Data Analysis on Our Happiness and Environmental Indicators
The skills the author demoed here can be learned through taking Data Science with Machine Learning bootcamp with NYC Data Science Academy.
GitHub
A Lot to Chew On
With the Glasgow Climate Summit starting this Halloween, we are reminded once more of our national and global commitments to halve carbon emissions by 2030---a daunting goal for only 8 years. As Senator Joe Manchin plays tug-o-war with climate strategies in Congress and Covid-19 restrictions decrease in waves, data shows the minor dip in emissions threatens to be meaningless. And so the question is asked: What does our future hold?
This study was an attempt to explore the relationship between human happiness and the environment. It is intended to provide useful insight to policy makers, sustainability strategists, and any responsible company or organization who wants to know how human affect, perception, and mobility might relate to changes in the environment.
Conversely, this knowledge may also be wielded to implement strategies for reducing greenhouse gas emissions and pollution while maintaining or enhancing a happy and healthy constituency / clientele / societal population.
Research Question: What is the relationship between our collective happiness and the state of the environment?
The following analyses were derived from data provided by the United Nations Statistics Division (UNSD, dataset for Global Environment Indicators, 1990-2017) as well as Gallup's World Happiness Report (WHR, 2004-2019).
Looking for Data Patterns
The Exploratory Data Analysis (EDA), "spagghetti to the wall" phase of this investigation was a correlation heatmap. After cleaning and synthesizing the available data, patterns emerged which indicated the correlative strength of three Global Environmental Indicators (GEIs) with many measures of World Happiness (WH). The three strongest GEI variables were: (1) Population (%) Supplied by the Water Industry, (2) Energy Supply Per Capita, and (3) Population (%) Connected to Wastewater Treatment Systems.
For WH measure, I chose to focus on three variables that were most indicative of emotional well-being and positive outlook: (1) Life Ladder, (2) Freedom to make life choices, and (3) Positive affect. Future studies may want to consider exploring the inverse correlation of Negative affect, as well, to determine what GEIs can be predictive markers for social unrest or unwellness.
EDA Correlation Matrix highlights high correlations between WH Measures and three GEI measures: Population (%) Supplied by Water Industry, Energy Supply Per Capita, Population (%) Connected to Treatment of Wastwater.
(For the curious reader unfamiliar with correlation matrices, consider the preceding chart a mirror image of itself across the diagnal yellow line. The same list of variables naming rows from top to bottom apply to the columns from left to right. Each box's variables can be found by following the line to a name on the left, and doing so again with that point's mirror image on the other side of the yellow line.)
To observe these patterns in more detail I compared these relationships with lesser-correlated variable pairs and conducted analyses with groupings by time of data overlap (2004-2017) and by economic tiers (Log GDP per capita).
Direct Data Comparisons
The following comparisons are a glance at the how the patterns revealed in the correlation matrix manifest as contrasted with lesser-correlated variables in the same GEI sector.
The two scatterplots on the left are Energy Supply Per Capita (high-correlation, top) and Energy Supply (low-correlation, bottom), and the two on the right are Population (%) Supplied by the Water Industry (high-correlation, top) and Freshwater Resources Total (low-correlation, bottom). All four variables were plotted with average Life Ladder score, a happiness measure that surveys participants on where they feel they land the "ladder of life"; their sense of achievement or satisfaction with their job, family, house, etc.
(Due to the diversity of culture and language around happiness coupled with the arguably ethnocentric measures of Gallup's World Happiness Survey, the WH measures in this study are subject to limitation of implicit bias and other cross-cultural research dilemmas.)
Energy Supply Measures (left) and Water Measures (right) show varying levels of contrast in correlation coefficients between general environmental indicators and environmental indicators which pertain directly to population individuals.
These direct comparisons elucidate the correlation as a reflection of whether the GEI measures general natural phenomena and resource measurements not directly related to human activity (Freshwater), or is an indicator directly related to human activity. The population and per capita measures plotted above are the most strongly correlated, while a general measure related to human activity but not explicitly telling of the individuals' experience is slightly lesser correlated (Energy Supply), and a natural phenomenon or resource that in a globalized economy often no longer determines the human experience (Freshwater) has a coefficient near to zero.
This yielded the tentative conclusion in the form of a question:
Is human happiness strictly correlated with environmental indicators of personal access to resource supply and waste?
Though hippies and dreames might be disappointed, such an implication---which of course only reflects the mapping of this specific dataset---is somewhat intuitive and unsurprising. The seemingly unfortunate aspect of this implication is that those working to move our society towards carbon neutrality and sustainable living methods must carefully yield to the fact that over 80% of the world's energy supply is derived from fossil fuels, and that to lower this supply dramatically without sufficient replacement with renewable energy could broadly decrease population happiness.
This question led me to investigate other possible solutions, by exploring the interplay between the high-correlating GEIs and how they relate comparatively to WH variables.
Supply and Waste Treatment
To further investigate the nuances of how the high-correlating GEI variables interact with each other and with the WH variables (plots below), I isolated water system features (supply and waste treatment) over time (top), and afterwards plotted them against the "Freedom to make life choices" WH measure (bottom). The water systems plot (top) demonstrated a visible correlation in movement, as well as a gap (~15%-30%) between the percentage of population being supplied water by the water industry and percentage connected to wastewater treatment.
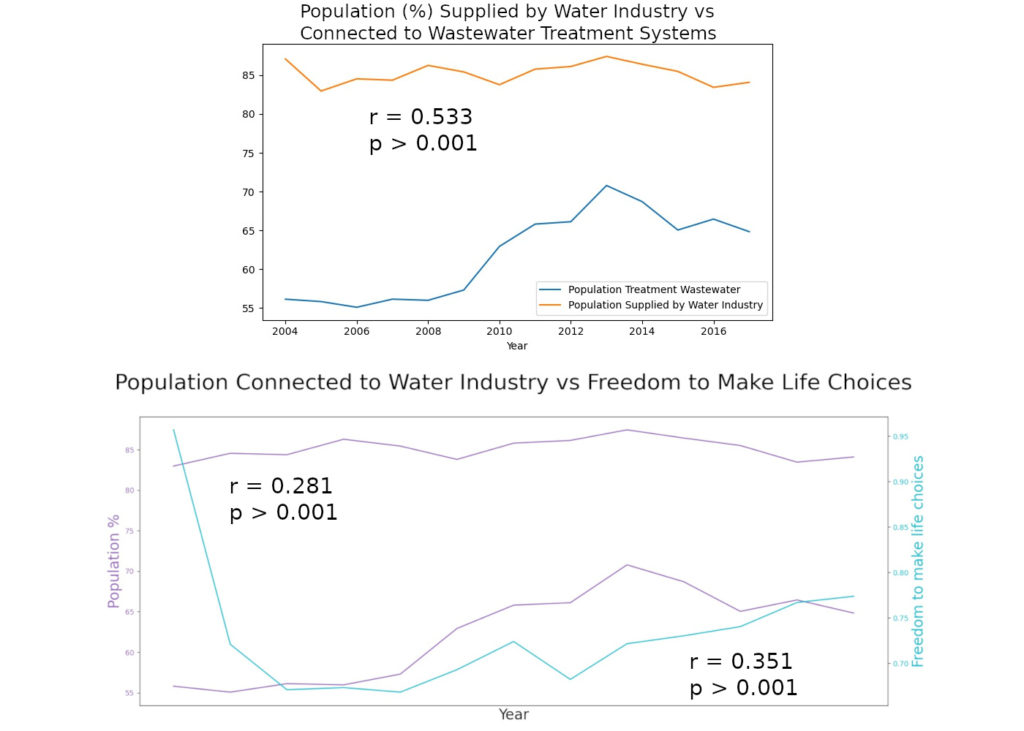
(bottom) Population (%) water system measure vs happiness measure "Freedom to make life choices" demonstrates the respective correlation between water systems access and happiness.
The added WH measure (bottom) allows us to compare the strength of these correlations. Since water supply is relatively high and consistent for many countries, and waste treatment is a luxury that many populations still do not have access to, it makes sense that the correlation coefficient with happiness would be stronger for the latter. However, in the interest of pursuing actions that bring our infrastructure and geopolitical landscape closer to one of sustainability and peace, this relatively strong correlation between wastewater treatment access and happiness measures leads me to tentatively suggest that:
...improving and expanding waste treatment systems might be a way to help populations be happier while simultaneously moving toward sustainability.
Another consideration:
The plot above could be a reflection of a general societal prioritization of creation over cleanup. That is to say, the disparity beween supply and waste management in terms of averaged global water systems might be an indicator that our industry is more focused on extracting and distributing materials than it is about managing the downstream effects of these same materials. It might behoove our society to consider the prioritization of waste treatment as a valuable industry that provides both environmental wellness and and collective happiness, and could be prioritized to be at least as critical as manufacturing and material sales. Still, further investigation using a more complete dataset are needed before any strong conclusions can be drawn.
Untangling Nuances
To further understand the interplay of factors that contribute to the way environmental indicators relate to happiness variables, I began parsing populations according to economic factors. The economic variable available from the WHR was the "Log GDP per capita" measure, which takes the natural log of exponential economic growth to indicate how relatively quickly the economy is growing, as divided by population.
LGDPc Data
To better understand this variable and it's impact on countries' GEIs and WHs, I divided the data in four percentile tiers of Log GPD per capita (LGDPc), as imaged below in relationship with Population (%) Connected to Wastewater Treatment.
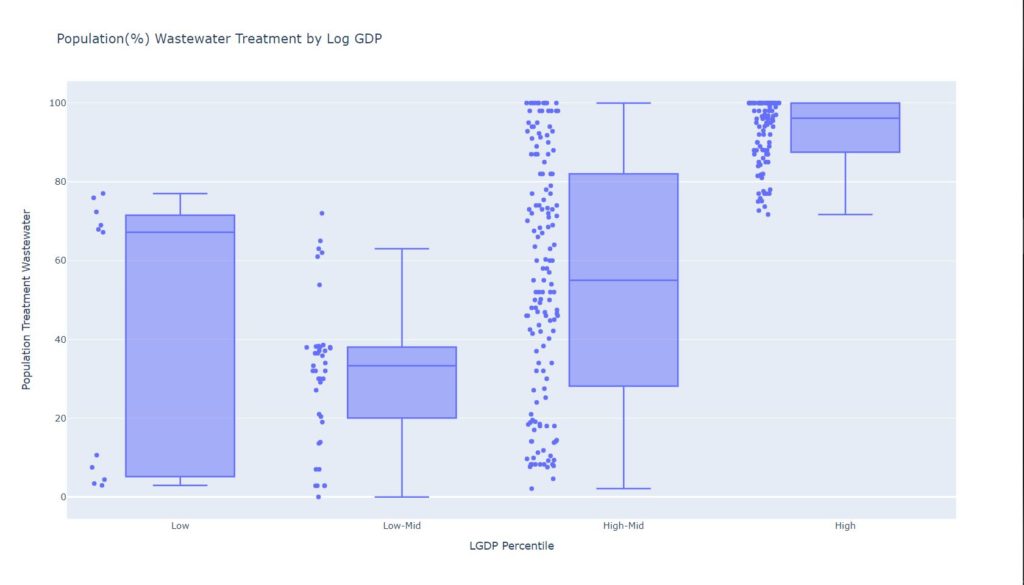
The plot indicates a broad dispersion within some tiers (a.k.a. that being in a High-Mid growing economy does not indicate that you will have access to wastewater treatment) and a much denser dispersion in others (the High tier, especially). Additionally, it indicates that:
...there is a dramatic gap in this GEI dataset for countries from slower growing economies.
With that in mind, let's take a look at how LGDPc interacts with GHG Emissions (1000 tonnes of CO2 equivalent) and the WH measure Positive Affect:
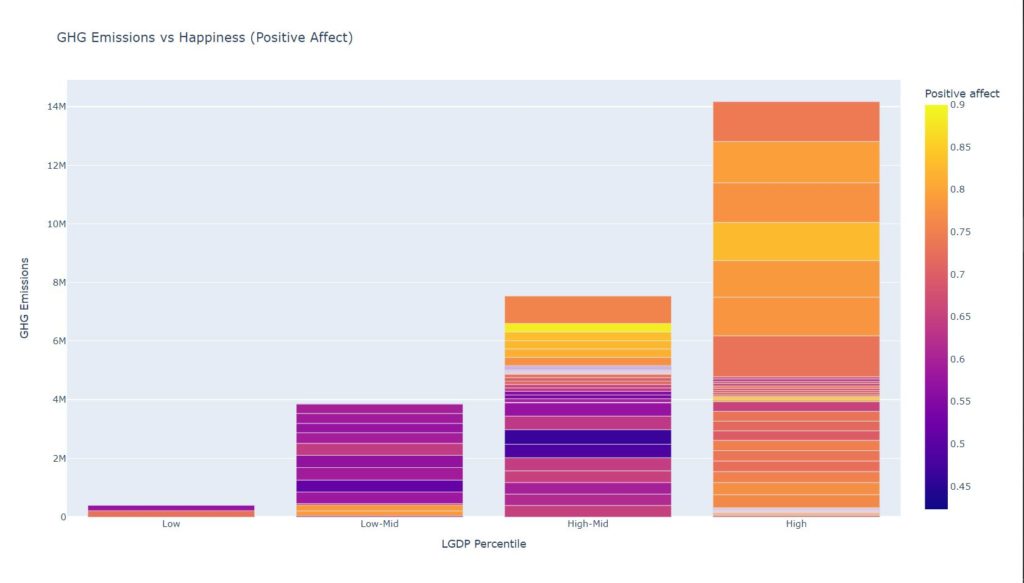
This plot colorfully depicts LGDPc as a strong indicator of both GHG Emissions and happiness. That is to say, high-growth economies are releasing more GHGs and also are generally hosted by happier populations, according to WHR measurements. However, considering the data gaps demonstrated previously, these statements can't hold water until further research is conducted on a supplemented data set.
Looking Forward
So where do we go from here? The above research and analyses yielded a wealth of questions and potential avenues for future research to parse the nuances of human happiness and environmental conditions. While the analyses and datasets selected were not sufficient to provide sea-worthy plans of action, there are a few takeaways for future steps with which I would like to leave you:
-
Improvement in data
In addition to filling in the GEI gaps for lower percentile LGDPc countries, there is a strong factor of unreliability in the way information of these indicators is collected. To quote directly from the UNSD dataset that informed this article:
"When interpreting these tables, it should be borne in mind that the definitions and estimation methods employed by member countries may vary considerably, which may be mentioned in the footnotes."
Besides the unquantifiability of human interpretation for the data that was present, it should also be noted that very little, undated information existed for broad sectors of environmental wellbeing. For example, the "Biodiversity" folder had one small chart named "Terrestrial-Marine Protected Areas" that listed an estimated percentage of land protected for each country.
To me, this is a strong indication that the data represented in this dataset specifically is less of a holistic representation of the environment, but more of a random sampling based on easier-to-access recorded human data that by nature is more likely to exist in more developed countries. However, such data can still yield at best ideas and springboards for exploration.
In conducting future research I should like to find and compile datasets with more detailed, nuanced information for local to global environmental conditions than was available here, as well as a investigate whether the WHR happiness measure explores and accounts for cultural diversity in quantifying the concept we call "happiness."
- The use of Machine Learning to further untangle contributing factors, such as LGDPc, regional climate, etc.
Conclusion
One of the most exciting results of this project to me was the realization that the detective work of data science is to use computers to observe unanticipated patterns and interactions between and within an infinite array of variables. Machine learning is a boon waiting to be unleashed, a compacted explosion of unimaginable implications beginning to be weilded to it's full, all-life-benefitting potential.
Most sectors have been moving in this direction with their research, I believe if we begin with small regional projects to analyze relationships like the one studied here to create reliable machine learning models that incorporate widely varying environmental information with widely varying information of human wellness, that we would have an analytical tool that would be able to provide much more thorough and reliable analyses of past, current, and possible future conditions. I look forward to learning more about such techniques and learning to handle them responsibly and for the benefit of all.