Data Analysis: What's in Your (Doctor's) Wallet?
The skills I demoed here can be learned through taking Data Science with Machine Learning bootcamp with NYC Data Science Academy.
RShiny App | LinkedIn | GitHub | ResearchGate
Project Summary
Financial relationships between clinicians and the healthcare industry are common, and frequently necessary to improve the standard of care. Nevertheless, while patients like you and I are the consumers of life-saving products, the doctors and hospital administrations are the actual customers, as they decide which products to administer. To what extent, if any, do healthcare industry payments to physicians affect the subsequent rates of prescriptions - especially within the context of the current opioid epidemic?
Using publically available datasets, the current project aims to gain key insights into the relationships between (1) industry payments and physician subspecialty, and (2) pharmaceutical payments and the number of prescription claims. The results found that modest industry payments, less than $100 in meals, were associated with a two-fold increase in Oxycontin. While the elimination of all physician payments is unrealistic, it is vital that we, as patients, are aware of potential forces influencing the doctors responsible for our care.
Background
The Department of Health and Human Services (HHS) regulates and heavily enforces the 'Anti-Kickback Statutes', allowing the industry to pay physicians for services rendered while forbidding physician payments tied to the use of medical products [hss.gov]. Physician services may include developmental research and product design, post-market clinical studies, general consulting and promotional speaker fees, and perhaps most commonly - meals.
Two datasets were used in the current project. The first, obtained from the Centers for Medicare & Medicaid Services (CMS), contains over 24 million physician payments occurring in 2016. The publically available database, established by the Affordable Care Act passed by Barack Obama in 2010, includes over 70 variables describing the physician, healthcare practice, corporation and industry type, and nature of the payments.
The second dataset, obtained from ProPublica.org, contains over 3 million prescription claims written by doctors in 2016 and includes payments from pharmaceutical companies to doctors specifically treating Medicare patients, the number of prescription claims, and the cost of the medication (since it is paid for by the government). The ProPublica dataset combines specific information from the CMS dataset, and a separate Medicare Part D database established by the Medicare Modernization Act passed by George Bush in 2003.
RShiny App
While the ProPublica dataset contained the Top 50 drugs based on total physician payments (3 million observations), the dataset was reduced to the top 20 drugs due to the limited computation power of the RShiny app. The truncated dataset included key variables such as number of payments, total payments, total payments for meal, non-meal, or promotional lectures, number of 30-day Rx claims, and cost of drugs paid for by Medicare, per physician. In total, the dataset used in the project included 900,000 observations collected from over 250,000 physicians.
Figure 1 includes the drug name, organized by drug type, analyzed in the current project. An R Shiny app [link] was created for exploratory data analysis of the truncated dataset. The app includes four sections that include project background (Figure 2), exploration of the dataset by variable (Figure 3), initial analysis of 'effect of physician support' by payment type (Figure 4), and an 'about author' section. The CMS dataset was used only for exploring the number of industry payments across subspecialty and healthcare product type (biological, pharmaceutical, or device), and was not included in the RShiny app due to the size of the dataset (24 million payments)
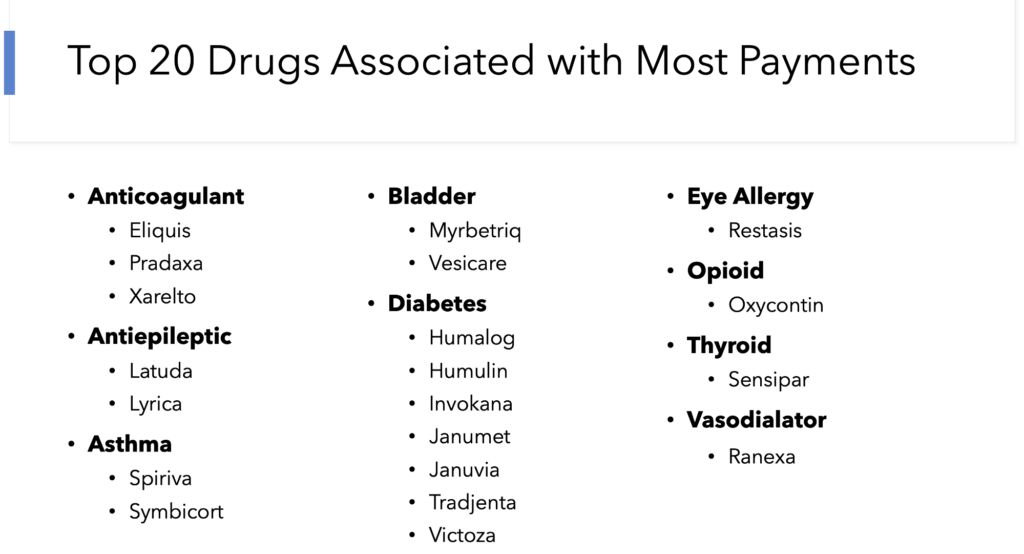
-
Figure 2. Project Background -
Figure 3. Explore Drug Data -
Figure 4. Initial Analyses
Results
Amount and quantity of payments vary by subspecialty
Internal Medicine subspecialty includes over 150,000 physicians, receives the most number of payments, and as you would expect, receives the largest total payment amount. Alternatively, Orthopedic surgery includes far fewer physicians, a tenth of the number of payments, yet receives nearly the same amount of total payments (Figure 5).
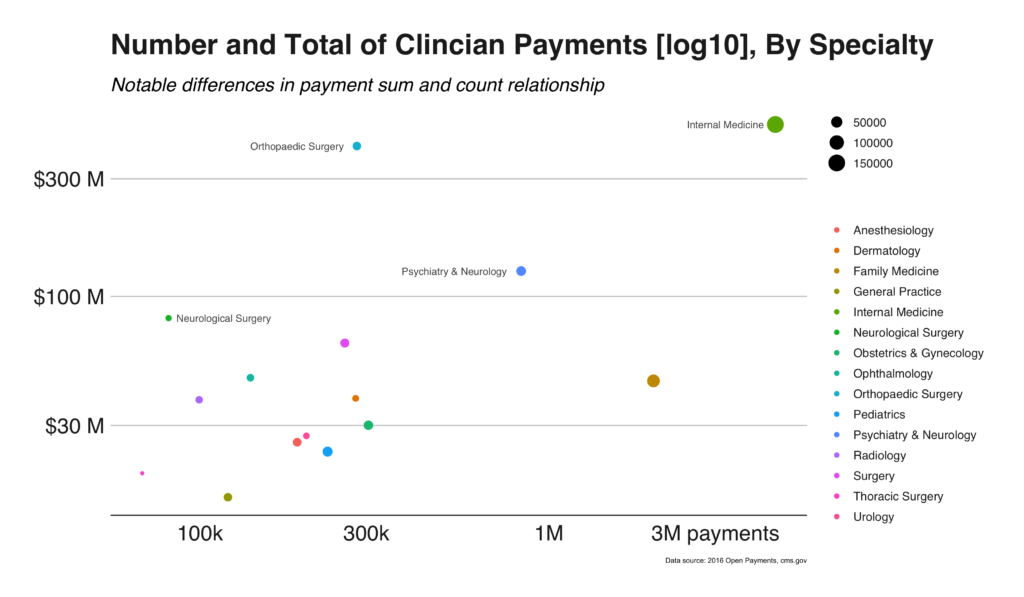
Figure 5. Total payment sums as a function of the number of physician payments, per sub-specialty
Drug companies make a majority of payments
Regardless of physician subspecialty, drug companies make up a significant amount of the payments to physicians (Figure 6), further emphasizing the need to study the relationship between physician payments and Rx claims written.
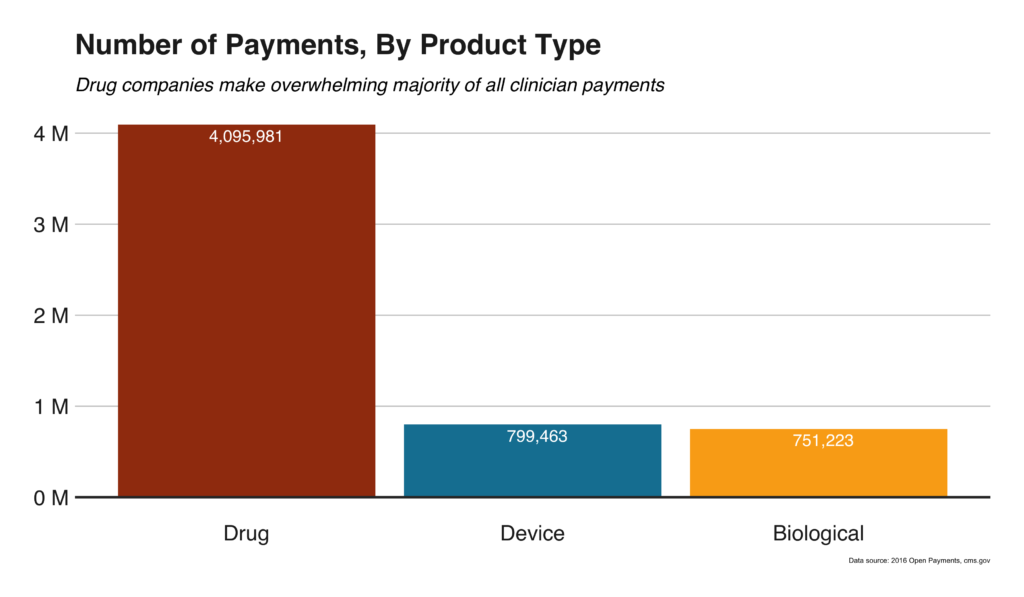
Oxycontin among most prescribed drugs
Exploratory data analysis featured in figures 7-8 include the average number of prescribing physicians and 30-day Rx claims, and percentage of physicians receiving payments, per drug, respectively; Oxycontin is highlighted in yellow.
-
Figure 7. Prescribing physicians -
Figure 8. Number of claims -
Figure 9. Percentage of paid physicians
Free meals affect number of Rx claims
Figure 10 highlights the discrepancy between the number of payments and the type of drug. For example, Ranexa (vasodilator) averaged $1,800 in payments, per doctor, while Oxycontin only averaged $95, suggesting different payment types may exist between drugs. Across all drugs, promotional lectures, on average, accounted for nearly 40% of physician payments while non-meal payments like R&D, consulting, and travel accounted for a slim majority of payments per physician (Figure 11)
-
Figure 10. Average clinician payment -
Figure 11. Average payment type, across all drugs
More significantly, Figure 12 highlights differences between number of Rx claims for physicians that received (1) no payments, (2) payments only in the form of meals, and (3) payments of all types (R&D, promotional lectures, or meals). The general trend was observed across all drugs: no payments < meals-only < all payment types. Surprisingly, physicians that received payment in the form of meals prescribed drugs at a dramatically higher rate than their counterparts that received no meals.
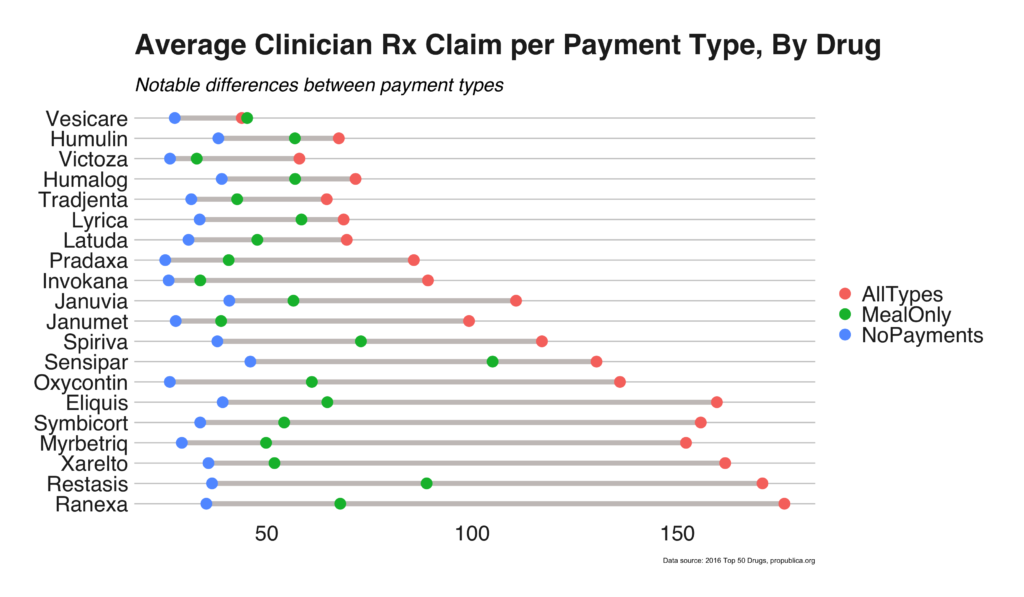
Payment amount affects number of Rx claims
While the previous figure suggests different payments may be attributed to the different number of Rx claims, in all likelihood, the differences are more likely attributed to different payment amounts. When the physicians are binned based on payment amount, a noticeable trend becomes apparent: physicians receiving more payments (by amount) write more Rx claims (Figure 13); Figure 14 includes Oxycontin sub-analyses. Of the clinicians included in the ProPublica dataset, receiving only $100 in a given year increases the number of Oxycontin claims by 120%.
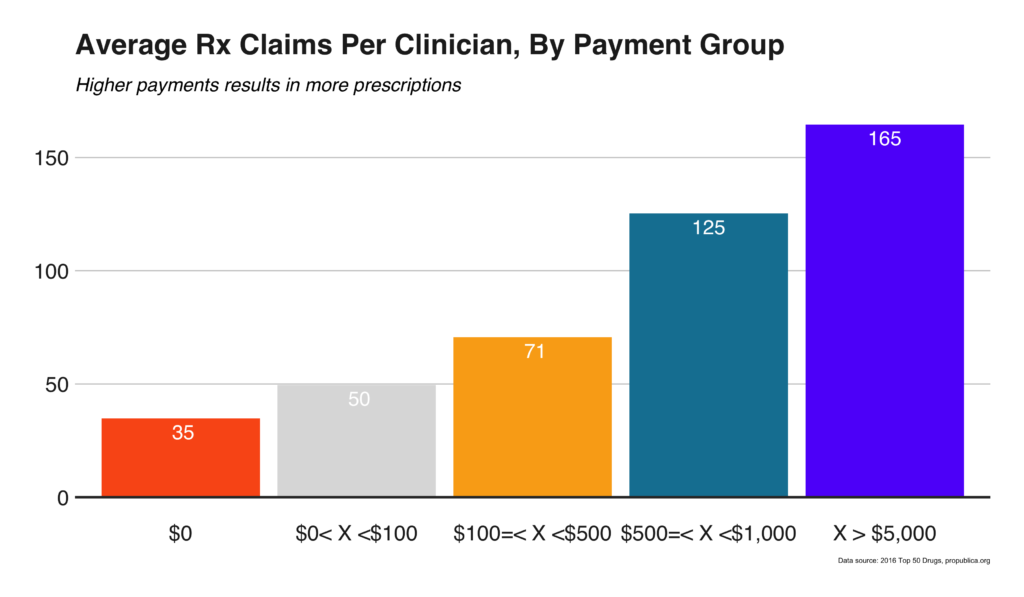
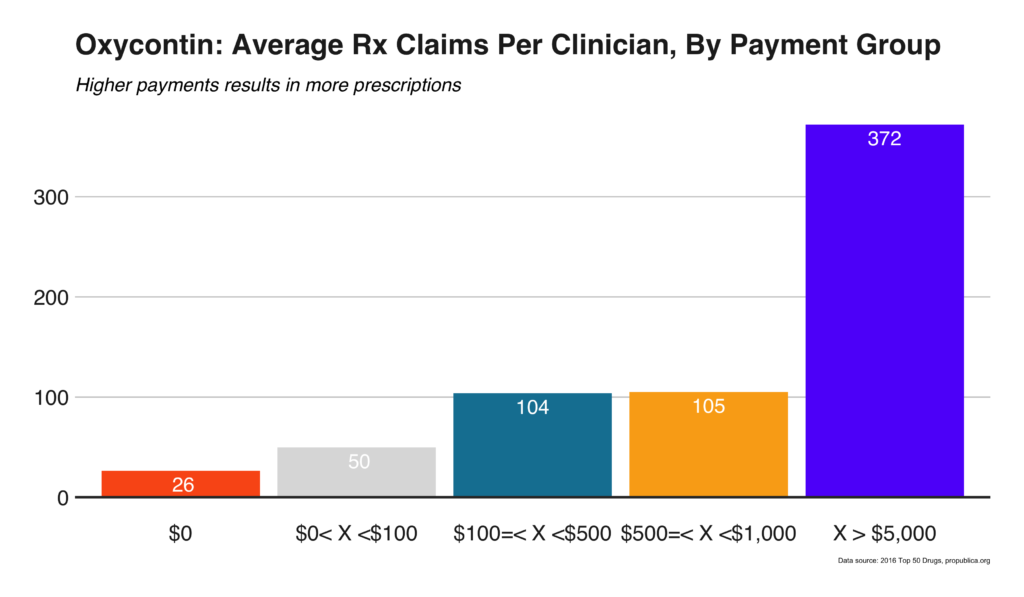
Paying physicians remains highly profitable
Finally, while physicians are paid for services not related to the direct use of their products, the question must be asked: is paying clinicians financially advantageous based on the total payment amount in a given year?
Figure 15 highlights the percentage of physicians that result in a net profit [Cost of drugs - payments made to clinician > $0].
Across all drugs, physicians receiving payments between $100 and $1,000, on average, prescribe enough drugs to offset those payments by the manufacturer (100% of physician payments resulted in a profit); that percentage drops when physicians are paid more than $5,000.
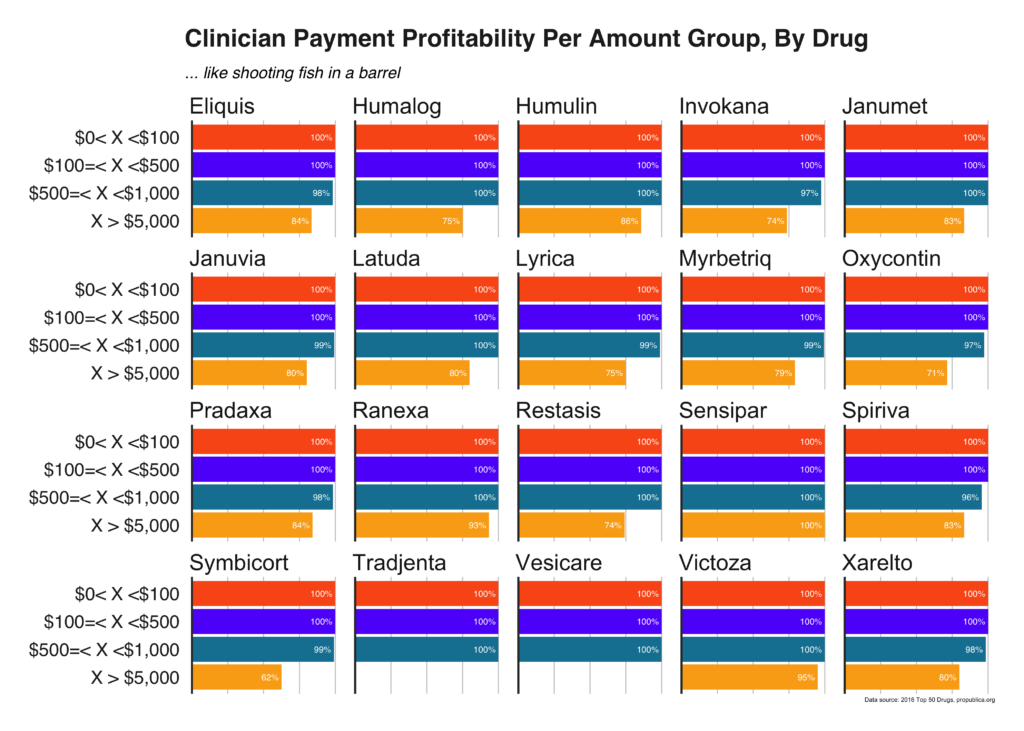
Limitations
While the presented results suggest physician payments are associated with an increase in prescription rate, it should be prefaced that correlation does not equal causation. Moreover, the drug claims in the ProPublica dataset only include medications paid for under the Medicare Part D prescription drug program.
Additionally, the data analyzed is not representative of all Medicare/Medicaid patients since it only includes two-thirds of all beneficiaries. Lastly, the interpreted data is not representative of the physician's entire practice and does not account for the quality of care. Nevertheless, results of the current project are supported by previous academic investigations by DeJong et al. (2016) and Brax et al. (2017); Nusrat et al. (2018) go even further writing,
"Considering the known impact of such benefits on prescribing patterns and other professional behaviors, policy makers should consider revising regulations governing interactions with industry and disclosure formats alerting others to their potential biasing impact."
Conclusion
In 2016, the number of payments and total amounts varied widely by physician subspecialty. Drug companies made twice as many payments as devices and biological companies combined. Prescribing doctors averaged 35 30-day Rx claims, and as expected, maintenance drugs were among the most prescribed; Oxycontin among the top 20 drugs. Across all 20 drugs, 40% of physician payment came from promotional lectures, on average.
Most notably, the type of payment affected the increase in Rx claims written. For example, physicians prescribing Oxycontin that did not receive compensation prescribed, on average, 26 claims a year; increasing to 61 and 135 claims for physicians receiving only meals or all payment types, respectively. The average number of Rx claims increased as the sum payment amount increased; this trend was especially noticeable for Oxycontin. Regardless of the payment amount, high rates of profit can be achieved at all payment amounts.
About the Author
If you would like to learn more about the author, please check out my LinkedIn profile. Furthermore, if you would check out my relevant code, please check out my GitHub account.