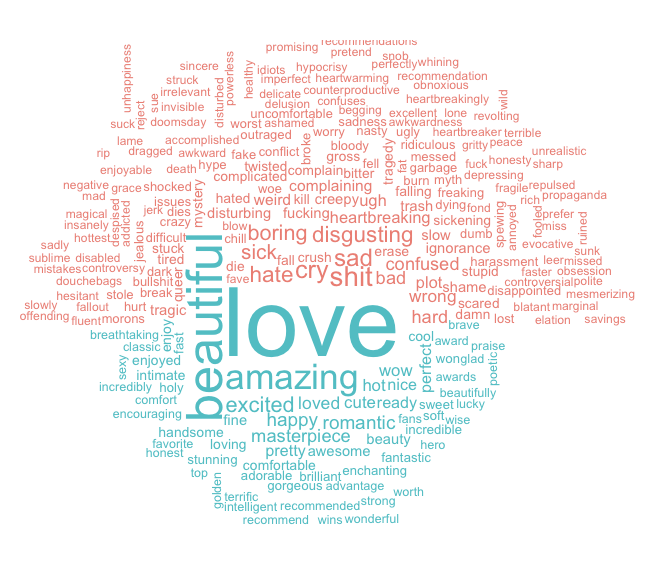
Word Clouds demonstrated the actual positive and negative words that were used within the trailer comments. Such technique clarified the mystery behind the numbers that I analyzed. For instance, word cloud of Call me by Your Name shows how peopleโs feelings are bifurcated on homosexuality. Some people deemed the movie as a beautiful recollection of love, while others thought it was a disturbing demonstration of the social minority.
On the other hand, some of the moviesโ word clouds revealed the connection of the movies with the ongoing social phenomena. The word cloud of The Post shows how people linked the movie about true journalism with Donald Trump. The comments included such words as fake, propaganda, and Trump. Ironically, Trump was interpreted as the verb โto trump,โ which made it count as a positive word.
Finally, I expanded the scope of analysis by using bigram. Bigram is the lowest number of n-gram, which is the sentiment analysis technique which looks into n number of words that were mentioned in a sequential manner. In this project, I used bigram to look into two words that came up together frequently to get more information about the word frequency within the YouTube comments.
Bigram of The Shape of Water shows that โApe Sapienโ is one of the most frequently linked two words. Ape Sapien is a fictional character in Hellboy that looks like the main charcter of Shape of Water. Consequently, many movie fans thought Shape of Water was the sequel of Hellboy,and they talked about Ape Sapien in the comments of the movie trailer.
Conclusion & Future Work
Throughout the sentiment analysis of Oscar 2018 nominee trailer YouTube comments, I could observe that the number of comments of trailers demonstrated the general popularity of the movies, but and roughly the number of Oscar nominations. In addition, the top 10 words used in comments and word clouds points out the relevant information about the corresponding movies.
Finally, analysis over time demonstrated the relevance between sentiments of comment and the Oscar nominations and bigram provided deeper insight into what audience expect from the movies that other analyses could not. Such observations offered a better understanding of the relationship between YouTube trailer comments and popularity/Oscar nominations of the movies.
In the future, I would like to investigate more into which comments got the most likes and visualize the correlation between comment counts and Oscar nominations. In addition, I would like to look into the sentiments of other Oscar 2018 nominees that were not nominated for the Best Picture and find if the ones that were nominated to the Best Picture had any specific traits.
Overall, it was an interesting project that taught me what kind of questions I want to ask when I look at a dataset as a data scientist.