Comparing Data Reviews of Beers by Style and Reviewing Body
The skills I demoed here can be learned through taking Data Science with Machine Learning bootcamp with NYC Data Science Academy.
Introduction
As a craft beer lover and homebrewer, I decided to scrape beer data reviews from one of my favorite brewing magazines, Craft Beer & Brewing. For their reviews, three different "entities" supplied comments/reviews. These were the brewer's themselves, a panel of judges, and editors of the magazine. I wanted to see the different ways the entities described the beers and also how these descriptions changed with different beer styles.
Data on Beers & Styles
Reviews were obtained for nearly 1000 beers and were representative of 202 "distinct" styles. The average number of beers per style was under 5, with only a few styles having more than 10, as can be seen below.
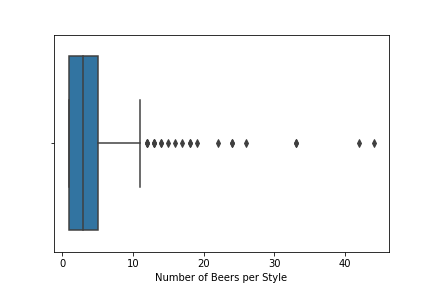
I decided to group beers based on their base style. For example, a coffee or vanilla or chocolate stout became just a stout. I then focused on 10 beer styles: IPA, Stout, Porter, Ale, Lager, Pilsner, Helles, Sour, Saison, Witbier.
NLP Data Analysis
The reviews were processed and word clouds created to compare the content of each review based on both the style of beer and reviewing entity. Below are the word clouds for each reviewing body for the beers taken as a whole.
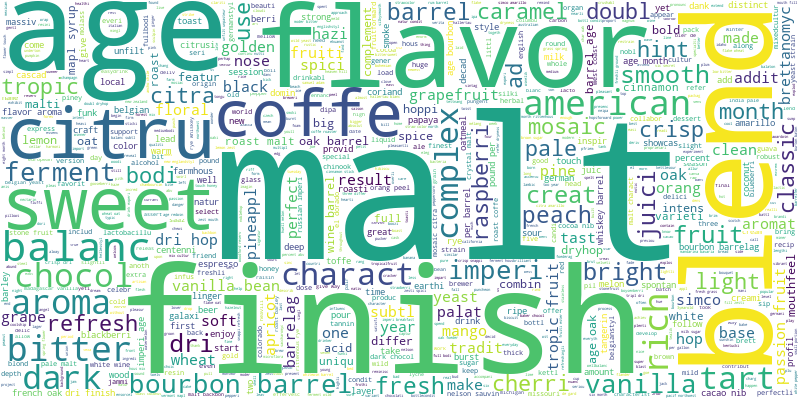
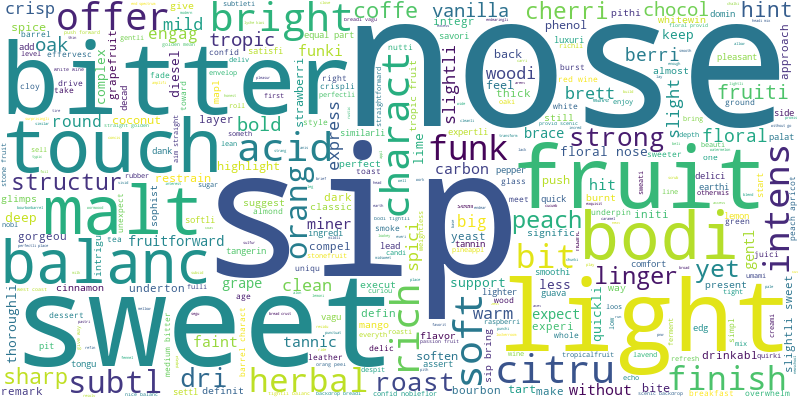
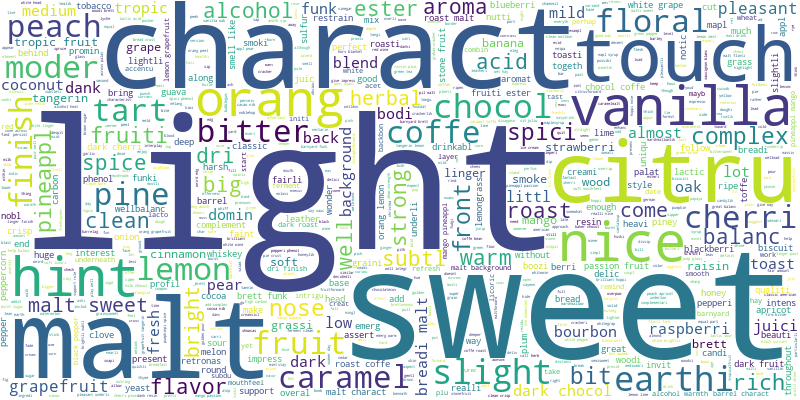
And word clouds for the IPA beer style.
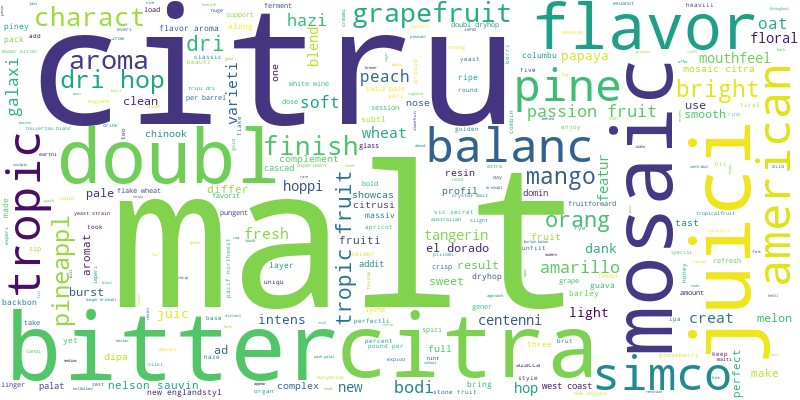
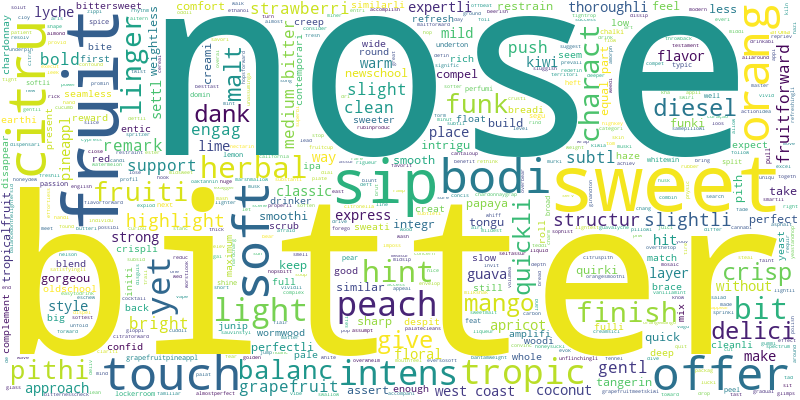
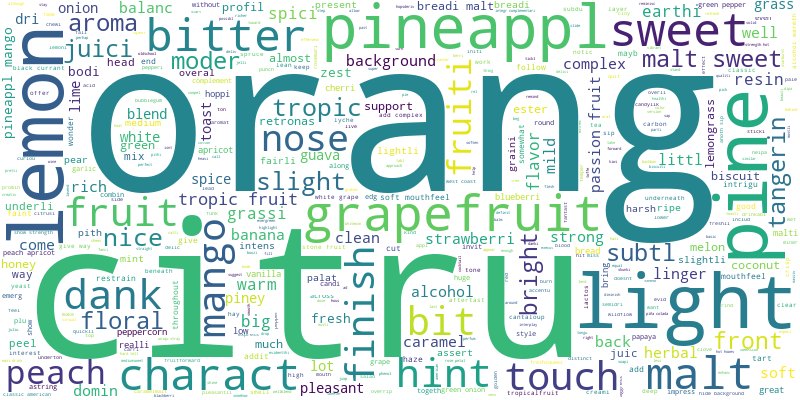
Conclusiovns
There are the obvious differences between descriptions for beer styles. The typical descriptions used to describe and differentiate beer styles are present. As far as the different reviewing entities, there are distinct differences. The brewer's comments focus on how the beer was brewed, similar to if you would ask a brewer at the brewery to describe the beer. The panel's comments focus on the technical aspects of the beer, similar to those from a beer judge. The editor's comments focus on the experience of drinking the beer, similar to if you ask a friend to describe a certain beer. A Shiny app was developed to display the word clouds and can be accessed here.
Code available on GitHub
Photo by Meritt Thomas on Unsplash