Using Data to Identify Medicare Fraud using machine learning
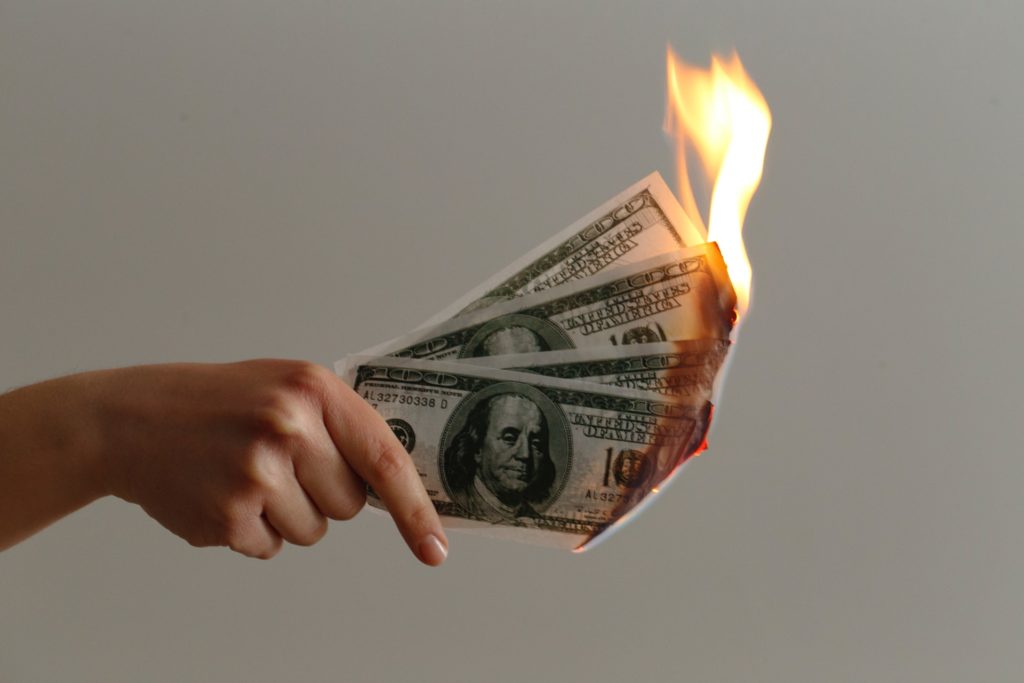
Data Overview
Data tells us that losses due to Medicare fraud in the United States are estimated to inflate public health expenditures from between 3 and 10%. This means that up to $300 billion is misappropriated from Medicare patients into the hands of criminals on an annual basis. Despite extensive efforts from state and federal law enforcement, only a fraction of Medicare fraud is thought to be identified and further investigated to lead to convictions.
The difficulty in identifying health care fraud is due to the range of deceptive methods used and the difficulty to distinguish legitimate from fraudulent claims. This has lead to a lucrative market for nefarious parties and has garnered the participation of international rings of criminals to partake.
Machine learning is an important tool that is increasingly used to identify providers involved in fraudulent transactions. This project uses supervised machine learning for the identification of fraudulent Medicare inpatient service providers and is a step forward towards stopping swindlers.
The data used for this project are from Medicare claims from 2009 and can be found on Kaggle.
The code is available on GitHub
Connect with me on LinkedIn
Types of Medicare Fraud
- Duplicate Claims
- Kickbacks
- Billing for Services not Rendered
- Upcoding of Services
- Unbundling
- Excessive or Unnecessary Services
Data
The data is in four parts:
- Beneficiary data contains information about the patients, encoded for gender, race, age, state, county, chronic conditions, etc. The data encoding key is available in the Center for Medicare Services Record Data Dictionary 2009.
- Claims data contains a listing of inpatient or outpatient treatments, with information regarding the beneficiary, the provider, the ICD codes (admit, group, diagnostic and procedure), the reimbursement amount for the treatment, the deductible amount, etc.
- Potentially fraudulent provider data contains a list of all the providers and whether they are potentially fraudulent or not. It is important to note that the dataset does not provide any specific information about what the "potentially fraudulent" indication signifies, ie. suspected, under investigation, charged, convicted, etc.
EDA: Beneficiary-focused
The Medicare beneficiary dataset contains a predominantly over-65 patient population with approximately 80% suffering from two or more comorbitities (Figure 1). Medicare also serves patients of all ages with serious chronic illnesses, thus explaining the quantity of younger beneficiaries.
Additionally, looking at the frequency with which each chronic condition was suffered by the beneficiaries, the trend followed the most common ailments in the US that lead to morbidity and mortality, with ischemic heart disease, diabetes and heart failure being the most common illnesses (Figure 2).
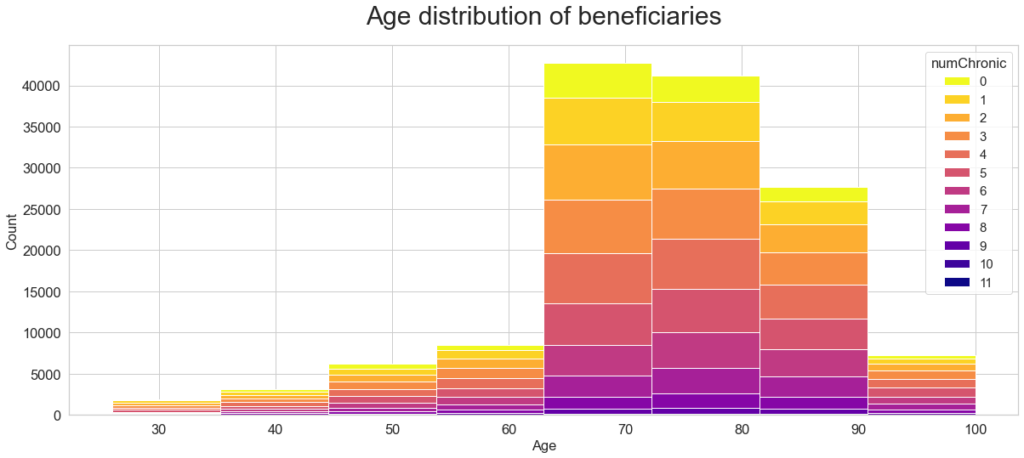
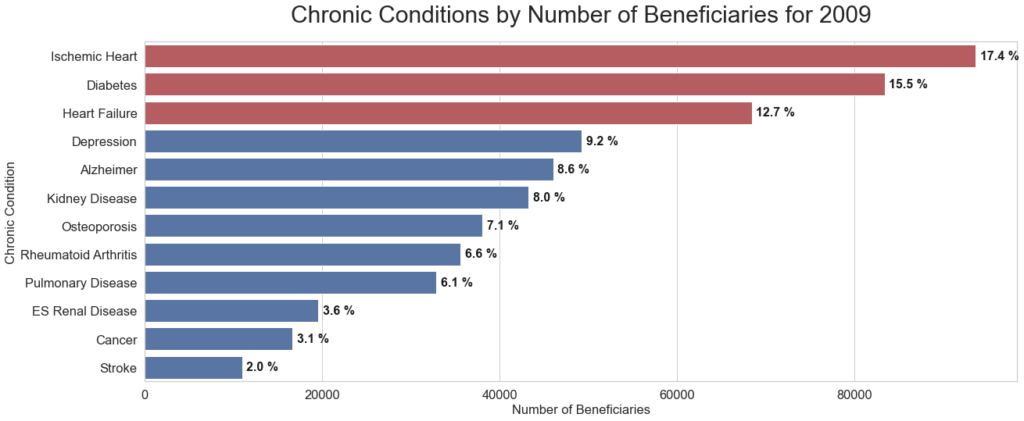
The percentage of beneficiaries suffering from each condition is indicated.
The relationship was investigated between reimbursement quantity and the number and type of chronic conditions. The box plot in Figure 3 shows that for the inpatient claims, the reimbursement amounts rose dramatically with an increased number of chronic conditions. However, this increasing trend was much more subtle for inpatient claims.
Moreover, the mean overall reimbursement amount approached $11,000 for inpatient claims whereas for outpatient claims the mean was approximately $300. This demonstrates that having multiple comorbidities resulted in an increasingly large outlay in medical care and expenditures, especially for inpatient treatments.
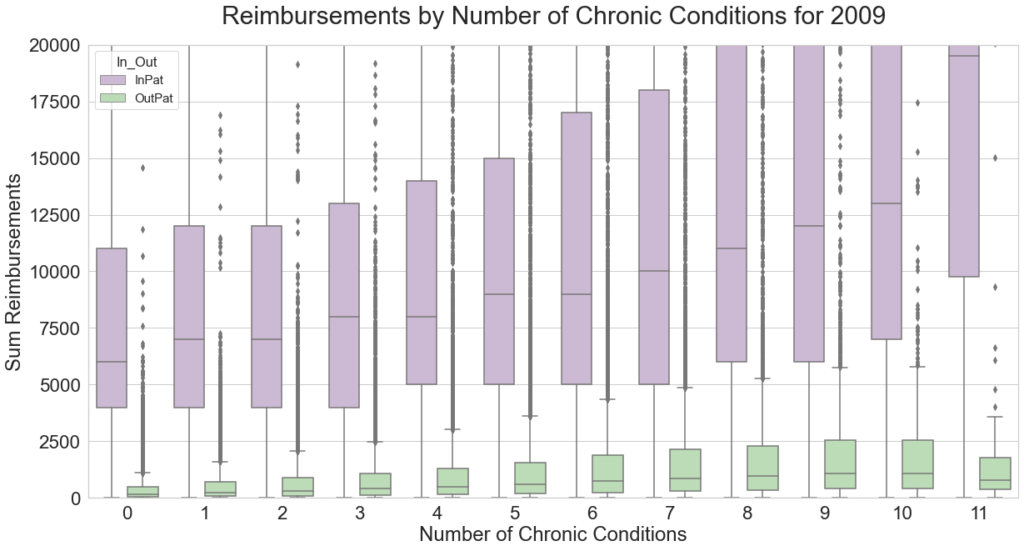
Abbreviations: InPat, inpatient claims, OutPat, outpatient claims.
The relationship between the reimbursement amount and each chronic condition is demonstrated in Figure 4. Overall, the range of the sum of inpatient reimbursements for year 2009 was similar for many of the conditions, with the median values ranging from between approximately $5000-10000 and the means ranging between approximately $5000-14000. Kidney disease proved to be the most expensive condition on average, while end stage renal disease (ESRD) was the least costly in terms of total reimbursements.
It is interesting that these two extremes in expenditures both concern serious kidney ailments. One explanation could be that patients indicated as suffering from kidney disease are more likely to undergo kidney transplant operations (a costly procedure) whereas patients indicated with ESRD are undergoing dialysis on a permanent basis (likely less costly than a transplant). In contrast, the sum of reimbursements for outpatient procedures did not show substantial variance across the different conditions.
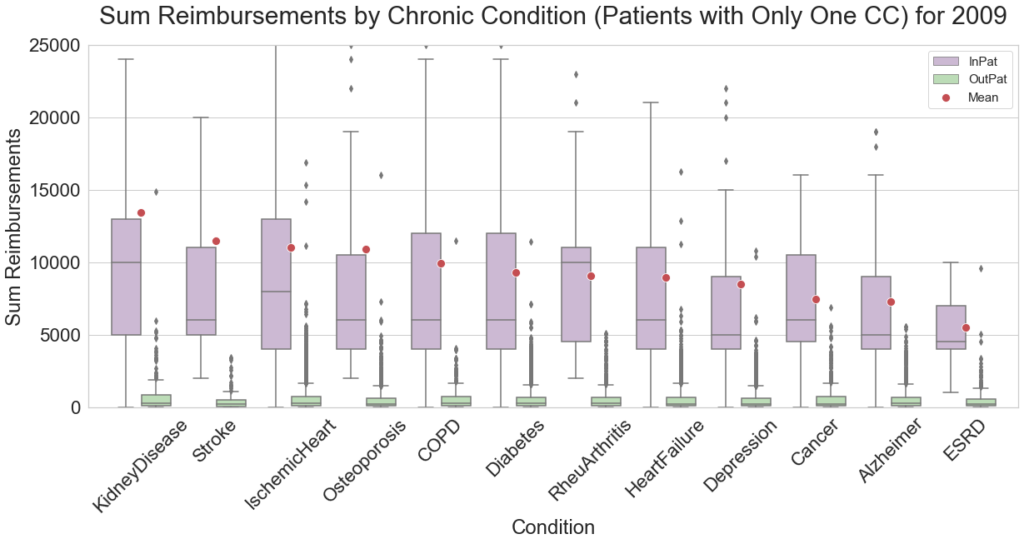
The mean inpatient reimbursement amount for each chronic condition is indicated. Abbreviations: InPat, inpatient claims; OutPat, outpatient claims; COPD, chronic obstructive pulmonary disease; ESRD, end stage renal disease.
EDA: Provider-focused (inpatient claims only)
I determined to focus on the inpatient claims and potential fraud for associated providers for the following reasons. The first is that the overall magnitude of the reimbursements of the inpatient claims was several fold higher than for the outpatient claims (Table 1). Thus, any predictive machine learning model would be tuned to the higher reimbursement amount of the inpatient claims and would be more impactful as far as predicting a higher overall dollar amount of fraud.
Second, the difference in the minority and majority classes (potential fraud vs. not fraud) for the inpatient data was smaller (21% potential fraud) than that of the outpatient data (9% potential fraud). Thus providing a more balanced dataset for the application of predictive modeling algorithms.
Total reimbursements |
|
INPATIENT |
40,474 claims totaling $408,297,020 |
OUTPATIENT |
517,737 claims totaling $148,246,120 |
Total potentially fraudulent providers |
|
INPATIENT |
440 of 2092 providers 21% of providers are potentially fraudulent Slightly imbalanced dataset |
OUTPATIENT |
462 of 5012 providers 9% of providers are potentially fraudulent Imbalanced dataset |
Table 1. Differences between the inpatient and outpatient claims datasets.
A wide variety of exploratory data analyses were carried out with the provider-grouped data. This required a three-way merge of the beneficiary, inpatient claims and provider fraud data followed by grouping the data by provider (2092 providers total) and calculating various summary statistics. Focusing on just a few analyses relevant to the modeling below, the average reimbursement or annual sum of reimbursements were visualized for the two classes (Figures 5, 6).
It was clear that the distribution for both was distinct between potential fraudulent and not fraudulent providers, in that potentially fraudulent providers tended to receive higher reimbursement amounts. Additionally, the length of inpatient stay was compared between the two classes as unnecessarily extending the inpatient stay length may be a fraud strategy employed by unscrupulous providers (Figure 7).
Similar to the analysis of reimbursement amounts, there appeared to be a slight average increase in length of admit days for the potentially fraudulent providers in addition to there being a difference in the variance of the data. These differences were further supported by testing for equal means by T-test and equal variances by Leveneโs test (Table 2).
Comparing the potential fraud and not fraud classes failed testing for equal means and equal variances in all cases, suggesting that there are statistically significant differences between the two classes. Therefore, reimbursement amounts and admit length may be distinguishing features with predictive power for modeling.
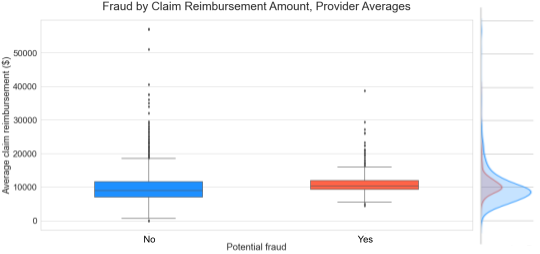
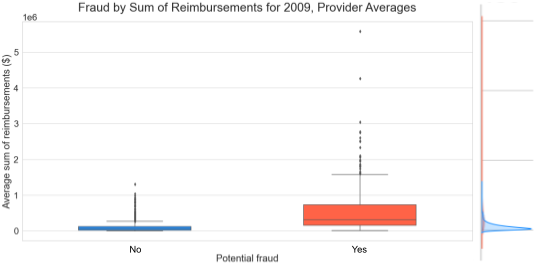
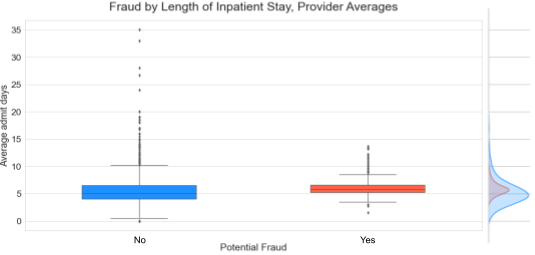
Not fraud vs. fraud class comparison | p-values | |
T-test | Levene's test | |
Average reimbursement | 1.2x10-4 | 7.3x10-9 |
Average annual sum of reimbursements | 1.7x10-138 | 4.1x10-95 |
Average length of inpatient stay | 1.2x10-4 | 1.8x10-13 |
Table 2. Statistical analysis comparing not fraud and potentially fraudulent provider classes.
Supervised Machine Learning
Provided with the EDA results demonstrating that differences exist in the features for potentially fraudulent versus non-fraudulent providers, a supervised machine learning approach was pursued as a means to develop a predictive model for fraud. The schema outlined in Table 3 was used to create the model.
DATA PREPARATION | 1. | Pre-processing |
2. | Feature engineering | |
MODELING | 3. | Establish important scoring metrics |
4. | Model selection | |
5. | Assess need for data scaling and class balancing | |
6. | Feature importance | |
7. | Hyperparameter tuning |
Table 3. Supervised machine learning model schema
1. Data preparation: Pre-processing
The three-way merged data for the inpatient claims was cleaned and simplified.
2. Data preparation: Feature engineering
Extensive feature engineering was carried out on the three-way merged data. In summary, 4 features were either dropped, or converted and then dropped (eg. features with identical values in all rows, DOB converted to age and dropped), 8 new beneficiary-based features were created (claim duration , admit days, DOD to binary, sum of number of diagnostic or procedure codes, sum of number of physicians, reimbursement amount per day, number chronic illnesses).
27 new provider-based features were created (eg. number of unique beneficiaries per provider, number of unique claims per provider, sum of reimbursements per provider, number of unique admit, group, diagnostic, procedure codes used by provider). Moreover, summary statistics, including mean and mean absolute deviation (MAD) were calculated for each provider for a number of features. This resulted in a dataset containing 51 features, with all continuous data. The feature engineering is summarized in Figure 8.
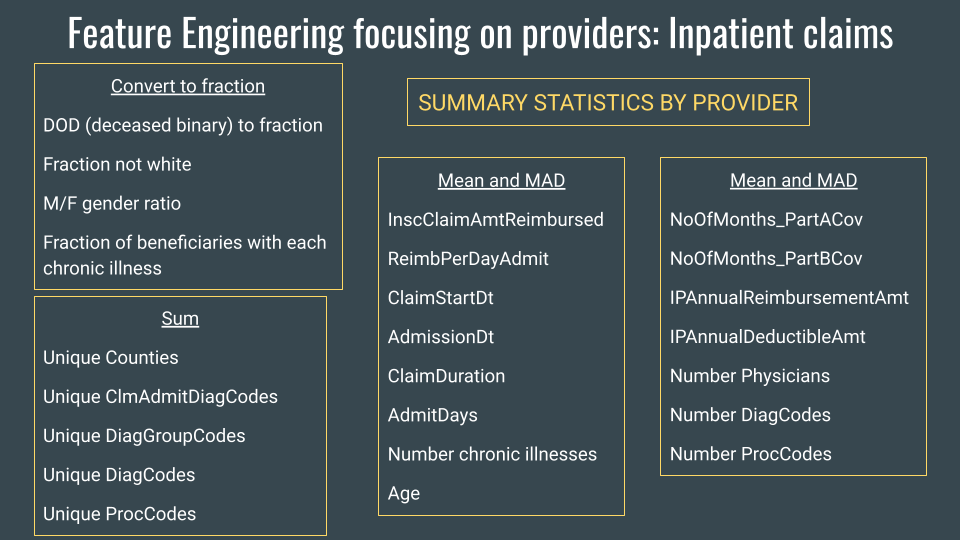
3. Modeling: Establish important scoring metrics
A number of different metrics can be used to score classification models, with two important metrics being precision and recall (Figure 9). In order to understand what model scoring metrics would be important for this case, values were obtained to estimate the relative cost or benefit of the false positive (FP), true positive (TP) and false negative (FN) classes. These values were approximated from the average Medicare fraud case investigation costs published in the US Office of Inspector General, Health Care Fraud and Abuse Control program report for fiscal year 2009.
Estimates for the per case cost of FPs or TNs and the benefit of TP settlements are presented in Figure 10. Given this analysis, FNs were found to be roughly twice as costly as FPs. Therefore, recall score was chosen as the scoring metric as this selects for minimizing the number of predictions in the FN class (Figure 9).
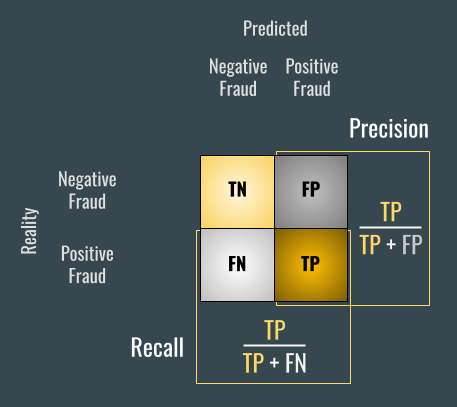
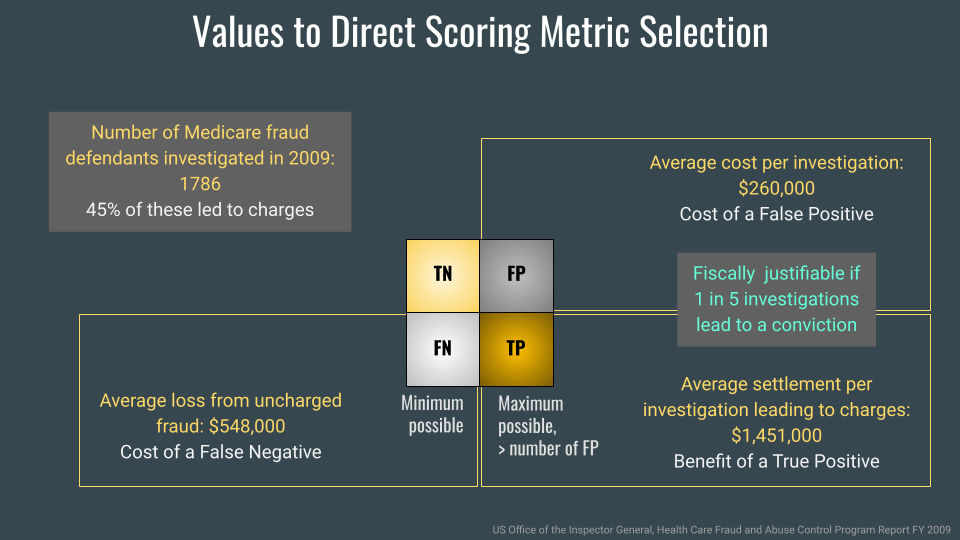
Approximate calculations were made for an average FP (investigations that did not lead to criminal charges) and average TP settlement amounts using Medicare fraud investigation data from the US Office of Inspector General, Health Care Fraud and Abuse Control program report from fiscal year 2009.
4. Modeling: Model selection
Classification model selection was carried out by screening 14 baseline algorithms (a broad mix of linear, nonlinear and ensemble methods) with scoring for recall. The highest recall score was obtained using a gradient boosting classifier model.
5. Modeling: Assess need for data scaling and class balancing
The gradient boosting classifier was further tested on the dataset that had undergone various scaling (standard, min/max) and/or class balancing (over/undersampling, SMOTE, etc.) methods. The best results were obtained with data balanced by undersampling (as assessed by highest recall score). Data scaling had no effect on the model metrics. The scoring metrics for the resulting model are presented in Table 3.
Train performance | |
Recall | 0.983 |
Precision | 0.983 |
Validation / test performance | |
Recall | 0.852 |
Precision | 0.573 |
Table 3. Performance scores of the gradient boosting classifier model.
6. Modeling: Feature importance
Feature importance was determined for the gradient boosting classifier model with the top twenty features presented in Figure 11. Interestingly, the annual sum of reimbursements per provider (InscClaimAmtReimbursed_sum) was the most important feature for fraud prediction by a large margin.
The partial dependence of this feature was determined across all values and showed that the fraud prediction peaked for annual reimbursements ranging between approximately $30,000 and $130,000 (Figure 12). This ranges from just over the median value into the upper 1.5IQR (1.5 ร interquartile range) of the population of annual sum of reimbursements for the providers (compare with the box plot for the potential fraud class in Figure 6).
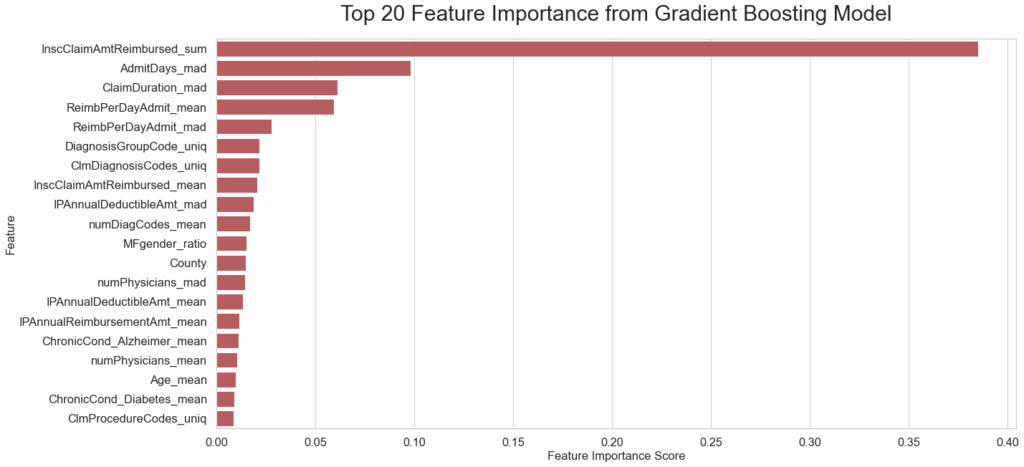
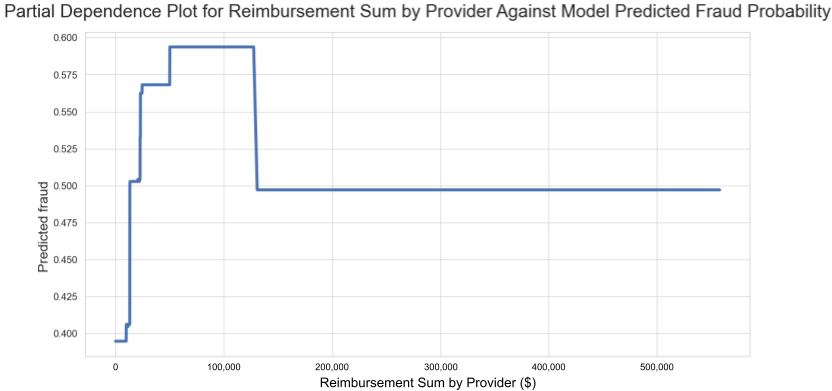
7. Modeling: Hyperparameter tuning
The gradient boosting classifier model required only minimal hyperparameter tuning by grid search and yielded 85% accuracy for predicting the potential fraud class. Model predictions for the validation (test) data are shown in the confusion matrix in Figure 13.
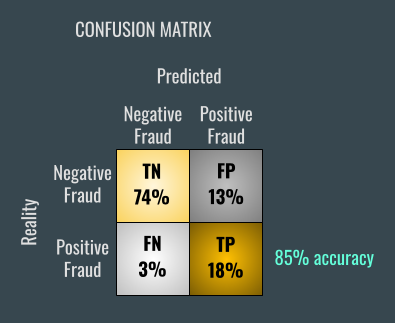
Top Important Features
The top eight important features for the gradient boosting model are listed below. It is notable that four of the top features were directly related to the reimbursement amount, including the sum of reimbursements per provider, the mean reimbursement per provider, and the mean and MAD values for reimbursement per day admitted. Furthermore, MAD values for both the admit days and claim duration features were also highly important. Taken together, this suggested that both the reimbursement amounts and the number of days admitted (highly similar to number of claim days) were highly important determinants of whether a provider was fraudulent.
Furthermore, two additional features making up the top 8 most important features were the number of unique diagnosis group codes or the number of unique clinical diagnosis codes used by a given provider. It can be speculated that these features were proxies for the overall size of a provider and indicated the range of codes that a given provider had available. It can be inferred that larger providers had more codes available to use due to having more resources at a larger medical center.
Top 8 Features from Gradient Boosting Model
- Amount reimbursed (sum for provider)
- Admit days (MAD for provider)
- Claim duration (MAD for provider)
- Reimbursement per day admitted (mean for provider)
- Reimbursement per day admitted (MAD for provider)
- Number of unique diagnosis group codes (for provider)
- Number of unique clinical diagnosis codes (for provider)
- Amount reimbursed (mean for provider)
Actionable Insights
A calculation was done to determine the revenue saved by optimizing this model to minimize false negatives. The model was applied to the validation dataset and average values from the Health Care Fraud and Abuse Control Program Report were used to determine cost savings:
- Investigation cost for positive class (TP + FP): 131 x $260,000 (avg. investigation) = $34 million
- Loss to incorrectly classifying false negatives: 13 x $548,000 = $7.1 million
- Gain from correctly identifying true positives: 75 x $1,451,000 (avg. settlement) = $109 million
- Benefit of applying model (millions $): 109 - (34 + 7.1) = $68 million
A further important benefit of using this machine learning model was that the number of false positives was lower than those found using the standard Health Care Fraud Abuse Control measures in place in 2009 which assigned 55% of the positive class as false positives (considered to be investigations that did not lead to charges (983 / 1786)). In contrast, this model assigned 44% of the positive class as false positives and can be re-tuned to further decrease the percentage of false positives as desired. This decrease in false positives lead to an average savings of $30,000 per fraud investigation.
Improving the Model
Considering the top important features, it is likely that the model can be improved when provided with additional data. Consider that inpatient admission days impacted four of the 8 top features. Therefore, any additional information regarding admission duration could be helpful to bolster model accuracy.
For example, having information regarding the US average admission stay for the 12 chronic conditions present in the beneficiary data could be used to establish a baseline hospital stay for each. Additionally, having information indicating the exact location of the providers (which could only be inferred by the mode values of the beneficiaryโs states) could help to correlate provider state with states known to have higher or lower rates of Medicare fraud (available in records from the FBI and US Sentencing Commission).
Sources
- National Health Care Anti-Fraud Association
- FBI Financial Crimes Report 2009
- Center for Medicare Services, Research Data Distribution Center LDS Denominator Record Data Dictionary 2009
- US Office of Inspector General, Health Care Fraud and Abuse Control Program Report FY 2009
- US Sentencing Commission Quick Facts on Health Care Fraud Offenses
The skills I demoed here can be learned through taking Data Science with Machine Learning bootcamp with NYC Data Science Academy.