Data Is In The Eye Of The Beholder
The Ames Housing Data has become a rite of passage at the New York City Data Science Academy. A simple search for the terms “Ames,” “Housing,” or Machine Learning” will yield over a few hundred blog posts that all refer to the same dataset. Is the relationship between house prices and the 79 features linear? Non-linear? Some shade of gray? Or are they simply in the eye of the beholder?

In search of the “perfect” model, I reviewed countless blog posts. Who achieved the highest R-squared? What features, methodology and model did they use? Did they cross-validate their results?
What I discovered was that while every write-up presented a different viewpoint, there was no “perfect” model. As data scientists, each one of us has our own “eye” for the data.

Dr. Dean De Cock compiled this expansive dataset and created a model that uses 36 variables to explain 92% of the variation in sales. I experimented with more than 75 different machine learning models and variations to see if I could find a combination that performed better. I observed that simple and multiple linear regression underperformed penalized and tree-based methods. Out of all the models that were tested, the CatBoostRegressor emerged as the top performer.
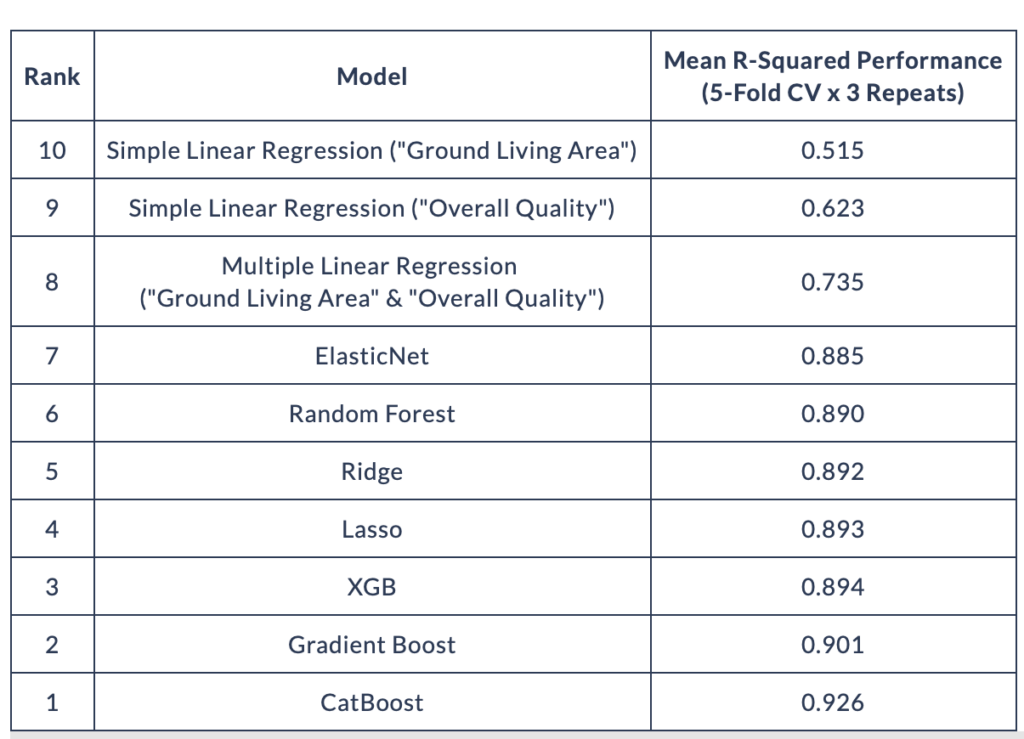
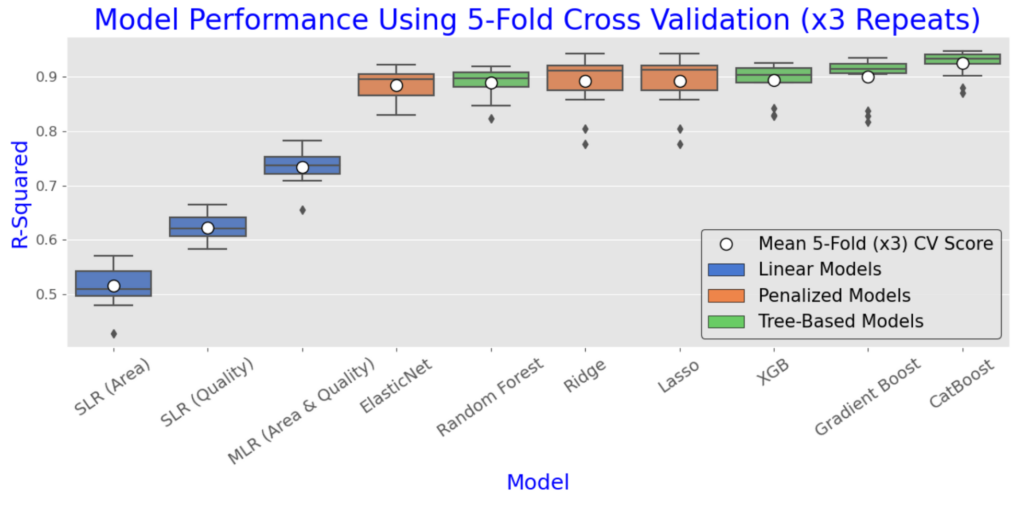
CatBoost is a gradient boosting algorithm; its name is derived from “category” and “boosting” for its exceptional ability to deal with categorical data. The large number of categorical features in the Ames Housing Data is one of the main reasons why the CatBoostRegressor outperforms all other models out of the box. Categorical features in this dataset include the type of dwelling, the type of road access to the property, the general shape of the property, and the neighborhood. In total, there are 46 such categorical features and only 33 numerical ones.
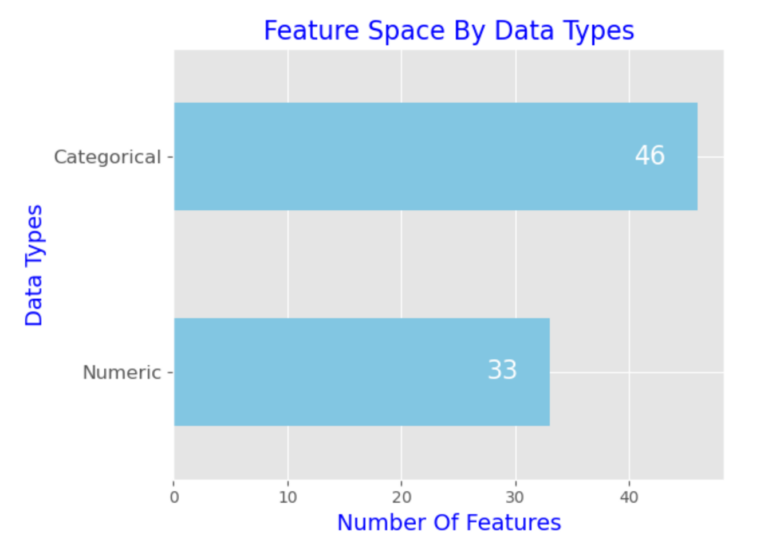
The CatBoostRegressor achieves a 92.6% mean cross-validated R-squared without removing any features or observations from the dataset. Dr. Dean De Cock recommends the removal of all observations with more than 4,000 square feet of “Ground Living Area.” The model performance improves to 93.8% if I follow this recommendation.
However, identification and removal of outliers often requires domain expertise and a detailed understanding of the data collection process. Data is expensive and must be treated with care. While an observation could visually or statistically be an outlier in a two-dimensional space, such as “Ground Living Area" vs “Sales Price” or “Lot Area” vs “Sales Price,” it is possible that the same observation provides value and insight to tree based boosting models which learn sequentially and are better equipped at capturing non-linear relationships and feature interdependence.

A simple summary plot using SHAP values highlights that the CatBoostRegressor interpreted “Ground Living Area” to be the most important feature when predicting house prices. The size of the Ground Living Area could affect the SHAP value (or model output of the CatBoostRegressor) by as much as US$100,000 or more.
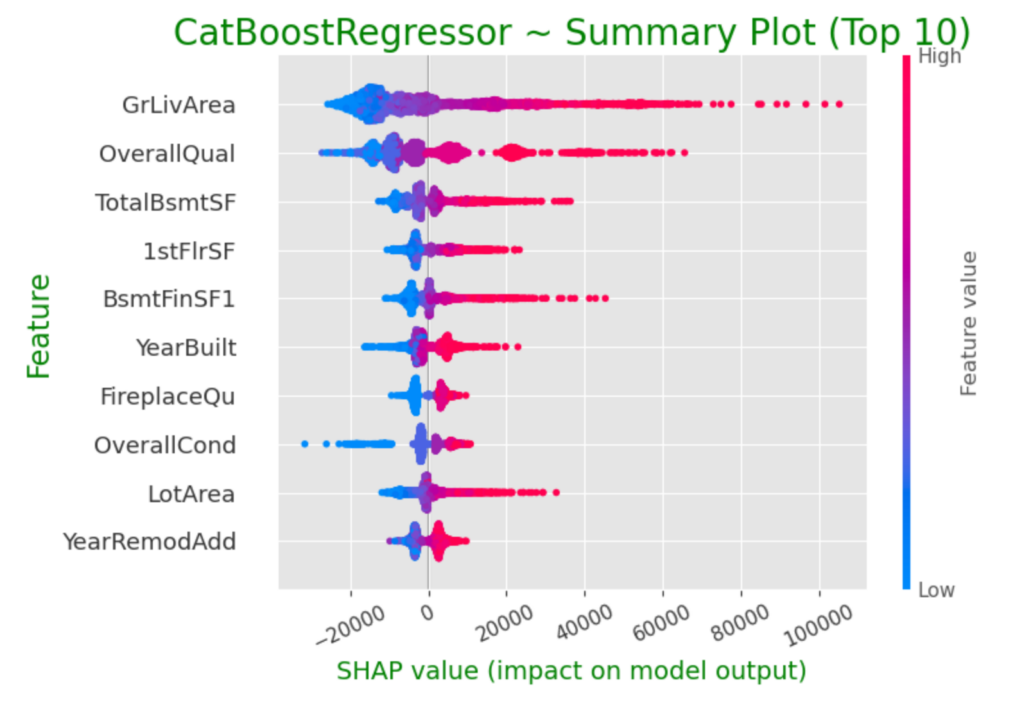
In contrast, both the GradientBoostingRegressor and XGBRegressor opted for “Overall Quality” of the home as the most important predictor.
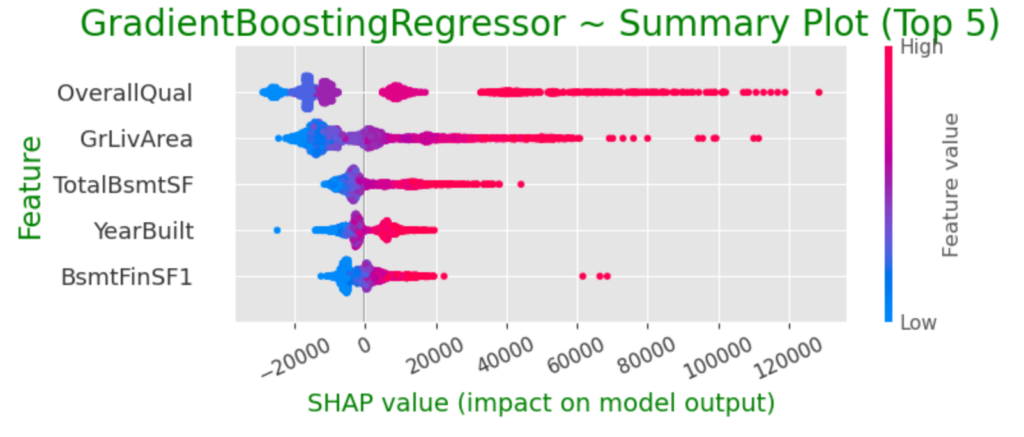
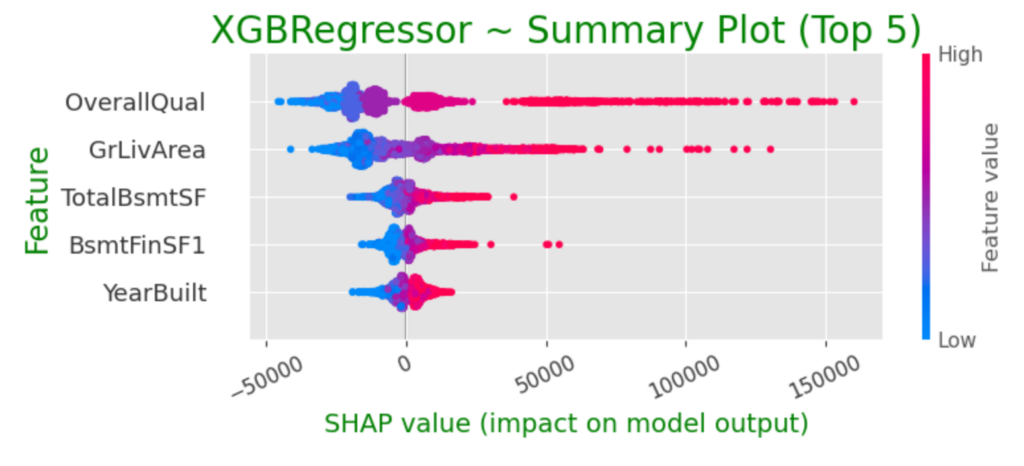
When dissecting a SHAP dependence plot for “Overall Quality”, we can gain further insights from each of the three tree-based models. Let us first compare the three dependence plots without any feature interactions.
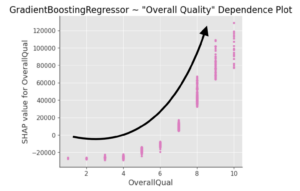
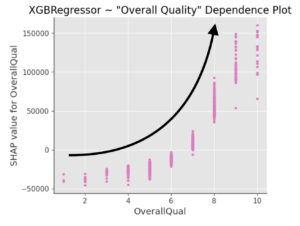
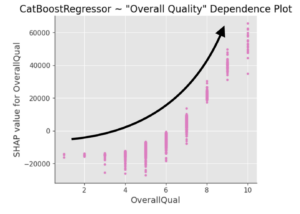
In each of the dependence plots above, we notice a positive exponential relationship between the Overall Quality of the home and its SHAP value. Homes with an Overall Quality greater than 6 are likely to receive a positive SHAP on their valuation. Furthermore, the positive SHAP contribution increases in greater amounts at each incremental level of overall quality from 7 to 10.
SHAP dependence plots can also highlight an “interaction feature”, allowing us a better understanding of any feature interdependence that was captured by the respective models. Let us now compare the same three dependence plots using feature interactions below.
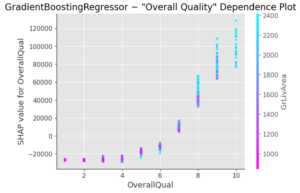
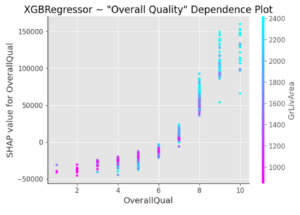
The GradientBoostingRegressor and XGBRegressor conclude that “Ground Living Area” was the feature with which “Overall Quality” had the greatest interaction. In both of the dependence plots above, we see that homes with an “Overall Quality” of 8 or higher were mostly those with larger “Ground Living Area.”
However, the CatBoost (once again) comes to a different conclusion. The CatBoost determines that “1stFlrSF” (First Floor Square Footage) has greater interaction with “Overall Quality” than “Ground Living Area”. In the plot below, the SHAP values for Overall Quality behave differently above and below an “Overall Quality” of 8. Homes with a lower “Overall Quality” (i.e. a score of 7 or lower) had higher SHAP values for those with smaller First Floor Square Footage and lower SHAP values for those with larger First Floor Square Footage.
Here, the common sense approach of larger living space equating a larger valuation does not hold. For homes of lower quality, there was a positive valuation if the size of First Floor Square Footage was smaller. For homes of higher quality (i.e. a score of 9 or 10), however, a larger First Floor Square Footage corresponded to a higher SHAP contribution. An Overall Quality of “8” is where this relationship pivots as depicted in the dependence plot.
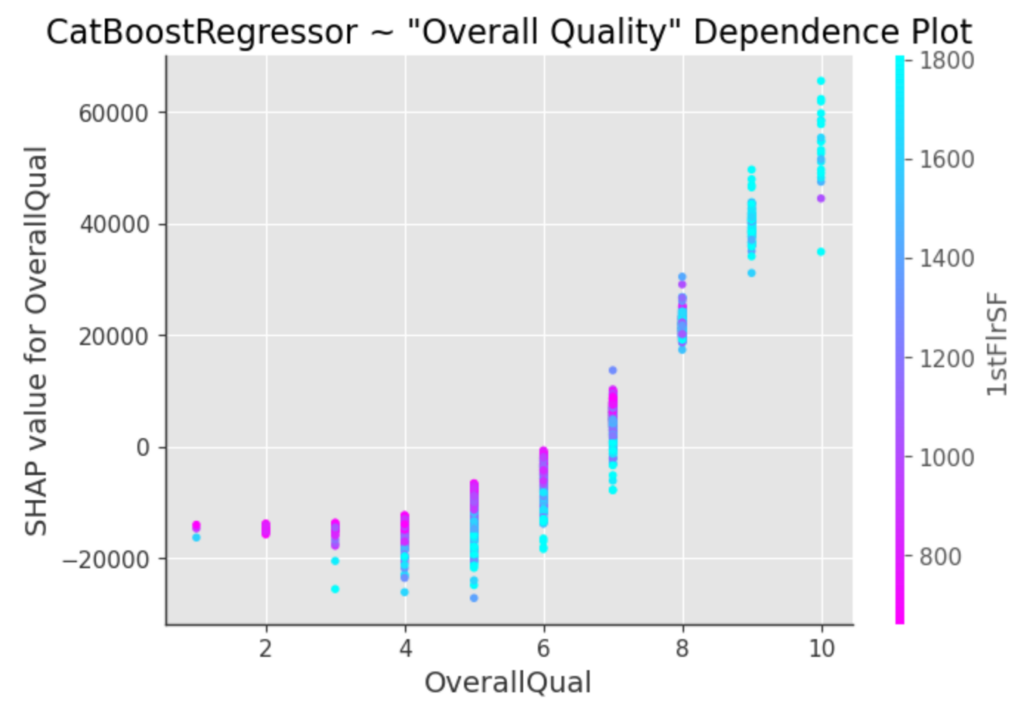
While GradientBoosting and XGB fail to capture the importance of First Floor Square Footage in their top 5 most important variables, CatBoost ranks “1stFlrSF” as the fourth most important when determining house prices. The combination of these subtle differences are few of the reasons why the GradientBoostingRegressor and XGBRegressor underperforms the CatBoostRegressor by at least 2.5%.
Perhaps the CatBoost (like the cat pictured below) has the best “eye” when it comes to the Ames data set.

Credits, References & Special Thanks:
- Instructors: Zeyu Zhang, Sam Audino, Luke Lin, Vivian Zhang
- Mentors: Jonathan Presley, Ariella Brown, Anant Swarup, Courtney Graves
- Data Sets: Please refer to My Github for the variations available
- My Github
- Shutterstock Image Credits ~ Licenses Purchased
- Black and White Abstract Woman (ID: 552131713)
- Hi Tech Biometric Retina Security Scan (ID: 663722314)
- Close up view of blue eye in glasses (ID: 1980190340)
- Conceptual abstract picture of the eye (ID: 1445018480)
- Handsome man is holding cat (ID: 1845180379)