Web Scraping and Analysis of CPU and Graphic Cards on Newegg.com
As 2016 Black Friday is just around the corner, this is the right time for people to upgrade their desktop. As a computer fan for years, I am aiming to explore the hardware that are selling trend on Newegg.com for my web scraping project. The questions that I am trying to answer are:
Which brand of CPU and GPU that currently sells the best, and how does the parameters of the hardware affect the price?
1. Introduction
When someone wants to check out a newly released hardware, Newegg.com is always the right place to go. The broad product line, great deals and good service makes this online retailer very popular among computer fans. The highly organized web structure also makes it well-suited for getting and comparing hardware parameters. Therefore I decided to scrape this website for all the data needed for analysis.
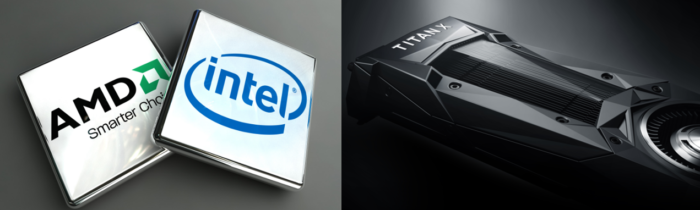
Figure 1. Left: AMD and Intel are competing for CPU market. Right: Nvidia's flagship graphic card---Titan X Pascal
CPU and graphic card, in my opinion, are top-2 hardware in a desktop that directly determine its computing power. Hundreds of channels can be found on Youtube that benchmarking the gaming performance for each CPU and graphic card product everyday. Besides video gamers, data scientists need good computers too. A good CPU and save great amount of computation time when working on a relatively large dataset. More interestingly, GPU computing via graphic cards is going mainstream at this moment. The reason behind this is that a modern GPU will have far more cores than a CPU, thus make GPU well-suited for parallel computing. Today more and more people use high-end graphic cards to perform heavy computational work, like training models for neural network.
2. Web Scraping
As shown above, when searching for a type of desktop components, a page consisting of 36 products will be displayed. The URL contains the information like page number, number of products in a page, and the criteria for sorting the products. The main panel consists of 36 icons. When clicking the "View Detail" bottom, you will be directed to single product page. In this page, most of the parameters can be found under "Specification" tab.
With the web structure in mind, the strategy for scraping is clear. Python Scrapy package was selected for web scraping job. The workflow of web spider can be briefly described as:
- Generate a list of URLs ranging from the first page to the last page.
- At each page, grab the customer rating data, generate a ranking number for each product, filter the undesired (Refurbished and Open box) item, and extract the URL for each product.
- Go to each product page, grab the hardware parameters under "Specification" Tab, and yield the item info.
It should be noted that the product information becomes less organized in the latter pages. Such case are handled by multiple "try/except" statements. The complete code for web scraping can be found here.
3. Data
After web scraping from Newegg.com, the data were cleaned using this R script.
For CPU data, the key variables that are included in analysis are:
name | brand | series | # cores | freq (GHz) | power (W) | price ($) | URL | rating | rank |
chr | chr | factor | int | num | num | num | chr | factor | int |
For graphic cards data, the key variables are:
name | brand | chip | gpu | coreclock (MHz) | memory size | price ($) | URL | rating | rank |
chr | chr | factor | chr | num | num | num | chr | factor | int |
Other variables like L3/L2 cache for CPU, and memory clock/interface for graphic cards are not included in data analysis.
4. Visualization
In this part, exploratory graphs are drawn to visualize the selling trend of CPUs and graphic cards. In terms of the overall market distribution, AMD and Intel are competing for dominance in CPU area, while AMD and Nvidia are two biggest GPU chip manufacturers.
First let's look at the customer rating distribution of CPU and graphic card that are currently selling on Newegg. For CPU, the customer ratings is ranging from 4 points to 5 points, indicating that CPU is overall reliable for nearly all products. On the other hand, some graphic card get rated only 1 points and 3 points, meaning the quality for graphic cards varies from product to product. When looking at the overall markets distribution, the number of Intel products is greater than AMD CPU. As for graphic card market, AMD fall behind Nvidia.
From the barplot above, there are 10 companies sharing the graphic card markets. Some of them, like Powercolor and Sapphire, stand on the AMD side, while others like EVGA and PNY adopt Nvidia GPUs only. It is interesting to note that for companies that use both GPUs, they tends to have more Nvidia chip products over AMD chip.
From the figure above, we can see that in top-100 best selling CPUs, although the average price of Intel product is higher than it opponent AMD, it is still leading in popularity.
Above is the product price plotting against selling rank for top-50 CPU products. The average price increases from $200 to $500 as the rank increases to around 25, and drop below 200 when reaching rank 50. There are three Intel products ranking between 20 and 30 that are selling at above $1000 dollars, thus pulling up the average price in this region.
As shown from the boxplot above, a positive correlation can be observed between price and number of cores. We can also clearly observe the price gap between two CPU manufactures, and such gap becomes more clear at higher number of cores.
5. Statistical Analysis
In this section, the relationship between the price of CPU products and their parameters is explored. First let's look at the correlation plot including all numeric variables in the CPU dataset.
It is interesting to note that the price of CPU almost has no correlation with the operating frequency, meaning that the operating frequency no longer reflects the computing power. Today CPU manufactures direct their attention on cores, threads and inner architecture to increase the performance, instead of simply adding clock speed.
Now let's see if we can use linear regression to model the price of CPUs against other variables. First let's check the normality of the price distribution.
From the Q-Q plot above, the prices are not quite normally distributed. Therefore we do a box-cox transformation.
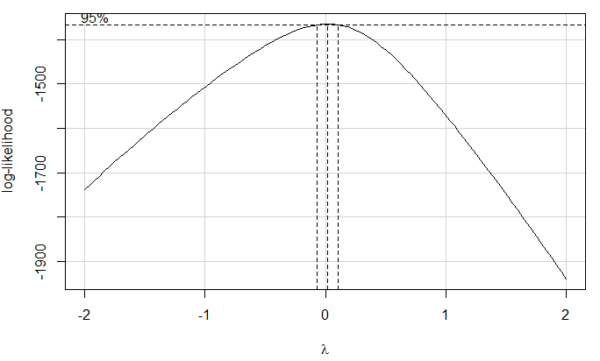
Figure 11. Log-likelihood vs. lambda indicating 95% confidence interval about the maximum observed value for lambda
From figure 11, we take the natural log of response value to increase the normality. For variable selection, CPU brands, series, number of core, power consumption and operating frequency are selected as the full model. Then AIC was applied to select the best linear model via step function in R. The variables for final model are series, power and number of cores.
Increasing of the power consumption will slightly increase the price, and CPU will cost a lot more when adding more cores to it. According to the p-value of the F-test, the overall regression model is significant, where 87% of the variability can be explained.
6. Conclusion and Future work
In summary, all CPU and graphic card product information was scraped from Newegg.com using Python Scrapy module. the data showed that the price of the CPU have a negligible correlation with the operating frequency. When looking at both CPU and graphic card data, although AMD has a wide range of product line, it is dominated by Intel and Nvidia in CPU and GPU market, respectively. A linear regression model is selected to model the natural log of price using CPU series, core and power consumption as predictors, which can explain 87% percent of variability.
In the future, this work can be expanded as follows:
- Build an interactive app to allow others to explore and subset the dataset.
- Scrape more features from the web, like product release date and customer reviews.
- Apply machine learning algorithms to predict the user rating of a product based on the hardware parameters and reviews.