Analysis of TripAdvisor Review Analysis: MGM Grand Hotel and Casino
Introduction
Data shows that customer reviews are some of the most useful tools that a consumer can use to gauge the quality of a product or service, and more specifically the overall customer satisfaction. To purchase a product or use a service without checking reviews is like playing Russian roulette with your wallet and your sanity (I learned this the hard way!).
My motivation for choosing this topic is two-fold: (i) I recently had a not-so-pleasant experience with Airbnb partly due to the fact that I did not read enough reviews, and (ii) my friends and I would like to visit the MGM Grand Hotel and Casino when I complete this Data Science program at the end of September.
The MGM Garden Arena is well known for hosting marquee events such as world championship boxing and music concerts by top performing artists, and that is one of the many experiences that my friends and I would like to have. It would be nearly impossible to read the thousands of reviews on the MGM Garden Arena that you can find on the web.
I intend to limit my focus on the reviews submitted at one popular website: TripAdvisor.com. To provide a comprehensive perspective on the overall customer experience, the focal point of my analysis will assess customer sentiments (i.e. the reviews) as well as identifying other relevant factors such as the peak and low occupancy periods of a given year.
No single review (or even a few reviews) can sufficiently capture the customer's experiences at a hotel. Thus the objective of this exercise to provide a majority sentiment of the MGM Garden Arena based on recent and past data so that a consumer may use it to make an informed decision on a future visit.
Web Scraping Approach
The data was scraped from the MGM Grand Hotel and Casino page on TripAdvisor.com (link here: https://www.tripadvisor.com/Hotel_Review-g45963-d91891-Reviews-MGM_Grand_Hotel_and_Casino-Las_Vegas_Nevada.html). The following data was scraped: (i) customer review title; (ii) customer review comments; (ii) date of stay; (iv) overall rating on a scale of 1 to 5 (1 is terrible, 2 is poor, 3 is average, 4 is good, and 5 is excellent). Figure 1 below is representative of configuration containing all the data that was scraped.
A total of 14,268 reviews spanning the period from September 2014 to July 2019 were scraped. A python script based on the selenium web driver was employed. The script and data are publicly available on GitHub (https://github.com/rattafynn/WEB_SCRAPING_PROJECT). Python and R tools were employed to analyze the data.
Results and Discussions
I. Quantitative Analysis of Reviews: Figure 2 depicts the percent distribution of all the review ratings. Per the figure, the 5-star and 4-star ratings comprise approximately 35% and 25% respectively; the 3-star rating comprise approximately 18%. Thus average (3-star) to excellent (5-star) customer experience constitute 78% of all the reviews, implying that the overall customer experience is above average (the mean rating is 3.6 stars).
Figure 3 depicts the distributions of all the reviews over all twelve months. In addition, the bar for each month is stacked with the distribution of the reviews corresponding to that month. Since the dates correspond to the date of stay, it is immediately apparent that the MGM Grand attracted the most visitors in the month of July over the 2014-2019 period. This is followed by the August-October months. This information is important to visitors who, for example, do not like crowds and want to avoid visiting when the total visitor population is high.
An examination of the variations in the ratings for each month in Figure 3 indicates that the 4-star and 5-star ratings constitute more than 50% of the ratings for a given month (in the overall case in Figure 2, the 4-star and 5-star rating constituted 60% of all the ratings). The variations in the 1-3 star ratings appears to be fairly uniform across the various months.
To further investigate if there are significant differences in rating distributions for each month, we examined the case of July and February in Figure 4; these are the months with the most and least visitors respectively. The figure shows that the ratings distributions for July and February are fairly consistent with each other. In addition, they are both consistent with Figure 2. Thus, the global distribution of reviews in Figure 2 is a good representation of the reviews for each month.
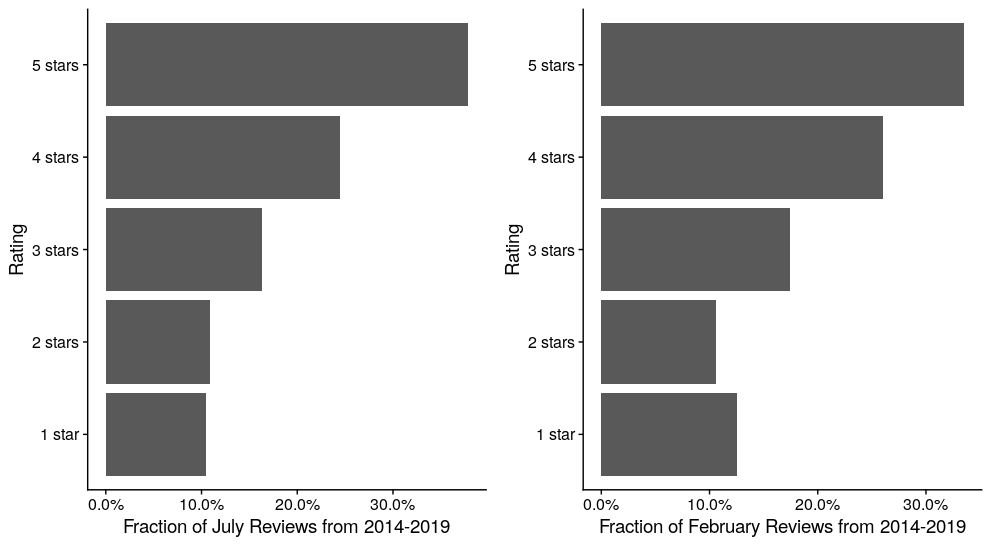
Figure 4: A side by side comparison of the ratings distributions for July (month with the most visitors) versus that of February (month with the least visitors).
II. Text Analysis of Reviews
The analysis of the words in the reviews was based on a N-gram analysis, which essentially is a frequency analysis of a continuous string of N words in a sentence or phrase: a single word analysis is a unigram analysis; a two-word analysis is a bigram analysis, and a three-word analysis is trigram analysis. The number of times a word or a string of words occur in a text is known as the term frequency (or frequency for short). Obviously the analysis can be carried out for an arbitrary value of N.
Here the focus will be on the trigram analysis of the words in the customer reviews because they best reveal customer sentiments. To further qualify the text, the trigram analysis was performed per each rating. More specifically, trigram analysis for 1-star and 5-star ratings are presented here. Figure 5 depicts the trigram frequency plot and associated word cloud for all 1-star rating.
As expected, a fair amount customers who gave this rating vowed that they "will never stay" at MGM hotel again. They also expressed their displeasure with the front desk. Other minor complaints included hot water shower issues and room smell.
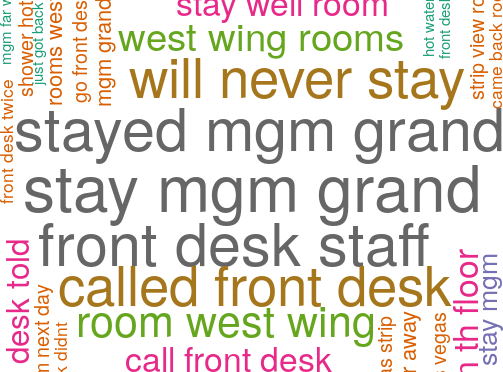
Figure 5: Top: trigram frequency analysis of 1-star customer rating comments. Bottom: corresponding word cloud of the trigrams in the top figure.
Figure 6 depicts the trigram frequency plot and associated word cloud for all 5-star rating. The 5-star rating was the dominant rating (see Figure 1) and this can be seen in the overly positive expressions in the trigrams. Customers characterized the hotel staff as helpful and friendly; they also characterized the hotel as a great place to stay. These trigrams were similarly seen in the 4-star rating analysis.
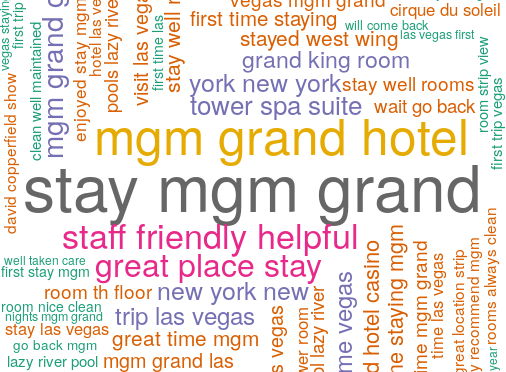
Figure 6: Top: trigram frequency analysis of 5-star customer rating comments. Bottom: corresponding word cloud of the trigrams in the top figure.
Conclusion
MGM Grand Hotel and Casino attracts the most visitors in July-October (peak visitation is in July) and the least number of visitors in November-February (the least number of visitations occurs in February). The 4-star and 5-star reviews dominate all the reviews (they constitute about 60%) of the reviews. As expected, customers who gave 5-star ratings seemed very pleased with their experience.
Customers who gave 1-star ratings had a fair amount of complaints ranging from the front desk staff to hot shower baths. Overall, the analyses suggest that visitors to the MGM Grand Hotel and Casino expressed an above-average or fairly positive experience.
Future Work
This exercise will be improved in the near future as follows:
(i) Data collection will be expanded to include several hotels and casinos along the Las Vegas strip so as to compare and contrast a much broader customer experience on a much broader scale.
(ii) A thorough customer sentiment analysis of the data in (i) above will be carried out in addition to what was presented here; this includes the level of polarity (specifically overly positive, positive, neutral, negative, and overly negative) and emotional analysis which aims to identify emotions in the expressions used by customers.